BLOG SPOTLIGHT
Navigating the chaos of data security in the age of GenAI—let’s break down what needs to happen next.
Read more
.png)
Popular
.png)
Sep 19
0
min
Data Security for Generative AI: Where Do We Even Begin?
If you haven’t noticed the wave of Generative AI sweeping across the enterprise hardware and software world, it certainly would have hit you within 5 minutes of attending Big Data London, one of the UK’s leading data, analytics, and AI events. Having attended last year’s show, I can confidently say AI wasn’t nearly as dominant. But now? It’s everywhere, transforming not just this event but countless others. AI has officially taken over!
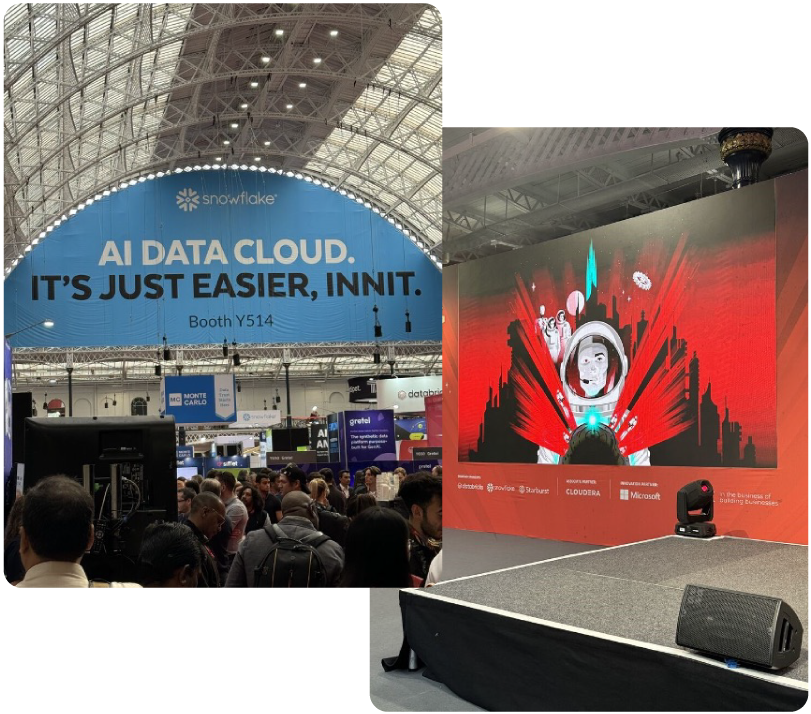
As a data security focused person, it is exciting and terrifying to see all the buzz. I’m excited because it feels like we’re on the verge of a seismic shift in technology—on par with the rise of the web or the cloud—driven by GenAI. And I get to witness it firsthand! But it is terrifying to see all the applications, solution consultants, database vendors and others selling happy GenAI stories to customers. I could scream into the loud buzz of the show floor, “We have seen this movie before! Don’t let the development of GenAI applications outpace the critical need for data security!” I’m thinking about the rush to web, the rush to mobile, the rush to cloud. All of these previous shifts suffer from the same thing: security is boring and we don’t want to do it. What definitely wasn’t boring was using a groundbreaking mobile app from 1800flowers.com to buy flowers—that was cool! Let’s have more of that! Who cares about security, right? That can wait…
Cyber security, and data security in particular, have had the task of keeping up with the excitement of new applications for decades. The ALTR engineering office is in beautiful Melbourne, FL just a few hours away from Disney. When I see a young mother or father with a concerned look racing after their young child who couldn’t care less that they are about to get run over by a popcorn stand, I think “Application users are the kids, security people are the parent, and GenAI is whichever Disney character the kid can’t wait to hug.” It’s cute, but dangerous. This is what is happening with GenAI and security.
As applications have evolved so has data security. Below is an example of these application evolutions and how security has adapted to cover the new weaknesses of each evolution.

What is Making Generative AI Hard to Secure?
The simple answer is: we don’t fully know. It’s not just that we’re still figuring out how to secure GenAI (spoiler: we haven’t cracked that yet); it’s that we don’t even fully understand how these Large Language Models (LLMs) and GenAI systems truly operate. Even the developers behind these models can’t entirely explain their inner workings. How do you secure something you can’t fully comprehend? The reality is—you can’t.
So, what do we know?
We know two things:
1. Each evolution of applications and data products has been secured by building upon the principles of the previous generation. What has been working well needs to be hardened and expanded.
2. LLMs present two new and very hard problems to solve: data ownership and data access.
Let’s dive into the second part first. To get access to the hardware currently required to train and run LLMs we must use cloud or shared resources. Things like ChatGPT or NVIDA’s DGX cloud. Until these models require less hardware or the hardware magically becomes more available, this truth will hold.
Similar to the early days of the internet, sensitive information was desired to be sent and received on shared internet lines. The internet was great for transmitting public or non-sensitive information, but how could banking and healthcare use public internet lines to send and receive sensitive information? Enter TLS. This is the same problem facing LLMs today.
How can a business (or even a person for that matter) use a public and shared LLM/GenAI system without fear of data exposure? Well, it’s a very challenging. And not a problem that a traditional data security provider can solve. Luckily there are really smart people working on this solution like the folks at Protopia.ai.
So, data ownership is being addressed much like how TLS solved the private-information-flowing-on-public-internet-lines. And that’s a huge step forward. What about data access?
This one is a bit tougher. There are some schools of thought about prompt control and data classification within AI responses. But this feels a lot like CASB all over again, which didn’t exactly hit the mark for SaaS security. In my opinion, until these models can pinpoint exactly where their responses are coming from—essentially, identify the data sets they’ve learned from —and also understand who is asking the questions, we’ll continue to face risks. Only then can we prevent situations where an intern asks questions and gets answers that should only be accessible to the CEO.
Going back to what we know, the first item, we will need to build upon the solid data security foundations that got us to this point in the first place. It has become clear to me that for the next few years, Retrieval-Augmented Generation (RAG) will be how enterprises globally interact with LLMs and GenAI. While this is not a silver bullet, it’s the best shot busineses have to leverage the power of public models while keeping private information safe.
With the adoption of RAG techniques, the core data security pillars that have been bearing the load of a data lake or warehouse to date will need to be braced for extra load.
Data classification and discovery needs to be cheap, fast, and accurate. Businesses must continuously ensure that any information unsuitable for RAG workloads hasn’t slipped into the database from which retrieval occurs. This constant vigilance is crucial to maintaining secure and compliant operations. This is the first step.
The next step is to layer access control and data access monitoring such that the business can easily set the rules for which types of data are allowed to be used by the different models and use cases. Just as service accounts for BI tools need access control, so to do service accounts for the purposes of RAG. On top of these access controls, near-real-time data access logging must be present. As the RAG workloads access the data, these logs are used to inform the business if any access has changed and allows the business to easily comply with internal and external audits proving they are only using approved data sets with public LLMs and GenAI models.
Last step, keep the data secure at rest. The use of LLMs and GenAI will only accelerate the migration of sensitive data into the cloud. These data elements that were once protected on-prem will have to be protected in the cloud as well. But there is a catch. The scale requirements of this data protection will be a new challenge for businesses. You will not be able to point your existing on-prem-based encryption or tokenization solution to a cloud database like Snowflake and expect to get the full value of Snowflake.
When prospects or customers ask me, “What is ALTR’s solution for securing LLMs and GenAI” I used to joke with them and say, “Nothing!” But now I’ve learned the right response, “The same thing we’ve always done to secure your data—just with even more precision and focus for today’s challenges.” The use of LLMs and GenAI is exciting and scary at the same time. One way to reduce the anxiety is to start with a solid foundation of understanding what data you have, how that data is allowed to be used, and whether you prove that the data is safe at rest and in motion.
This does not mean you cannot use ChatGPT. It just means you must realize that you were once that careless child running with arms wide open to Mickey, but now you are the concerned parent. Your teams and company will be eager to dive headfirst into GenAI, but it’s crucial that you can articulate why this journey is complex and how you plan to guide them there safely. It begins with mastering the fundamentals and gradually tackling the tough new challenges that come with this powerful technology.
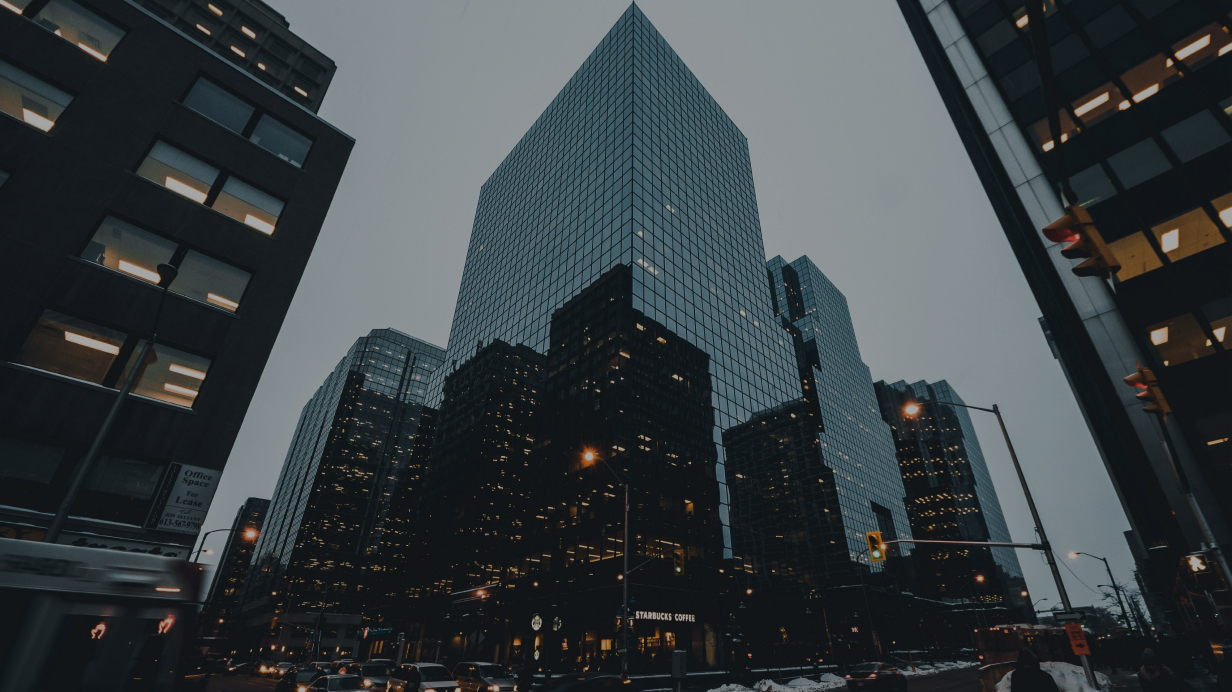
Sep 9
0
min
ALTR Expands GTM Team with Powerhouse Hires to Lead the Charge in Data Security
ALTR isn’t just keeping pace with the evolving data security landscape—we’re setting the speed limit. As businesses scramble to safeguard their data, ALTR is not just another player in the game; we’re the go-to solution for bulletproof data access control and security. And today, we’re doubling down on that promise with three strategic hires to turbocharge our Go-To-Market (GTM) strategy.
Meet the Heavy Hitters
Christy Baldassarre
Christy Baldassarre joins us as our new Director of Marketing, bringing a formidable blend of strategic vision and execution prowess. With a track record of driving brand growth and market penetration, Christy excels at crafting compelling narratives that resonate with target audiences. She’s a master at turning complex concepts into clear, impactful messaging and knows how to leverage the latest digital marketing tactics to amplify ALTR’s voice.
"I am excited to be on such a great team and to be a part of taking ALTR to the next level. I chose ALTR because of its excellence in Cloud Security and Data Protection. This is a great opportunity to collaborate with such a visionary team and contribute to groundbreaking solutions that not only push boundaries but set new standards of how to keep everyone’s data safe." - Christy
Rick McBride
Rick McBride, our new Demand Gen Manager, brings a deep expertise in go-to-market strategy. With a strong foundation in business development, Rick has honed his skills in identifying opportunities and driving pipeline growth from the ground up. He’s not just about crafting campaigns; Rick knows how to connect with decision-makers and convert interest into action.
“A successful go-to-market strategy thrives on seamless collaboration across various teams, and our GTM group is poised to be the driving force behind it. We're set to champion the Snowflake ecosystem—engaging with customers, Snowflake’s Field Sales team, and partners alike—to fuel strategic growth. By leveraging Snowflake's powerful native capabilities in Security and Governance, we aim to deliver at the speed and scale that Snowflake users expect. We're thrilled to extend this value to every organization that prioritizes and trusts Snowflake for their data management needs!” - Rick
George Policastro
Next, we've got George Policastro as our newest Account Executive. George is a seasoned sales professional with a proven track record of closing complex deals and delivering results. His strengths lie in his ability to deeply understand client needs, build lasting relationships, and strategically navigate the sales process to drive success.
"I’m thrilled to join ALTR and tackle one of the biggest challenges organizations face today: securing their sensitive data while unlocking its full potential to drive business growth." - George
ALTR: Defining the Future of Data Access Control and Security
The world of data security and governance has evolved dramatically from the days of simple perimeter defenses. Now, we’re dealing with sophisticated, multi-layered security strategies that need to keep up with cybercriminals who are more aggressive and resourceful than ever. The core principles—knowing where your data is, who can access it, and ensuring its protection—haven’t changed. However, as data moves to the cloud, the challenge is achieving these goals at an unprecedented scale and speed.
That’s where ALTR excels. We’re not just providing solutions; we’re reimagining what data access control and security can be in a cloud-first world. By cutting through the complexities and inefficiencies of traditional methods, we deliver a streamlined, scalable approach that makes data security both simple and powerful. Our intuitive automated access controls, policy automation, and real-time data observability empower organizations to protect sensitive data at rest, in transit, and in use—effortlessly and at lightning speed. With ALTR, securing your data isn’t just more accessible; it’s smarter, faster, and designed for today’s dynamic cloud environments.
With our latest GTM team expansion, we’re fortifying our foundation to evolve into a cloud data security market leader who’s not just part of the conversation but is driving it.

Sep 3
0
min
Unleashing the Power of FPE: ALTR Key Sharing Meets Snowflake Data Sharing
In a world where data breaches and privacy threats are the norm, safeguarding sensitive information is no longer optional—it's critical. As regulations tighten and privacy concerns soar, our customers are demanding cutting-edge solutions that don't just secure their data but do so with finesse. Enter Format Preserving Encryption (FPE). When paired with ALTR's capability to seamlessly share encryption keys with trusted third parties via platforms like Snowflake's data sharing, FPE becomes a game-changer.
Understanding Format Preserving Encryption (FPE)
Format Preserving Encryption (FPE) is a type of encryption that ensures the encrypted data retains the same format as the original plaintext. For example, if a credit card number is encrypted using FPE, the resulting ciphertext will still appear as a string of digits of the same length. This characteristic makes FPE particularly useful in scenarios where maintaining data format is crucial, such as legacy systems, databases, or applications requiring data in a specific format.
Key Benefits of FPE
Seamless Integration
FPE maintains the data format, allowing easy integration into existing data pipelines without requiring significant changes. This minimizes the impact on business operations and reduces the costs associated with implementing encryption.
Compliance with Regulations
Many regulatory frameworks, such as the GDPR, PCI-DSS, and HIPAA, mandate the protection of sensitive data. FPE helps organizations comply with these regulations by ensuring that data is encrypted to preserve its usability and format, which can sometimes be a requirement in these standards.
Enhanced Data Utility
Unlike traditional encryption methods, FPE allows encrypted data to be used in its existing form for specific operations, such as searches, sorting, and indexing. This ensures organizations can continue to derive value from their data without compromising security.
The Role of Snowflake in Data Sharing
Snowflake is a cloud-based data warehousing platform that allows organizations to store, process, and analyze large volumes of data. One of its differentiating features is data sharing, which enables companies to share live, governed data with other Snowflake accounts in a secure and controlled manner while also shifting the cost of the computing operations of the data over to the share's consumer.
Key Features of Snowflake Data Sharing
Real-Time Data Access
Snowflake's data sharing allows recipients to access shared data in real-time, ensuring they always have the most up-to-date information. This is particularly valuable in scenarios where timely access to data is critical, such as in financial services or healthcare.
Secure Data Exchange
Snowflake's platform is designed with security at its core. Data sharing is governed by robust access controls, ensuring only authorized parties can view or interact with the shared data. This is crucial for maintaining the confidentiality and integrity of sensitive information.
Scalability and Flexibility
Snowflake's architecture allows for easy scalability, enabling organizations to share large volumes of data with multiple parties without compromising performance. Additionally, the platform supports a wide range of data formats and types, making it suitable for diverse use cases.
The Power of Combining FPE with Snowflake’s Key Sharing
When FPE is combined with the ability to share encryption keys via Snowflake's data sharing, it unlocks a new level of security and flexibility for organizations. This combination addresses several critical challenges in data protection and sharing:
Controlled Access to Encrypted Data
By leveraging FPE, organizations can encrypt sensitive data while preserving its format. However, there are scenarios where this encrypted data needs to be shared with trusted third parties, such as partners, auditors, or service providers. Through Snowflake's data sharing and ALTR's FPE Key Sharing, companies can securely share encrypted data along with the corresponding encryption keys. This allows the third party to decrypt the data within the policies that they have defined and use it as needed.
Data Security Across Multiple Environments
In a multi-cloud or hybrid environment, data often needs to be moved between different systems or shared with external entities. Traditional encryption methods can be cumbersome in such scenarios, as they require extensive reconfiguration or critical management efforts. However, with FPE and Snowflake's key sharing, organizations can seamlessly share encrypted data across different environments without compromising security. The encryption keys can be securely shared via Snowflake, ensuring only authorized parties can decrypt and access the data.
Regulatory Compliance and Auditing
Many regulations require organizations to demonstrate that they have implemented appropriate security measures to protect sensitive data. By using FPE, companies can encrypt data that complies with these regulations. At the same time, the ability to share encryption keys through Snowflake ensures that data can be securely shared with auditors or regulators. Additionally, Snowflake's robust logging and auditing capabilities provide a detailed record of who accessed the data and when which is essential for compliance reporting.
Enhanced Collaboration with Partners
In finance, healthcare, and retail industries, collaboration with external partners is often essential. However, sharing sensitive data with these partners presents significant security risks. By combining FPE with ALTR's key sharing, organizations can securely share encrypted data with partners, ensuring that sensitive information is transmitted throughout the data's lifecycle, including across shares. This enables more effective collaboration without compromising data security.
Efficient and Secure Data Processing
Specific data processing tasks, such as data analytics or AI model training, require access to large volumes of data. In scenarios where this data is sensitive, encryption is necessary. However, traditional encryption methods can hinder the efficiency of these tasks due to the need for decryption before processing. With FPE, the data can remain encrypted during processing, while ALTR's key sharing allows the consumer to decrypt data only when absolutely necessary. This ensures that data processing is both secure and efficient.
Use Cases of FPE with ALTR Key Sharing
To better understand the value of combining FPE with ALTR's key sharing, let's explore a few use cases:
Financial Services
In the financial sector, organizations handle a vast amount of sensitive data, including customer information, transaction details, and credit card numbers. FPE can encrypt this data while preserving its format, ensuring it can still be used in legacy systems and applications. Through Snowflake's data sharing, financial institutions can securely share encrypted transaction data with external auditors, partners, or regulators, along with the necessary encryption keys. This ensures compliance with regulations while maintaining the security of sensitive information.
Healthcare
Healthcare organizations often need to share patient data with external entities, such as insurance companies or research institutions. FPE can encrypt patient records, ensuring they remain secure while preserving the format required for healthcare applications. Snowflake's data sharing allows healthcare providers to securely share this encrypted data with third parties. At the same time, ALTR enables the sharing of the corresponding encryption keys, enabling them to access and use the data while ensuring compliance with HIPAA and other regulations.
Retail
Retailers often need to share customer data with marketing partners, payment processors, or logistics providers. FPE can be used to encrypt customer information, such as names, addresses, and payment details while maintaining the format required for retail systems. Snowflake's data sharing enables retailers to securely share this encrypted data with their partners; with ALTR, the encryption keys are also shared, ensuring that customer information is always protected.
The Broader Implications for Businesses
The combination of Format Preserving Encryption and ALTR's key-sharing capabilities represents a significant advancement in the field of data security. This approach addresses several critical challenges in data protection and sharing by enabling organizations to securely share encrypted data with trusted third parties.
Strengthening Trust and Collaboration
In an increasingly interconnected world, businesses must collaborate with external partners and share data to remain competitive. However, this collaboration often comes with significant security risks. By leveraging FPE and ALTR's key sharing, organizations can strengthen trust with their partners by ensuring that sensitive data is always protected, even when shared. This leads to more effective and secure collaboration, ultimately driving business success.
Reducing the Risk of Data Breaches
Data breaches, including financial losses, reputational damage, and regulatory penalties, can devastate businesses. Organizations can significantly reduce the risk of data breaches by encrypting sensitive data with FPE and securely sharing it via Snowflake. Even if the data is intercepted, it remains protected, as only authorized parties with the corresponding encryption keys can decrypt it.
Enabling Innovation While Ensuring Security
As organizations continue to innovate and leverage new technologies, such as artificial intelligence and machine learning, the need for secure data sharing will only grow. The combination of FPE and ALTR's key sharing enables businesses to securely share and process data innovatively without compromising security. This ensures that organizations can continue to innovate while protecting their most valuable asset – their data.
Wrapping Up
Integrating Format Preserving Encryption with ALTR's key sharing capabilities offers a powerful solution for organizations seeking to protect sensitive data while enabling secure collaboration and innovation. By preserving the format of encrypted data and allowing for secure key sharing, this approach addresses critical challenges in data protection, regulatory compliance, and data sharing across multiple environments. As businesses navigate the complexities of the digital age, the value of this combined solution will only become more apparent, making it a vital component of any robust data security strategy.
ALTR's Format-preserving Encryption is now available on Snowflake Marketplace.
.png)
Aug 21
0
min
Data Protection at Snowflake Scale
“Today is the day!” you exclaim to yourself as you settle into your desk on Monday morning. After months of meticulous planning, the migration from Teradata to Snowflake begins now. You have been through all the back-and-forth with leadership on why this migration is needed: Teradata is expensive, Teradata is not agile, Snowflake creates a single source of data truth, and Snowflake is instantly on and scales when you need it. It’s perfect for you and your business.
As you follow your meticulously planned checklist for the migration, you're utilizing cutting-edge tools like DBT, Okta, and Sigma. These tools are not just cool, they're the future. You're moving your database structure, loading the initial non-sensitive data, repointing your ETL pipelines, and witnessing the power of modern technology in action. Everything is working like a charm.
A few weeks or months of testing go by, your downstream consumers of data are still using Teradata but are starting to give thumbs up on the Snowflake workloads that you have already migrated. Things are going well. You have not thought about CPU or disk space for the Teradata box in a while, which was the point of the migration. You finally get word from all stakeholders that this trial migration was a success! You call your Snowflake team, and tell them to back up the truck, you are clear to move the remaining workloads. Life is good. But then, comes a knock at the door.
It’s Pat from Security & Risk. You know Pat well and enjoy Pat’s company, but you also do as much as possible to avoid Pat because you are in data and, well, we all know the feeling. Pat tells you, “Heard we are finally getting off Teradata; that’s awesome! Do you have a plan for the PII and SSNs that are kept in that one Teradata database that we require using Protegrity for audit and compliance reasons?” You nod, “I do, but I couldn't do it without your expertise. I’ve been reading the Snowflake documentation, and I'm in the process of writing a few small AWS Lambdas to interface with Protegrity. Your input is crucial to this process.” Pat smiles, gives a non-assuring hand on your back and walks out. Phew, no more Pat.
Four weeks later, you're utterly exhausted. You've logged over 50 hours in Snowflake with fellow data engineers, and tapped into the expertise of one of the cloud ops team members who knows Lambda inside out. You have escalated to Snowflake support, but your external function calls from Snowflake to AWS keep timing out. AWS support is unable to help. Now, you have memory limits being hit with AWS Lambda. Suddenly, the internal network team does not want to keep the ports open to hit Protegrity from AWS, and you need to use a Private Link connection with additional security controls. You are behind on the Teradata migrations. There is no end in sight of the scale problems. Shoot, this is not working.
Don’t worry, you are not alone. This is the same experience felt by hundreds of Snowflake customers, and it stems from the same problem: everything about your Snowflake migration was planned for the new architecture of Snowflake except for one thing: data protection. You followed all the blogs and user guides, and your stateless data pipeline feeding Snowflake with a Kafka bus is perfect. Sigma is running without limits. The team is happy, but they want that customer data now. Except, you can’t use it until you solve this security problem.
Snowflake and OLAP workloads, generally, turned data protection on its head. OLTP workloads are easy to secure. You know the access points and the typical pattern of user behavior, so you can easily plan for scale and up-time. OLAP is widely unpredictable. Large queries, small queries, ten rows, 10M rows, it’s a nightmare for security. There is only one path forward: you must get purpose-built data protection for Snowflake.
You need a data protection solution that matches Snowflake’s architecture, just like when you matched Protegrity to Teradata. If Snowflake is going to be elastic, your data protection needs to be elastic. If Snowflake is going to be accessed by many downstream consumers, you need to be able to integrate data protection into the access policies in Snowflake. Who is going to do that work? Who will maintain this code? How can you control costs? The answer to all those questions is ALTR.
ALTR’s purpose-built native app for data protection is an easy solution for Snowflake. You can install it on your own. You can use your Snowflake committed dollars to pay for the service. ALTR’s data protection scale is controlled by Snowflake and nothing else. It’s the easiest way to get back on track. Call your Snowflake team, ask them about ALTR. It will feel good walking back into Pat’s office with your head held high and your data migration back on track.
Whether your team currently has Protegrity or Voltage, you will face the same problems. Do not waste your time trying to get these solutions to scale, just call ATLR.
Don’t just take my word for it…

Browse All
Thank you! Your submission has been received!
Oops! Something went wrong while submitting the form.

Feb 8
0
min
Data Modernization: Challenges, Best Practices & the Importance of Data Governance
ALTR Blog
Organizations don’t have the time or resources to waste on technologies that don’t work or are difficult to assemble. Creating a future-proof data stack allows organizations to avoid adoption fatigue, build a data-centric culture, keep data secure and make the most of technology investments. We interviewed Pat Dionne, CEO of Passerelle, to find out the prerequisites for a successful data modernization strategy and why data governance plays a critical role in your data ecosystem.
What are the biggest challenges customers face when building a modern data architecture?
It isn’t hard to build a modern data stack – there are a dizzying variety of tools, and each comes with a compelling value proposition. The biggest frustration customers have after they have made the investment and have started trying to get an ROI from their tools. While ingestion can be simple, assembling data into reusable and manageable assets is much more complex. Data modeling and data quality directly impact an organization’s ability to maximize value and agility and are critical for finding a return on the technology investment. Unfortunately, the latter is often forgotten in the decision process.
What components are vital to successful data modernization projects?
When it comes to data modernization, it is critical to have a collaborative approach to cataloging and securing data across an organization. Collaboration builds consensus on data classification terms and rules, creating a universal definition of data asset ownership and a clear understanding of what is required to access data. The more complicated the access scenarios, the more critical it is to have a transparent, cohesive implementation strategy. Similarly, it is essential to invest in tools that support collaboration. For example, we like the simplicity and elegance of ALTR’s solution enabling data governance and security teams.
What role do data governance and data security play in modern data architecture?
Data governance moves data security from a controlling function to an enabling function, while data security protects data from unauthorized access and use. Data governance cannot exist without robust data security; in turn, data security should not inhibit business agility and creativity. Managing the interplay between data governance and security requires understanding how data is used and by whom and requires the proper tooling to enable businesses while providing the appropriate level of control and observability. ALTR simplifies the process by offering clear access controls and immediate visibility into data security protocols.
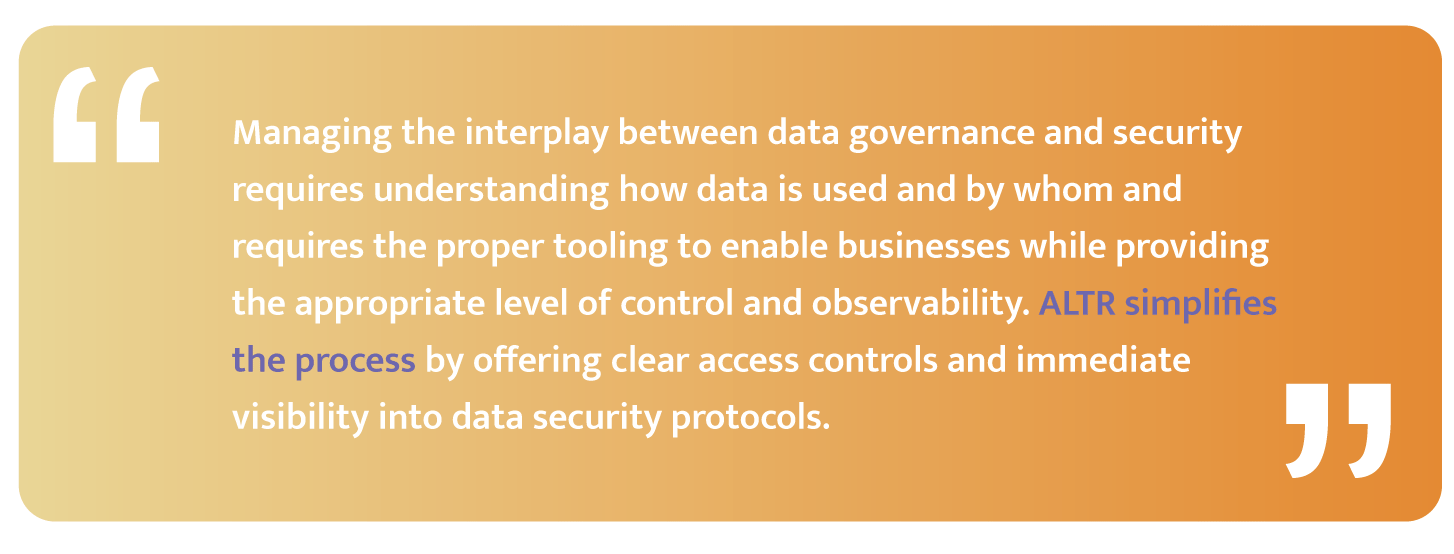
How do you foster a culture of data governance?
For data governance programs to succeed, IT and business stakeholders need to see the value in implementation and adoption. Tying data governance programs to business use is the ultimate unifier - it requires bringing together data stewards, business-line decision-makers and data engineers to a collective understanding of their roles and responsibilities. We refer to this as “Data as a Team Sport.” We are firm believers in use-case-based development – it is easier to get people on board when you have proven results and vocal champions.
What advice would you give to a company starting its data modernization journey?
Introducing practical data governance at the onset of data modernization is easier. Most of the time, organizations will introduce tools and proficiencies throughout a data modernization initiative - the proper data governance practices and tools will apply to every step of that modernization journey and scale with use. In building terms, it is easier to provide structural support with a sturdy foundation than to rely on scaffolding once the walls start to go up.
How do you predict the data management landscape will change in the next 3-5 years?
I see three major trends in the next three to five years:
- First, we will see an increase in automation and intelligence in data management tooling, fueled by AI developments and human brilliance.
- Organizations will demand more integrated solutions to reduce technical debt and manage leaner technology stacks.
- Not only will we see increased regulatory compliance requirements, but we will also enter an era of enforcement, where the government will become more aggressive at enforcing data privacy laws.
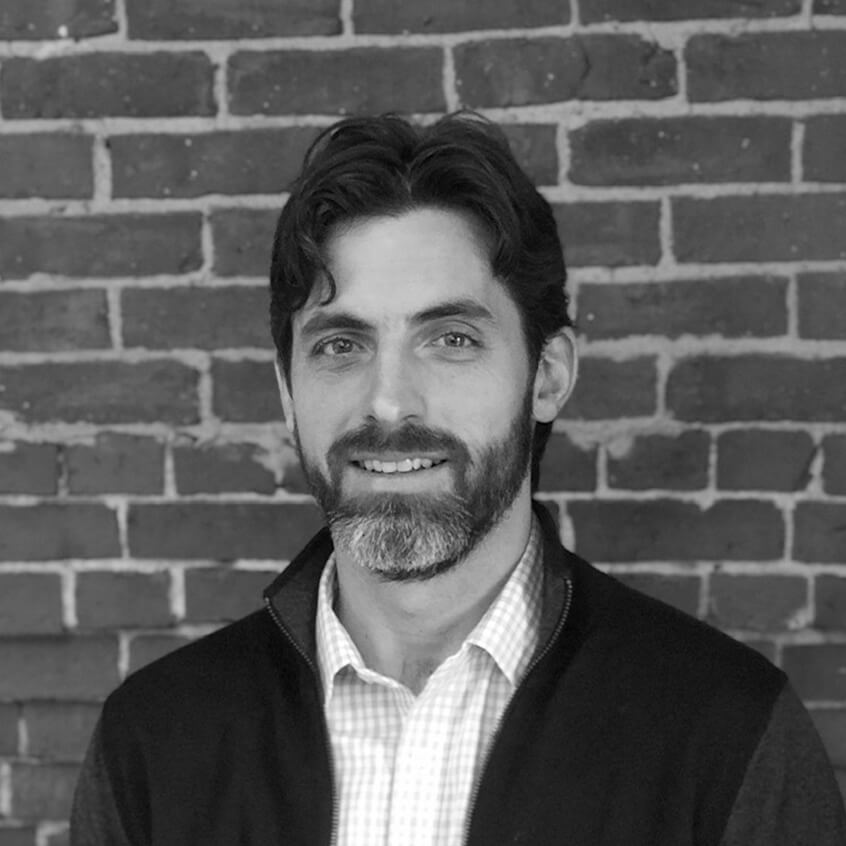
Pat Dionne, CEO of Passerelle
Passerelle offers solutions for business growth and results, and with that, a team of experienced technical contributors and managers and the innovative technologies to create the right solution for clients. Pat is at the heart of this synergy, bringing a deep understanding of the modern technologies capable of addressing today’s complex data business challenges, as well as the proven capacity to build and empower highly effective teams.

Feb 1
0
min
5 Tips for Data Governance in 2023
ALTR Blog
Many data governance solutions claim to solve every data privacy and protection issue, but we know that no two data governance solutions are created equal. As we launch into the New Year, we’ve listed our top 5 tips for Data Governance in 2023. These tips will help you determine what you need from your data governance solution, identify a few red flags to look out for, and point out some key differentiators that may help make your decision for you.
Tip 1: Keep tabs on your organization’s sensitive data.
The first step to ensuring your data governance solution is the right fit for you, is asking the question: “Where does sensitive data exist within my organization, and is it protected?” Understanding what sensitive data you store and who has access to it are critical first steps to ensuring the data governance solution you implement will fit your needs. While only certain data requires protection by law, leaked data can cause a headache across your organization – from damaging your reputation to the loss of loyal customers. It is essential that your data be discovered and classified across your organization’s ecosystem at all times.
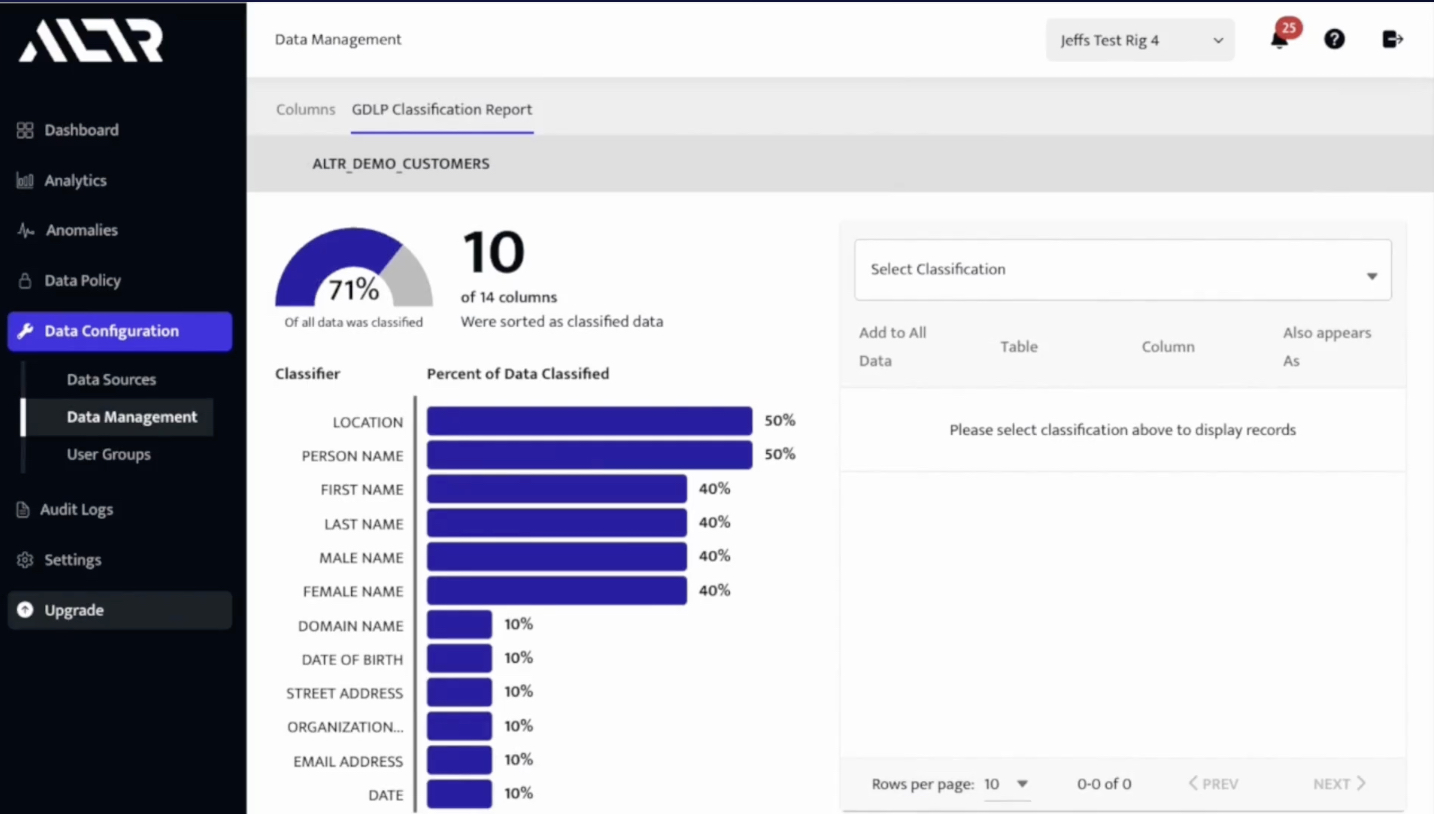
Tip 2: Does your Data Governance solution offer complete coverage?
Data classifiers and catalogs are valuable and are extremely necessary in context, but at the end of the day, they cannot offer you a full governance solution. For complete data governance, you must not only be able to find and classify your data, but see data consumption, utilize thresholds to detect anomalies and alert on them, respond to threats with real-time blocking, and tokenize critical data at rest. True data governance will need to address a wide spectrum of access and security issues, including Access Controls, Compliance, Automation, Scale, and Protection. ALTR simplifies these steps for you – allowing you the ease of point and click solutions to better secure and simplify your data.
Tip 3: More expensive doesn’t mean better.
Many data governance solutions cost anywhere from $100k to$250k per year just to get started! These large, legacy platforms require you to invest valuable time, resources and money to even get started. You may need an army of costly consultants and six months to implement. On the other hand, ALTR’s pricing starts at free for life. Our Free Plan isn’t a trial plan, it’s just that – Free. Our Free plan gives you the power to understand how your data is used, add controls around access, and limit your data exposure. You can see how ALTR will work in your data ecosystem without risk.
If you need more advanced governance controls, integration with your enterprise governance and security platforms, or increased data protection and dedicated support, our Enterprise and Enterprise Plus plans area vailable. ALTR’s tiered pricing means there’s no large up-front commitment—you can start for free and expand if or when your needs change. Or stay on our free plan forever.
Tip 4: The Who of Data Governance
Clearly defining roles within your organization surrounding who needs access to data and when will set you up for success when it comes to protecting sensitive data within your organization.
When you know why each person needs the data you are protecting, you can build access control policies to fit highly specific purposes. Using ALTR you can create policies that limit access based on which data is being requested, who is requesting it, the access rate, time of day, day of week, and IP address. ALTR’s cloud-based policy engine and management console allow you to control data consumption across multiple cloud and on-premises applications from one central location.
Tip 5: Does your data governance solution allow you to scale?
Scalability may be the one thing that makes or breaks your data governance solution in 2023. As regulations and laws surrounding data privacy become more common, the more the data you own will need to be protected. The more data you need protected, the more time your data team is needing to allocate to processes that could easily be automated within ALTR. Governance solutions should easily implement and manage access for thousands of users to match. Scaling policy thresholds as needed allows you to optimize collaboration while stopping data theft or accidental exposure.
Bonus Tip: Start for Free
We anticipate that 2023 will be a critical year for companies being held accountable for the sensitive data they own. ALTR makes getting ahead of the curve simple, easy, and achievable. With ALTR’s free data governance and security integration for Snowflake, you can automatically discover, classify, and tag sensitive data with a checkbox. Add controls like data masking from a drop-down menu. Get going in less than an hour. No SnowSQL is required.
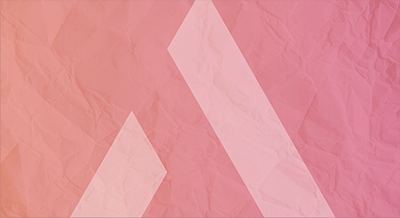
Jan 25
0
min
PII Security - Your Complete Guide to Protecting Personally Identifiable Data
ALTR Blog
What is PII Security?
PII security has become something just about everyone has had to think about in the last few years with the increase in personal data breaches and the passage of the GDPR regulations in Europe. But that doesn’t mean it’s well understood. What do we mean when we talk about PII data anyway? Personally Identifiable Information or PII data generally refers to information that is related to or key to identifying a person. There are broader terms such as “personal data” or “personal information,” but “PII” has become the standard acronym used to refer to private or sensitive information that can identify a specific individual. The US NIST framework defines Personally Identifiable Information as any representation of information that permits the identity of an individual to whom the information applies to be reasonably inferred by either direct or indirect means.
While the abbreviation “PII” is commonly used in the United States, the phrase it abbreviates is not always the same – there are common variants based on personal or personally, and identifiable or identifying. The meaning of the phrase "PII data" ends up varying depending on the jurisdiction and the purpose for which the term is being used. For example, where the General Data Protection Regulation (GDPR) is the primary law regulating PII data, the term "personal data" is significantly broader. Regardless of the definition used, the focus on PII security is also growing quickly.
PII security consists of ensuring that only approved users have access to this most sensitive of personal data. In some cases this is required by regulation, but in the US, without a federal regulation like GDPR, it's more often a requirement to maintain customer trust. In this blog, we'll outline PII data examples, the differences between PII, PHI and PCI and explain the steps you should take to identify PII and ensure it's secured.
PII Data Examples
The first step to PII security is understanding what is considered PII data. As mentioned above, it’s more complicated than it may first appear. Not all private information is PII and not all PII data is private information. In fact, much of the information considered PII data and covered by regulation is actually publicly available information, such as an individual’s name or phone number. However, some of the information, especially when combined and in the hands of bad actors, can lead to negative consequences for individuals. Here are some PII examples:
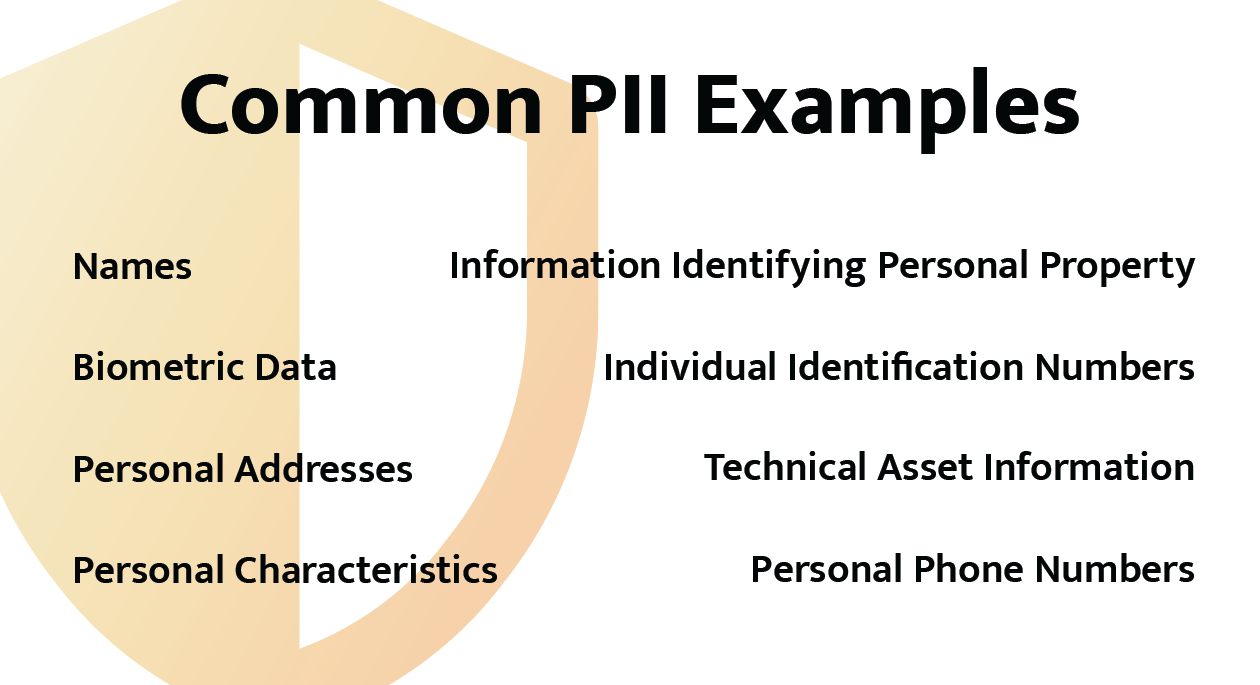
- Names: full name, maiden name, mother’s maiden name, or alias
- Individual identification numbers: social security number (SSN), patient identification number passport number, driver’s license number, taxpayer identification number, financial account number, or credit card number
- Personal address: street address, or email address
- Personal phone numbers
- Personal characteristics: photographic images (particularly of a face or other identifying physical characteristics), fingerprints, handwriting
- Biometric data: retina scans, voice signatures, facial geometry
- Information identifying personal property: VIN or title number
- Technical Asset information: Internet Protocol (IP) or Media Access Control (MAC) addresses that consistently link to a particular person’s technology
What Data Does Not Require PII Security?
PII security becomes easier if you understand what is not PII data. The examples below are not considered PII data alone as each could apply to multiple people. However, when combined with one of the above examples, the following could be used to identify a specific person:
- Date of birth
- Place of birth
- Business telephone number
- Business mailing or email address
- Race
- Religion
- Geographical indicators
- Employment information
- Medical information
- Education information
- Financial information
PII vs PHI vs PCI Data
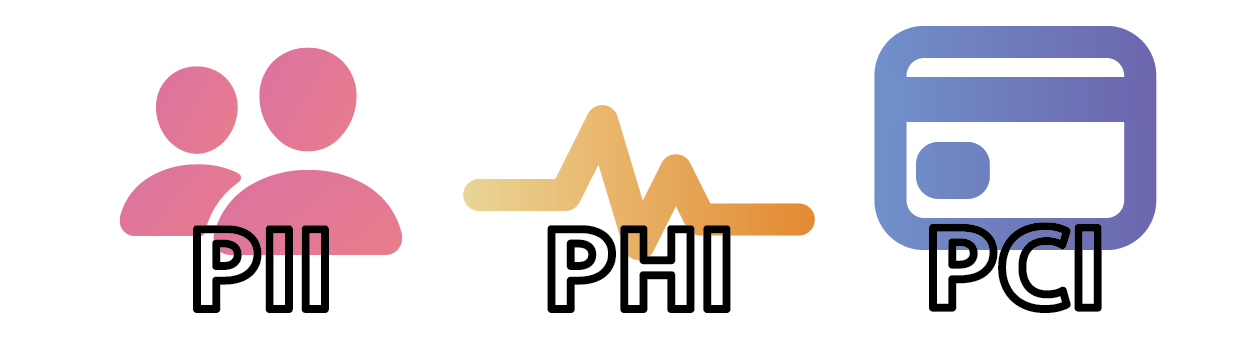
PII data has much in common and some overlap with other forms of sensitive or regulated data such as PHI and PCI, but it is not the same. Confusion often arises around whether PII means information that is identifiable (can be associated with a person) or identifying (associated uniquely with a person, so that the PII actually identifies them). In narrow data privacy rules, such as the Health Insurance Portability and Accountability Act (HIPAA), PII items have been specifically defined. In broader data protection regulations such as the GDPR, personal data is defined in a non-prescriptive principles-based way. Information that might not count as PII under HIPAA could be considered personal data per GDPR.
PHI data is personal health information as defined by the Health Insurance Portability and Accountability Act of 1996. HIPAA provides federal protections for personal health information held by covered entities and gives patients an array of rights with respect to that information. At the same time, HIPAA permits the disclosure of personal health information needed for patient care and other important purposes. This federal law required the creation of national standards to protect sensitive patient health information from being disclosed without the patient’s consent or knowledge. The US Department of Health and Human Services (HHS) issued the HIPAA Privacy Rule to effect the requirements of HIPAA. The HIPAA Security Rule protects a subgroup of information covered by the Privacy Rule. In addition to very clear health information, there is some overlap as when PII data like name, date of birth, and address are tied to personal health information, it is considered PHI as well.
PCI data stands for “payment card industry” and is defined by a consortium of financial institutions comprising the Payment Card Industry. The definition comes from the rules for protecting data in the PCI-DSS or payment card industry data security standard. The PCI Security Standards Council (SSC) defines “cardholder data” as the full Primary Account Number (PAN) or the full PAN along with any of the following identifiers: cardholder name, expiration date or service code. The rules were implemented to create an additional level of protection for card issuers by ensuring that merchants meet minimum levels of security when they store, process, and transmit cardholder data.
In the past PCI data might have been considered the most valuable and most at risk because it was related to financial data and could be used to directly access money. However, as many of us have unfortunately learned due to rampant credit card fraud over the last few years, credit card numbers can be easily changed. It’s not nearly as easy to move, change your social security number, or even your name. Those who have dealt with identity theft can understand how devastating it can be when unknown loans or other fraud show up on your credit report. And health information is simply unchangeable as its part of a person’s permanent “life record.” That puts PII data and PHI data in the lead in the race for data value and data risk. PII data might be considered more at risk due to its proliferation so PII security should always be a priority.
PII Security and the Internet

Before 1994, very little of our PII data was easily accessible so PII security wasn't as critical. If you wanted someone’s phone number, you had to know their name and have a hefty copy of what we called the “white pages” (a phone book) in order to look them up. Maybe a bank or telephone company had access to thousands of phone numbers, but not the average person. All of that changed with the advent of the Internet. The concept of PII data has become prevalent as information technology and the Internet have made it easier to collect PII. Every online order requires a name and email, not to mention physical address or phone number. This has led to a profitable market in collecting and reselling PII. PII can also be exploited by criminals in stalking or identity theft, or to aid in the planning of criminal acts. In reaction to these threats, many website privacy policies now specifically inform users on the gathering of PII, and lawmakers have enacted a series of regulations to limit the distribution and accessibility of PII making PII security a priority for consumers and companies.
PII Security Regulations
The era of stringent PII data privacy regulations that required PII security really kicked off with the implementation of the European Union’s General Data Protection Regulation (GDPR) in May 2018. This regulation requires organizations to safeguard personal data and uphold the privacy rights of anyone in EU territory. The regulation includes seven principles of data protection that are required and eight privacy rights that must be enabled. It also gives member state-level data protection authorities the power to enforce GDPR with sanctions and fines. The GDPR replaced a country-by-country patchwork of data protection laws and unified the EU under a single data protection regime. The regulation doesn’t apply to just European companies, however. Any company holding personal data of European citizens must comply.
The US is further behind the PII privacy regulation game. There is as yet no federal or national privacy regulation that applies across the country. The US is still in the patchwork era with some states like California, Utah, Colorado, Connecticut and Virginia passing state-level regulations. Five more states have introduced regulations. In 2022, a new bipartisan regulation called the American Data Privacy and Protection Act was introduced in the US House of Representatives. It follows the direction of GDPR and would apply to data controllers and accessors. It is effectively a consumer “Bill of Rights” around PII data privacy. The legislation currently sits in the House of Representatives for approval.
4 Steps to Complete PII Security
These privacy regulations have specific rules around PII security – what data should be protected and how. But in order to comply fully and reduce risk of censure, fees or fines, companies will need to take 4 key steps:
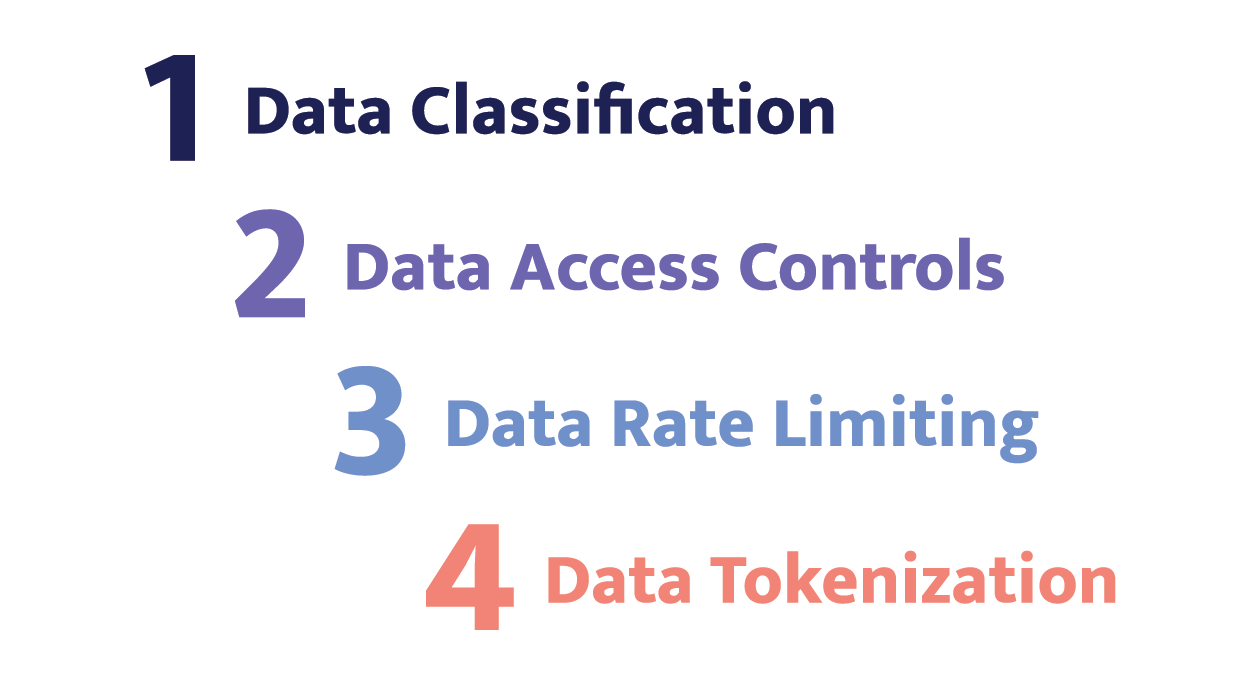
- Data classification: The first step to PII security is to identify sensitive information stored in your company’s databases. This can be done manually by reviewing all the databases and tagging columns or rows that contain PII. Some database solutions allow you to write SQL processes to do this also. However, it’s much faster and less error-prone to utilize an automated solution to find and tag social security numbers, date of birth or other key information wherever it’s located.
- Data access controls: Once PII data is identified controls that allow only approved individuals to access sensitive data should be applied. These controls can include data masking (changing characters to ***) and row or column-level access policies. A common additional requirement is auditable documentation of who has accessed what data and when.
- Data rate limiting: Because it’s best to assume any credentials could be compromised at any time, it’s best to limit the amount of damage even authorized access can do. Instead of allowing millions of lines of data to be downloaded, apply controls that limit the amount of data by role, by location, by time access to reduce the risk of a massive breach.
- Data tokenization: Finally, the most sensitive data should be secured via a data tokenization solution that ensures even if “data” is accessed by a bad actor, they will only get their hands on tokens that are useless to them. The real data is stored in an encrypted token vault.
Conclusion
The problem of PII security is only on the upswing. As companies extract more insight and value from personal data on consumers, product users and customers, they’ll continue to gather, hold, share and utilize data. In fact, companies are not just collecting data for their own use, but also to monetize it by selling the insights on their own customers to others to glean information from. While data collection and storage are increasing, laws regulating how this data can be stored and used are also increasing. Companies can stay ahead of the curve with processes and solutions to help scale PII security with the growth of PII data.
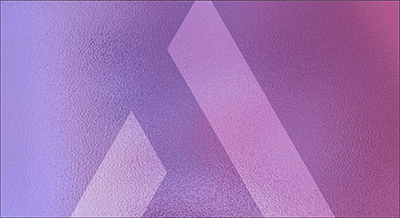
Jan 12
0
min
ALTR vs Other Snowflake Data Governance Solutions
ALTR Blog
As a Snowflake Premier Partner and founding member of the Snowflake Data Governance Accelerated Program, we get a lot of questions about how ALTR is different from other Snowflake data governance solutions, including Snowflake!
The short answer is that we automate the existing native Snowflake governance features for data masking policies and role-based and row-level access policies. Why is that important? Why Is that valuable? When you automate these Snowflake features, it allows Snowflake users to address some key challenges.
Bridging the Snowflake Skills Gap
First, you get to the opportunity to address a skills gap. Maybe some of your team members are not as trained up on SnowSQL yet, or they haven't taken all of the Snowflake certification training, especially if you're early in your Snowflake journey. Maybe you and your team don't have time to learn about data masking policies or some of the nuances that come with Snowflake row level policies, and so ALTR can help you automate that in a very simple and easy to use manner. ALTR’s fast SaaS implementation, access via Snowflake Partner Connect, and no-code policy management take the burden off your team and can even allow other data owners throughout the organization to handle data access controls and enforcement.
Snowflake Data Governance at Scale
The second thing you get to address is deploying these capabilities at scale. We've seen a number of customer projects where implementing these data controls at scale is taking up entire teams of people when it just shouldn’t need that many resources. If you have one centralized tool like ALTR to manage who has access to what data and how much, you take a lot of that kind of scale overhead, and that friction of growing with Snowflake, out of the equation. This comes into play if you set up your Snowflake governance policies the way you want for one database or one account. If you're part of a large organization, you may want to apply that across multiple databases. We recently encountered a company that had nine accounts across all three different cloud providers that Snowflake offers. How do you make that portable across all of those accounts, and all of those deployments? ALTR can make this easy.
See how ALTR’s features compare to other Snowflake Data Governance solutions

A Single Source of Snowflake Data Access Truth
There’s a lot of confusion out there in the market around what “data governance” is exactly. When you’re thinking of other “data governance” solutions for Snowflake like a Collibra or Alation or Immuta or others who are in this space, keep in mind that there are other parts of data governance and many handle some processes like data classification or cataloging, but ALTR’s sole focus is on delivering that single source of truth for data access. You can see how users are using data, control which users have access to which data, reduce risk by limiting the rate of data access, and put powerful tokenization data security over the most sensitive data, all with ALTR.
Low Cost, Fast Implementation, Enterprise Quality
This kind of Snowflake data governance is a really hard problem for companies to solve, even when they had full teams and full budgets to attack it. But moving into 2023, we’re seeing companies lose headcount and resources and getting very picky about selecting the specific tools to help accomplish specific goals. One of the other major differences between ALTR and some of the other Snowflake data governance solutions is that we’re waging a war on six figure price points for solutions and six months of professional services to implement. If you are being offered or sold a tool that is very expensive and is going to take you a long time to roll out and learn to use, those vendors don't have your best interest in mind. With our pure SaaS platform (which other solutions are notw) we’re making data governance easy to buy, easy to implement, and easy to use. Bottom line: ATLR provides the functionality companies need to govern data at a price point other solutions just can’t touch.
See how ALTR’s Snowflake Data Governance solution can work for you. Try our Free Plan today.

Jan 10
0
min
The 2023 Data Governance Landscape: Setting Data Privacy Goals
ALTR Blog
It’s no secret that Data Governance, PII, and Data Security were among the most talked about topics in Q4 of 2022. Security breaches were rampant and technology teams continued to feel stretched thin, while sensitive data was sometimes unwittingly left unprotected and at risk for exposure. We’ve compiled some key guidance from our partners and industry leaders to help you implement strong data governance in 2023 – from simplifying the definition of data governance, to emphasizing the importance of scalability and automation within your data governance plan.
Alation: Key Insights: Forrester’s New Data Governance Solutions Landscape
This blog, written by John Wills, Field CTO at Alation, takes a look at data governance from a wholistic perspective – explaining the big picture of creating a data governance plan for your organization, while recognizing certain aspects that may vary between companies. Wills teaches us that your company’s data governance solution should exist cross-departmentally and shares that people often miss the mark when their data privacy exists in a vacuum.
Tableau: Keep Your Data Private and Secure
Sheng Zhou, Product Manager at Tableau, writes about the importance of data privacy and protection – specifically from the perspective of protecting and securing PHI to meet HIPPA laws. Zhou shares that, regardless of the type of data you’re protecting, it is a critical business component to be vigilant about securing your sensitive data. Zhou mentions that data governance and data privacy are so important, these processes have to be part of normal and everyday business operations.
BigID: How Strong Data Governance With BigID Drives Down Privacy Compliance Costs
Peggy Tsai, Chief Data Officer at BigID, discusses how having strong data governance can help drive down your privacy compliance costs, and, at the end of the day, can save your company a lot of money. Tsai begins this blog by explaining certain laws around data privacy (General Data Protection Regulation (GDPR), California Consumer Privacy Act (CCPA) and what can happen to your company when the GDPR requests a Data Subject Access Request (DSAR). Tsai provides an in-depth analysis outlining the importance of strong data governance, so your company can avoid errors, legal fines, and the headache when you receive a DSAR.
Alation: Becoming a Data Driven Organization in 4 Steps
In this blog post, Steve Neat, GM EMEA at Alation walks through tangible steps you can take to ensure your organization is data driven. Neat explains that becoming a data driven organization isn’t just about adding new technologies to your tech stack, it truly requires full investment from all stakeholders. Neat shares how Data Governance plays a huge role in being a data driven organization, by creating processes surrounding where your data is stored and who can access it. Agility is a key factor in data governance – ensuring your organization is in the driver’s seat of protecting your data.
As uncertainty continues to rise in numerous business sectors across the globe, we’re seeing people recognize the need for strong data governance as well. We’re here to help you ensure your organization is ahead of implementing and streamlining a data governance plan. ALTR’s free plan is the perfect place to start - you can automatically discover, classify, and tag sensitive data with a checkbox. It’s easy to get going in less than an hour with no SnowSQL required.
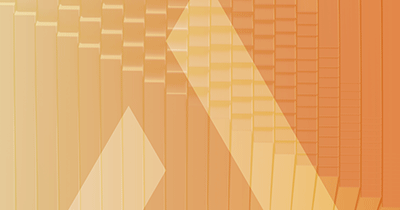
Jan 18
0
min
Snowflake Data Classification: DIY vs ALTR
ALTR Blog
Have you ever walked into a store and noticed that while some items are displayed freely on shelves, some are visible, yet locked behind glass? We can guess that those items are higher quality, higher value, higher risk. It's pretty clear when inventory comes into the store which items fit this category. It can be less clear when data comes into your database. That's where data classification can help.
In this blog post, we’ll explain what data classification is, why data classification is an important step in your data security strategy, and how you would classify data yourself with SQL versus doing it automatically with ALTR.
What is Data Classification?
Data classification is the process of identifying the type of information contained in each column of your database and categorizing the data. This is an important first step to securing your data. Once you know the type of information contained in each column, you will be able to compare the type to a list of information types that your business considers sensitive. This in turn will make it possible to protect that data with appropriate data access policies. Before you create a column-level policy, you should classify it. By implementing data classification, you can minimize the risk of a sensitive data compromise.
Data Classification Factors
To protect your company’s sensitive data, you must first know what type of data you have. Therefore, data classification is a must to avoid having your data hacked by cybercriminals or leaked by individuals inside your business. To determine how to apply data classification consider the following factors:
- Timing: In order to enforce a data policy, you must know which columns contain sensitive data. So, you need to classify your data before implementing data access policies. You should also reclassify any time you add new sources of data.
- Methods: The method you use should involve sampling actual data values found in the data. Avoid relying completely on the name of the column.
- Automation: Classification can be tedious when done manually. A typical database will have hundreds if not thousands of tables, and each table can have hundreds of columns giving rise to missed columns and errors in copy/pasting results.
- What Data is Sensitive: Have a list of the information types that are sensitive in your situation. For example, what data security regulations apply to your company, what does your internal data security team require, and so on.
These factors will help to ensure that your data classification efforts are efficient and thorough.
How Snowflake Data Classification Works DIY
Read on to learn what’s required to classify data in Snowflake yourself with SQL via three different methods: good, better and best.
Who Can Do It: A software developer who can manually write SQL code AND categorize and manage data well
Downsides to manually classifying data in Snowflake:
- Time-consuming
- Higher risk of missing data that needs to be classified
- You’ll have to manually store your results in a database, making it difficult for non-technical users to analyze the results
1) “Good” Method: Column Name
This is a way to identify what type of data is in a column by looking at the column name. You can run a query that uses a conditional expression for each data type against the information schema inside of Snowflake.
The query result will display every column of data that matches your condition in your Snowflake account. The downsides are that you must run the query for every data type you want to identify, and you might miss columns that need to be identified if they weren’t named clearly. For example, if you’re trying to identify all columns of ‘email’ but it’s abbreviated as ‘eml,’ then it won’t be returned in your query.
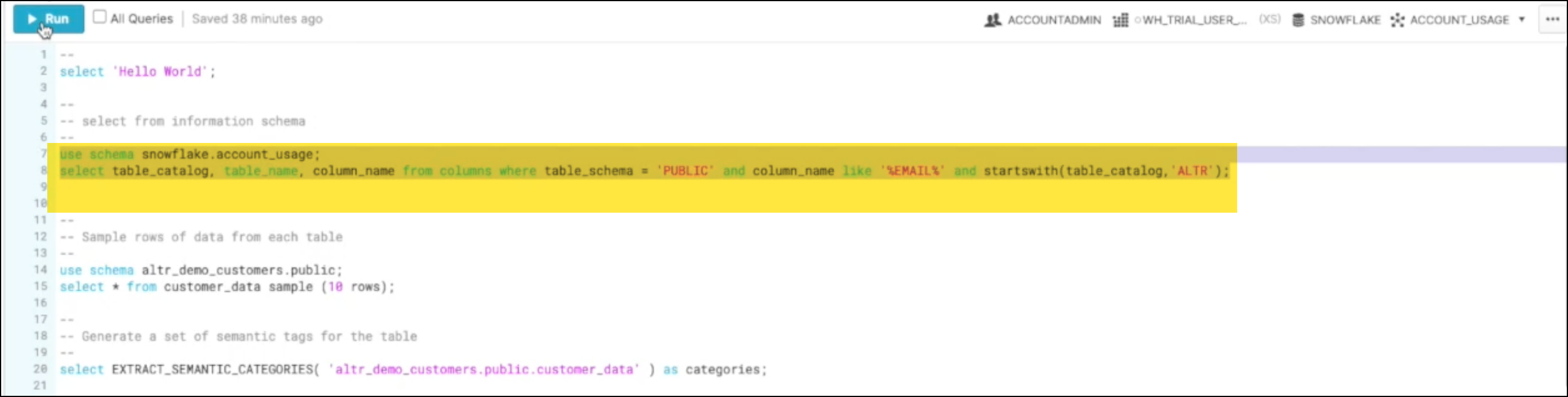

2) “Better” Method: Sample Rows of Data
This is better than the column name method because it will grab a sample of rows and then you can clearly see the content of each column. However, it’s still not the ‘best’ approach. Because the query will display multiple rows and column values for you to view, this can be time-consuming and overwhelming.
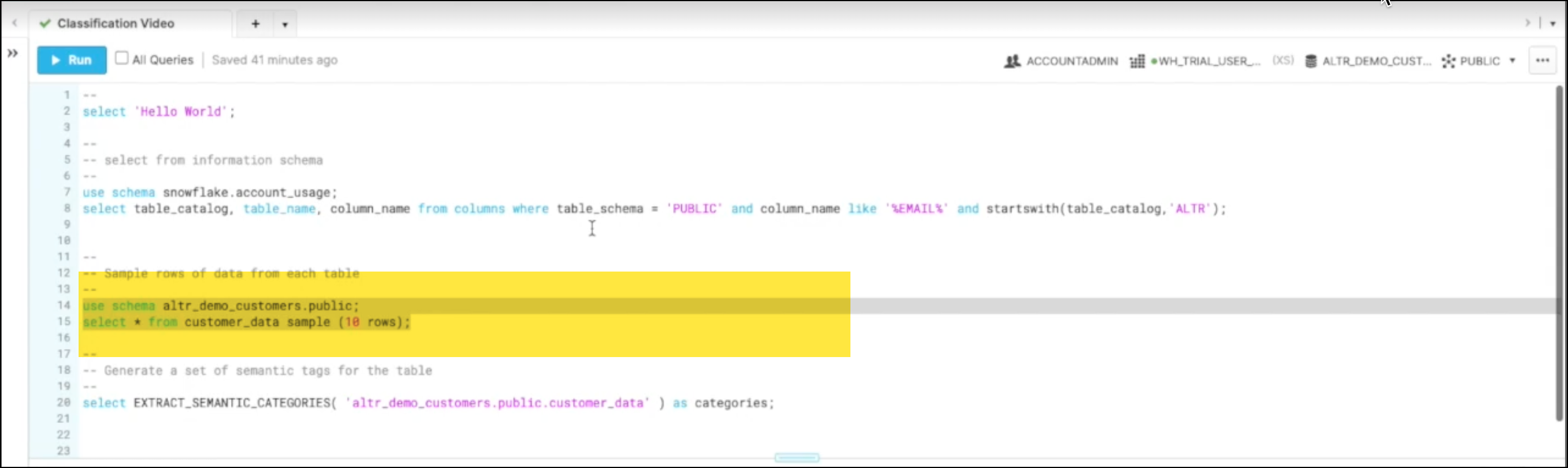

3) “Best” Method: Extract semantic categories
This data categorization method is the best one because it does the sampling for you. You can run extracted categories from a table and a JSON object with scored classification results will be generated in the query result. The caveats are that you must run this across each table in your database, and you must manually store and present results to use them to create access policies


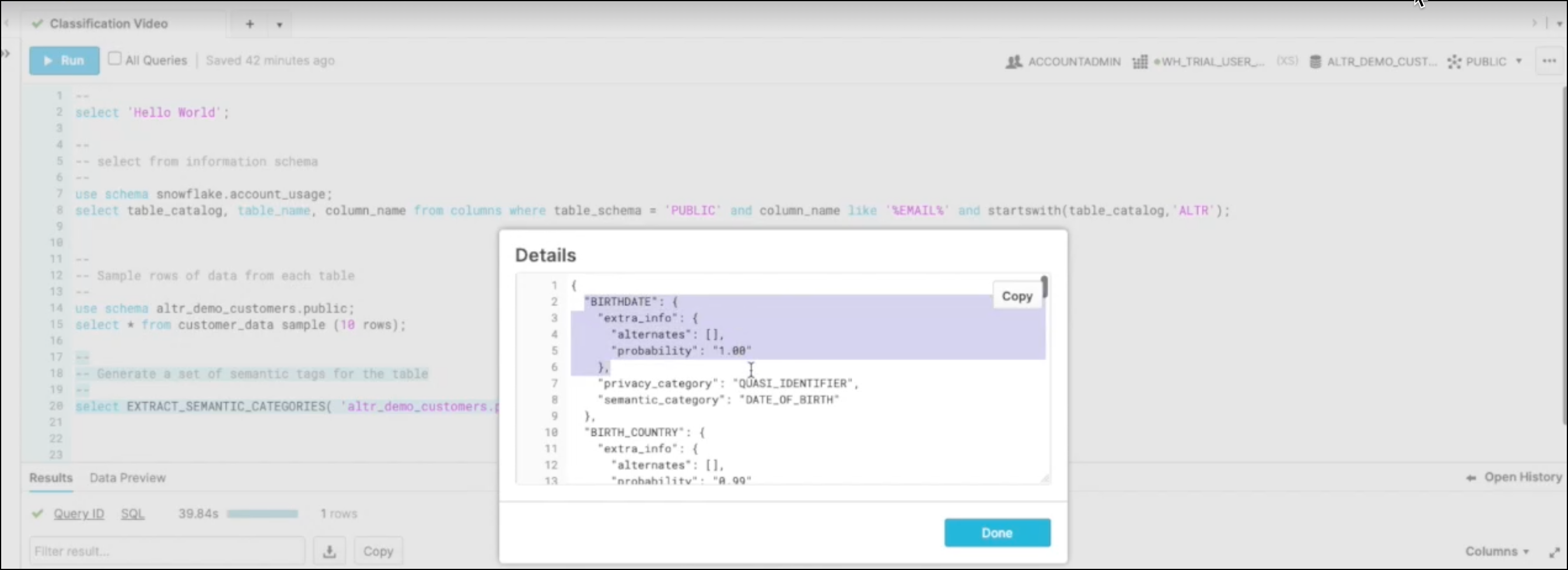
How Snowflake Data Classification Works in ALTR
While you could choose one of the ‘good, better, and best’ approaches above to classify your data manually in Snowflake, using ALTR to automate data classification is the ‘supreme’ approach.
Who can do it: Anyone can do it and you don’t have to write SQL or log in to Snowflake.
Downsides to classifying data in ALTR: None
There are only four steps to ALTR Snowflake data classification.
- Simply choose the database that you’d like to classify (shown in figure 8).
- Check the box beside Tag Data by Classification.
- Choose from the available tagging methods.
- Click on Update. This starts the process of classifying all the tables in that database. When the job is complete, you’ll receive an email to let you know it’s done.
NOTE: An object tag is metadata (such as a keyword or term) that is assigned to a piece of information as a description of it for easier searchability. ALTR can use object tags assigned to columns in Snowflake to classify data or, if those or not available, ALTR can assign tags to columns using Google DLP classification.
The classified data results will be integrated into a Data Classification Report.
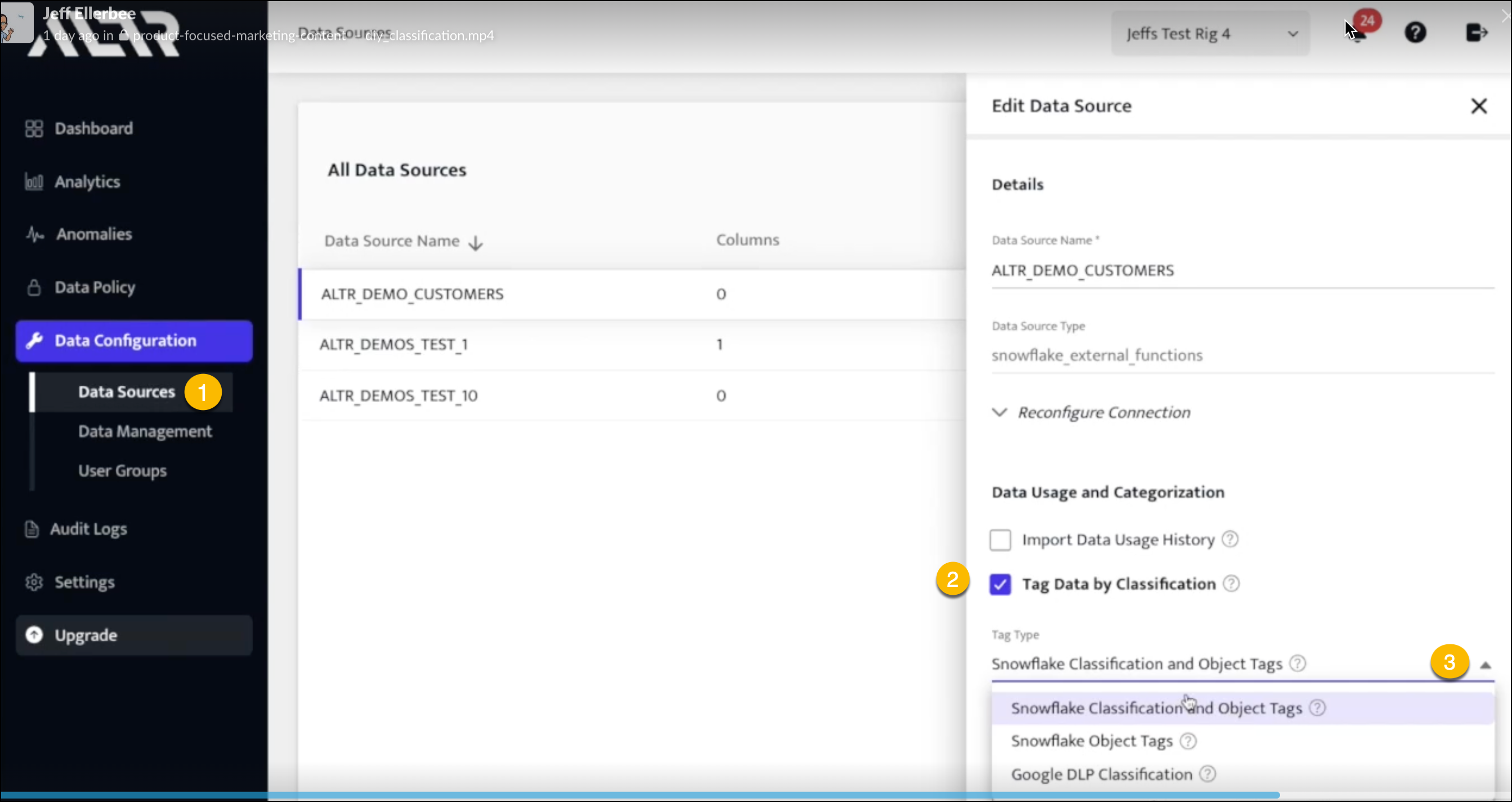
Snowflake Data Classification Use Cases
Here are a couple of use case examples where ALTR’s automated data classification capability can benefit your business as it scales with Snowflake usage.
Use Case 1. Protected health information
Your data team is integrating an employee dataset from a recently acquired hospital into your main data warehouse in Snowflake. You need to determine which database columns have healthcare-related data in them (e.g., social security numbers, diagnostic codes, etc.,). The original developers of the dataset are no longer available, so you use ALTR to classify the dataset to identify those sensitive columns.
Use Case 2. Financial records information from sales
You are a healthcare product manufacturer and you have just signed a new online reseller for your products. The resellers sales data will be dumped into your Snowflake database every week and will contain sales transaction data including addresses, phone numbers, and payment information; however, you don't know where this data is located in the database.
What You Could be Doing: Automating Snowflake Data Classification with ALTR
In today’s world, implementing data classification as part of your company’s security strategy is critical. You can’t afford to put your company at risk of fines and lawsuits due to data breaches that could’ve been prevented. Do you or your security team have hours in a day to spend manually writing SQL code each time that you add data to your databases? Do you want to spend hours trying to figure out why a query didn’t generate any results due to unclear column names or other issues? We’ve made using ALTR such a convenience that you don’t even have to write any SQL code or log into Snowflake! It’s a simple point-and-click four-step procedure in ALTR and you’re done!
Watch the ‘how-to’ comparison video below to see what it looks like to manually classify Snowflake data versus automating it with ALTR.
Ready to give it a try? Start with our Free Plan today

Dec 14
0
min
2022 Data Wrapped: Privacy, Governance and Security News
ALTR Blog
Data protection and data privacy have continued to appear on the front page of local and national news throughout the year and as we close the final chapters of 2022. Remote work and scattered teamwork continue for many, pulling IT, governance, data and security teams in disparate directions, often not allowing the capacity to face data privacy and protection issues. We saw that reflected throughout the year in the many trending topics and news headlines.
Without further ado, we present our 2022 Data Wrapped:
The 'Next Big Thing' in Cyber Security
15 industry experts from Forbes Technology Council, including ALTR CEO James Beecham, discuss cybersecurity awareness and key items every organization’s leadership team should take note of. Read More
US Federal Data Privacy Law Looks More Likely
While the United States doesn't have a federal data privacy law yet, in May of this year, legislators introduced the Data Privacy and Protection Act “which is a major step forward by Congress in its two-decade effort to develop a national data security and digital privacy framework that would establish new protections for all Americans.” In addition, 5 US States have data privacy legislation going into effect in 2023.
75% of the World Will Be Covered by Data Privacy Regulations
Whether your company exists in a state with data legislation or not, now is the time to think about protecting your sensitive cloud-based data. Gartner predicts that “by year-end 2024, 75% of the world’s population will have its personal data covered under modern privacy regulations.” Europe led the charge in formalizing modern privacy laws with the GDPR, a bill that passed in 2018 regulating the handling of sensitive data. And while the United States is still catching up on state-by-state laws, Gartner believes that due to the COVID-19 pandemic and rising cases of data breaches, security and risk management (SRM) will only gain in prevalence as we move into the new year.
Data Breaches Continue to Make Headlines in 2022
Unfortunately, data theft continued to be a prevalent issue in 2022, with Apple, Meta, Twitter, and Uber being among the list of companies who suffered significant data breaches. We're seeing data breaches this year, even more than years past, impact companies across all sectors and sizes.
In September of 2022, Uber's computer network suffered a "total compromise" due to a hacker wrongfully gaining access to their data. Email, cloud storage and code repository data were all breached. The hacker, an 18-year-old, told The New York Times that Uber was an easy target for him "because the company had weak security." Read more here.
ALTR Maintained Market Momentum Throughout 2022
In March 2022, Q2 Holdings, Inc a leading provider of digital transformation solutions for banking and lending, and ALTR announced the long-term extension and expansion of their strategic technology partnership through 2026 to deliver unrivaled data governance and security to Q2 financial institution customers. Learn more here.
In June, ALTR announced the expansion of its partnership with Snowflake with the release of its new policy automation engine for managing data access controls in Snowflake and beyond. This solution allowed data engineers and architects to set up data access policies in minutes, manage ongoing updates to data permissions, and handle data access requests through ALTR’s own no-code platform for data policy management.
And in October, ALTR Co-founder and Chief Technology Officer, James Beecham, became ALTR’s newest Chief Executive Officer. Beecham leverages his technical acumen and passion for the industry and the business to lead the company's next phase of accelerated expansion. ALTR also appointed Co-founder and Vice President Engineering, Chris Struttmann, to the Chief Technology Officer position. Previous CEO David Sikora remains actively involved with ALTR as a Board Director, CEO Advisor and financial investor.
Looking Forward to 2023
We anticipate 2023 will continue to prove the urgency of focusing on the protection of your sensitive data, making now the time to create your action plan to protect your data. ALTR's low up-front-cost, no-code data governance solution is a great place to begin. Are you ready to take the next step in controlling access to your sensitive data? We can't wait to show you how ALTR can help.
Get a Demo
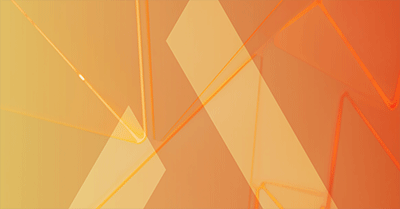
Dec 5
0
min
Snowflake Row-Level Security: DIY vs ALTR
ALTR Blog
Determining how to handle home security to protect your family is critical. After all, you don’t want to take risks when it comes to their safety. It’s an easy thing to put a lock on one door. But what about every door in the house? Every window? What if you need to handle security for the whole neighborhood? That’s when manual and DIY become unmanageable.
Snowflake database admins and data owners can run into the same issue with Snowflake Row-Level Security. While it may seem a simple task to set up one row access policy for one database using SQL in Snowflake, it quickly becomes overwhelming when you have hundreds of new users requesting access each week or thousands of rows of new data coming into your system daily.
In this blog post, we’ll explain what Snowflake Row-Level Security is and:
- Lay out the steps to set up Snowflake Row-Level Security policies manually using SQL vs setting up and managing these policies, with no code, in ALTR
- Provide examples of row-access policy use cases
- Show how using ALTR’s Row Access Policy feature can help minimize errors and make managing Snowflake row level security easier for anyone responsible for data security.
What is Snowflake Row-Level Security?
Snowflake’s row-level security allows you to hide or show individual rows in an SQL data table based on the user's role. This level of security gives you greater control of who you’re permitting to access sensitive data. For example, you may want to prevent personally identifiable data (PII) held in rows in a customer table from being visible to your call center agents based on the customers’ address. By using our ALTR Row Access Policy feature you will save:
- overhead costs from having to hire multiple developers to handle the work,
- developer time to manually write code, and
- effort to make configurations correctly when you need to restrict access to individual rows within a table.
How Creating Snowflake Row-Level Security Policies Works if you DIY
What’s involved to create a row-level security policy in Snowflake:
- Who can do it: A software developer who knows how to manually write SQL code
- Length of time to complete successfully: Hours or even days each week because the developer will have to manually do-it-yourself (DIY)
Each of the steps below requires code reviews, QA, validation, and maintenance that must be done. These tasks can cause this to take weeks to complete for each unique row access policy.
1. Grant the custom role to a user.
2. Write some code to determine if a table already has a row access policy defined.

3. Write some code to get the original row policy code if it was already defined.

4. Edit the code (or write new code) to implement the row access policy.

Step 4 is what will require most of your time because of everything that’s involved. For example, identifying all the criteria that could give a user access to a role, getting all department stakeholders to approve, turning those conditions into code, and having someone else to review that code and test it are all tasks to complete.
In addition, you’ll also need to make edits based on the code reviews and tests and constantly update the code each time the criteria changes.
How Creating Snowflake Row-Level Security Policies Works in ALTR
What’s involved to create a row access policy in ALTR:
- Who can do it: Anyone
- Length of time to complete successfully: Minutes because ALTR requires no code to implement and automates the security process
1. On the Row Access Policy page of our UI, select Add New.
This will allow you to specify the table that the Row Access Policy will apply to and the reference column that will control access.
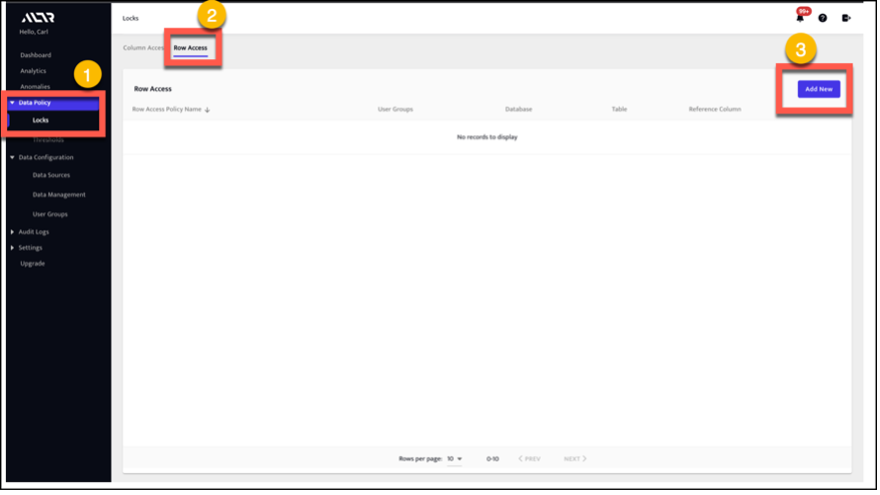
2. Indicate which Snowflake roles can access certain rows based on the values in a column. To do this, specify the mappings between User Groups (Snowflake Roles) and column values.
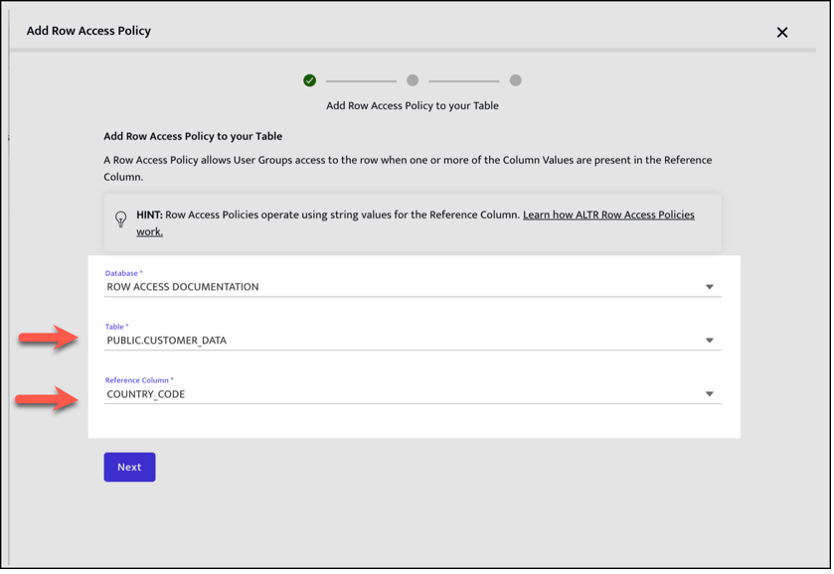
3. Review your policy, give it a name, click Submit, and you’re done. The name will be displayed in ALTR to reference the Row Access Policy. ALTR will convert the Row Access Policy into Snowflake. In just a few seconds, ALTR will insert the active policy into Snowflake!
Snowflake Row-Level Security Use Cases
Here are a couple of example use cases where our Row Access Policy feature in ALTR can benefit your business as it scales with your Snowflake usage.
USE CASE 1. Using Row-Level Policies to Enable Regional Sales Views
You have sales data that includes records for sales in all your sales regions. You only want your sales managers to see the data for the regions that they manage.
USE CASE 2. Using Row-Level Policies to Enable Separate Data Sets
You run a SaaS business and your customers want a data set for report of their transactions in your product; however, all the transactions are in a single table — the SaaS way.
What you could be doing: Automating your Snowflake Row-Level Security Policies with ALTR
Do you or your team have hours in a day to spend manually writing SQL code every time you need to create a unique row access policy for hundreds or thousands of users? Do you want to have to increase overhead by hiring multiple developers to manually create row access policies and manage them? Do you want to have to spend hours trying to figure out why a Snowflake row-access policy is not working correctly and you’re getting error messages?
While you can still choose to go down the SnowSQL do-it-yourself route, why not work smart instead of hard? Why risk data breaches and regulatory fines? Safeguard your data to make sure that only the right people have the right access.
By now, you have a better understanding of how using ALTR’s no-code platform enables users who don’t need to know SQL to create and manage Snowflake row level security through a simple point-and-click UI
Watch the “how-to” comparison video below to see manually setting up your own Snowflake Row Access Security Policy versus doing it with ALTR.
Ready to give it a try? Start with our Free Plan today
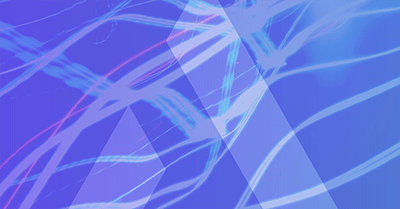
Nov 30
0
min
Cloud Data Migration: A Complete Guide
ALTR Blog
Cloud data migration is an inevitable part of every organization’s digital transformation journey. While a big data migration project can seem like an intimidating process, it doesn’t have to be. In fact, with the right preparation and implementation strategy, your organization can use your cloud migration as an opportunity to streamline internal processes, improve data security, reduce costs and gain insights.
To help you avoid some common pitfalls in your cloud data migration, we’ve put together this comprehensive guide with everything you need to know about moving data to the cloud. This post covers everything from why you should migrate to the cloud to what types of data you should migrate and how to do it securely. Let’s get started!
What is Cloud Data Migration?
Cloud data migration is the process of migrating data from on-premises systems to cloud-based systems. When migrating data to the cloud, it’s important to keep in mind that not all data is created equal. There are different types of data that each have unique needs when it comes to migration. While some data types can be migrated easily, others require a more careful approach that takes special considerations into account.
Why Tackle a Cloud Data Migration?
Cloud data migration is often a key step on the journey towards becoming a data-driven organization. Cloud data migration provides organizations with the opportunity to re-evaluate how they use data and make improvements to their data management processes. As part of your digital transformation journey, cloud migration allows you to transform data into a strategic asset by creating a centralized access point for all your organization’s data. This means data can be more easily retrieved, managed, and integrated across the enterprise. Moving data to the cloud not only provides access to more scalable computing resources than you may have on-premises, it also gives you admittance to a wide range of software-as-a-service (SaaS) apps that you can use to collaborate, process data, and collect insights. This access to a variety of business applications through a single user interface allows organizations to seamlessly integrate various functions and workflows within the organization.
How to Know Which Data to Include in Your Cloud Data Migration?
The best way to decide which data to migrate to the cloud is to start with your business objectives. Once you know what you want to achieve with your cloud data migration, you can start deciding which data to move. There are a few common objectives that most organizations have when it comes to data. These include:
- increasing employee productivity,
- improving customer experience, and
- boosting operational efficiency.
You should also consider moving data that is used frequently and is accessed by various departments. If a data source is critical to business operations, it should be migrated. This includes data such as employee data, customer data, and device data.
Migrating Device and Sensor Data to the Cloud
Moving device and sensor data to the cloud makes it easier to collect and analyze this type of data. This data can be collected from a wide variety of sources, including IoT devices and sensors. Moving device and sensor data to the cloud will allow you to store this data in a central location. Moving device and sensor data to the cloud will also make it easier to integrate this data with other systems such as data analytics tools and CRM systems like Salesforce. Doing so will help you generate more insightful business insights and make more strategic decisions.
Migrating Customer Data to the Cloud
Moving customer data to the cloud will give you access to a wide variety of customer data analytics tools. This will allow you to better understand your customer base and make strategic business decisions based on customer insights. Moving customer data to the cloud will give you access to data management tools that allow you to collect, organize, and analyze information such as customer data, purchase history, and account information. This will help you make more strategic business decisions, provide better customer service, and identify new business opportunities. Moving customer data to the cloud can also help you comply with data privacy regulations, including the GDPR.
Migrating Business-Critical Data to the Cloud
When deciding which data to migrate to the cloud, you should consider moving data that is most relevant to your business. Moving business-critical data to the cloud will give you access to more computing resources than what you may have on-premises and will allow you to scale up your data processing when needed. Moving business-critical data to the cloud will also deliver access a wide range of data analytics tools such as Tableau, Thoughtspot or Looker that will help you generate more helpful business insights.
Migrating Employee Data to the Cloud
Moving employee data to the cloud will give you access to cloud-based HR tools that can help you manage key business functions such as hiring, onboarding, and payroll. Moving data such as employee contact information, payroll data, internal and external communications, and customer information to the cloud can improve collaboration across departments by enabling real-time access to information. This access can be particularly beneficial for customer-facing teams such as sales and customer service.
.png)
Migrating Sensitive Data to the Cloud Securely
Employee data, device and sensor data, customer data and business critical data can all comprise sensitive information that is either regulated like personally identifiable information (PII) or simply extremely valuable to the company like intellectual property or payroll information. When moving that data away from company owned on-premises systems and data centers, controlling access and ensuring security throughout the journey is required. There are several places along the cloud data migration path that a cloud data security tool like tokenization can be implemented – from the on-prem warehouse to the cloud, as soon as it leaves the on-prem warehouse but before its handed off to an ETL, or as it enters the cloud data warehouse. The right process will often depend on how sensitive your data is and how regulated your industry is.
Cloud Data Migration Ecosystem
Part of moving data to the cloud is choosing the right tools for the migration itself (ETL), where to store and share the data (Cloud Data Warehouses), and how to analyze the data (Business Intelligence tools). Today's modern data ecosystem solutions are gathered and reviewed for you here. Your goal should be to build a modern data ecosystem tool stack that integrates easily and works together to deliver the data sharing and analytic goals of your cloud data migration project.
Wrapping up
If your organization has been using traditional systems, a cloud data migration may seem like an overwhelming process. To make it easier, first start by identifying the data types you want to migrate to the cloud. Moving customer data to the cloud, for example, will give you access to better customer analytics tools. Moving employee data to the cloud, on the other hand, will allow you to manage key business functions such as hiring, onboarding, and payroll. Knowing which data to move to the cloud is the first step towards successfully migrating to the cloud. Once you know what to migrate, and the security measures your data requires, you can start the process of planning and implementing your cloud data migration.
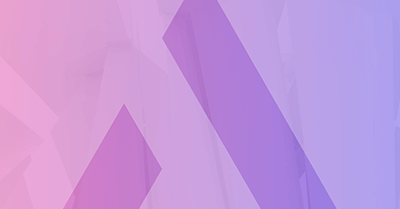
Nov 16
0
min
BIY Isn’t the Answer to Snowflake Data Access Control
ALTR Blog
In the last year, we’ve seen the awareness of the need for data access control and security in cloud data warehouses pass an inflection point. Most companies we talk to now, especially in the FinServ and Pharma industries, know they must have it. We don’t have to convince them sensitive data needs to be protected in the cloud or show them stats about data breaches or regulatory fines. They get it. But how they decide to get to it is a different story. Some decide to go down the do-it-yourself or build-it-yourself route, but I’m here to explain why you shouldn’t.
Automation Greases the Wheels
Identity providers like Okta and Active Directory have done a great job of enabling companies to automatically generate as many users and roles in Snowflake as needed. Today admins can go from 0 users to 1000 in about an hour or two.
On the other side of the equation, ETL providers like Matillion, FiveTran and Talend have made it easy for companies to transport their data into Snowflake. In an hour or two, admins can move gigabytes or even terabytes of source data and have it ready and waiting for users to access.
These two forces come to a head at the intersection between them: connecting users with data and defining the relationships between them. How do you make sure only the right users have access to only the data they should have?
Enter BIY Data Access Controls
Many companies start with DIY or do-it-yourself: the trusty Snowflake admin or DBA decides to write a handful of SnowSQL Snowflake data access control policies, one at a time. This works when you have one or two new users a week requesting access. But chances are, if you’re using an identity provider to create your profiles, you’re already dealing in hundreds or even thousands of users. DIY just doesn’t cut it – doing that work can suck up hours or even days each week, bringing access for new users as well as any other data projects to a halt, not to mention the human errors that can be introduced. It simply won’t scale.
Okay, so then our ingenious database admin thinks, “I can BIY this” or build-it-yourself. “I have a tool that puts my users in automatically. And I have a tool that puts my data in automatically. I can fix this problem if I just spend the next week writing a tool that automatically connects these two domains together. Easy-peasy.”
But wait, let’s take a step back and think about this. Snowflake also gives admins a way to add users without an identity management tool and add their data without an ETL tool. So, what’s the advantage of using an Okta or Matillion? The answer is reliability, scale and automation – those software vendors have built solutions that save you time and just do it better.
Risk of Crossing the Streams – User x Data
It’s ironic that of the tools they could create on their own, some companies focus on connecting users with data. Obviously, they’re doing this because they haven’t yet found the Okta or Matillion to handle this. But the irony is that this is the most dangerous spot in the process – that intersection is actually where all the risks are.
You can add data to Snowflake, and it’s pretty safe when users can’t get to it. And you can add users to Snowflake, but they can’t do much without access to data. Very rarely do you get in trouble for adding a wrong user or the wrong data. If users aren’t connected to the data, the risk is near zero. It’s in the middle part where the streams cross that is fraught with risk. Connecting the wrong user with the wrong data can be very bad for a data engineer, data steward, or privacy owner.
You could BIY, but Are You Enterprise-Ready?
So, an admin can write a quick and dirty Snowflake masking policy, but can others read and work with it? Do you have a QA team to eliminate errors? Once you get a proof-of-concept to work on one or two databases, can you ensure it scales correctly and can run quickly across thousands? Do you have the time to integrate it with Okta or Matillion or Splunk? Do you have a roadmap that ensures it’s staying in sync with new private-preview Snowflake features, keeping up with your changing data and regulatory landscape, and addressing new user service needs? Can you ensure it actually works correctly – did you build in feedback and alerting on fails and errors?
In other words, do you want to hire 30 engineers and spend millions of dollars to build enterprise-ready software you can trust with the risky connection between users and data?
Automated Snowflake Data Access Control for the Win
Wouldn’t it just be easier to grab a third leg of your stool for data access controls to go with your user role and data transfer solutions? That’s where ALTR comes in. We’ve already invested the time and resources to build a world-class, reliable solution that automates and enforces the connection between users and data. It leverages all of Snowflake’s native data governance features while adding a no-code layer that makes it easy to apply and manage. It also shows you how users are accessing data to be confident that data is shared correctly. And because it’s SaaS, it’s fast to implement, starts at a low cost and can scale with your Snowflake usage – to hundreds of users and thousands of databases. (You could even think of it as Okta for Data.)
Want to try it today? Sign up for our Free Plan. Or get a Demo to see how ALTR’s enterprise-ready solution can handle data access control for you. And avoid the BIY headache before it starts.

Nov 7
0
min
Nothing Better than a (Snowflake) Snowday
ALTR Blog
Today Christian Kleinerman (SVP Product, Snowflake) grouped his keynote announcements and discussions into three broad areas: core platform, data cloud content, and building on Snowflake.
There was certainly a bit of excitement in the air as it relates to partners at the keynote. Christian continued to emphasize partners, and he started off by repeating a thought from the other Data Cloud World Tours which is that Snowflake is one product. I think this message needs to remain in front of the partner mind: Snowflake will continue to encourage partners to invest in the single platform and will be unsupportive of any partner who wants to create a non-unified experience with Snowflake.
Snowflake Core Platform
Under the core platform pillar some of the big announcements centered on cross-cloud availability and replication which can make Snowflake a much safer platform to run your business on, especially at global scale. One big shout out for partners was the announcement around data listing analytics. If you are a data provider partner for Snowflake, this is a big win for you. Understanding how consumers are using your data listings will ensure you can make the best decisions as you look to add or remove data sets.
For the governance and security partners, these cross-cloud replication and failovers might cause some issues depending upon how your solution is implemented. For users of tokenization or external functions in general, we know there might still be some need for Snowflake to continue to invest in this area so governance and security features can also seamlessly failover.
Snowflake Data Cloud Content
The second pillar around data cloud content was focused on producing applications and workloads on Snowflake. Partners like EY, phData and Infosys were specifically called out. And it was clear these partners were in the crowd as there was some unexpected cheering! If you are not thinking about building an application natively inside of Snowflake this part of the talk would have you reconsidering. A new partner/ecosystem new startup called Samooha was brought on stage. In under 6 months, the company went from mockup to full working product to help build clean rooms within Snowflake. They noted it was still really new but showcases how quickly you can build an MVP and bring a value-added process to market directly in Snowflake.
Building on Snowflake
Snowpark for Python is now GA! This was the biggest announcement from the last segment around building on snowflake. They actually produced fake snow in the room to make it a true ‘snowday’! Partners can now have a secure single place in Snowflake to share data and run python models directly on data inside of snowflake. This is huge for partners. Christian noted a 6x uptick in usage since the initial announcement at Snowflake Summit ‘22.
Streamlit can be run directly in Snowflake, which will make building and selling an application inside of Snowflake much easier, was a big deal as well. This will make it much easier for users to consume these applications as data will no longer need to leave Snowflake.
It was great to see Sri Chintala on the stage. I remember two years ago when we were first looking at becoming a Snowflake partner, Sri was one of the first product folks we talked with. At the time she was leading the external function group which ALTR utilized heavily. Now she is working with python use cases and got the chance to demo her latest work on stage. It’s amazing to see the product folks mature and with that maturity continue to bring partners, like Anaconda who Sri mentioned on stage, along with them. She also did a good job handling those microphone issues!
All in all, it was another exciting Snowday, and ALTR is looking forward to the next six months as a Snowflake partner. I’ll be posting some thoughts on what I hear throughout the day on LinkedIn. Catch me there until next time!

Oct 26
0
min
Modern Data Ecosystem Tools - A Complete Guide
ALTR Blog
What is the Modern Data Ecosystem?
Today’s business environment is awash with data. From product development intellectual property (IP) to customer personally identifiable information (PII) to logistics and supply chain information, data is coming at us from all directions. And that data is making its way throughout the business in ways that it never did before.
In the past, your customer and prospect data may have stayed securely behind a firewall in a customer database in a company-owned datacenter. But from the moment Salesforce launched its pioneering Software-as-a-Service CRM, that data has been moving into the cloud. And the volume has only increased. Now, cloud data platforms like Snowflake and Amazon Redshift offer anyone the ability to host and analyze data with just a credit card and a spreadsheet. This has opened a pandora’s box of data analysis possibilities that comes with attendant challenges and risks.
By now most companies understand the significant opportunities presented by living in the “Age of Data.” Recently, a data ecosystem of technologies has developed to help organizations take advantage of these new opportunities. In fact, so many new tools, solutions and technologies have appeared that choosing solutions for a modern data ecosystem can be almost as difficult as dealing with data itself.
We put together this guide to help clear the clutter and explain who does what in the modern data ecosystem and how it can help your organization become more data-driven more quickly.
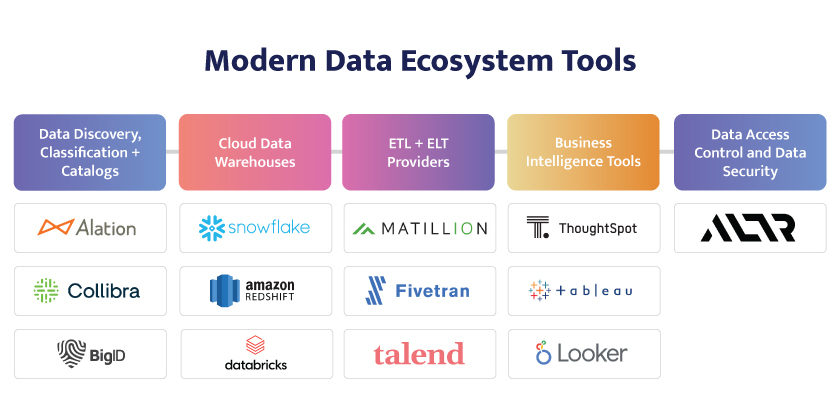
Your Data Ecosystem Guide
Data Discovery, Classification, and Catalogs
The rapid growth of data collection, security threats, and regulatory requirements has transformed what was previously an esoteric process conversation into a mainstream business challenge. It’s now a strategic priority for any organization to apply and enforce data governance standards, not just the traditional regulated industries like finance and healthcare. However, data owners must tread carefully to avoid running up against privacy laws like GDPR and CCPA: Gartner believes that modern privacy regulations will cover 75% of the world in a couple of years.
Many vendors focus on “knowing” your data—where it is (discovery), what is it (classification), where it came from (data lineage). Industry analysts call this “metadata management,” or getting a handle on the data itself. Data discovery, classification and cataloging are the critical first steps of a big data ecosystem.
Alation
Alation is credited with creating the data catalog product category – an early building block of the modern data ecosystem. Its signature software, the Alation Data Catalog, serves enterprises in organizing and consolidating their data. Alation’s enterprise data catalog dramatically improves the productivity of analysts, increases the accuracy of analytics, and drives confident data-driven decision making while empowering everyone in your organization to find, understand, and govern data.
BigID
BigID offers software for managing sensitive and private data, completely rethinking data discovery and intelligence for the privacy era. BigID was the first company to deliver enterprises the technology to know their data to the level of detail, context and coverage they would need to meet core data privacy protection requirements. BigID’s data intelligence platform enables organizations to take action for privacy, protection, and perspective. Organizations can deploy BigID to proactively discover, manage, protect, and get more value from their regulated, sensitive, and personal data across their data landscape.
Collibra
Collibra calls itself “The Data Intelligence Company.” They aim to remove the complexity of data management to give you the perfect balance between powerful analytics and ease of use. The company’s premier offering is its data catalog – a single solution for teams to easily discover and access reliable data. It allows companies to provide users access to trusted data across all your data sources. Delivering this end-to-end visibility starts with your data catalog, and Collibra gets you up and running in days. With Collibra’s scalable platform, you can future-proof your investment, no matter where business takes you next.
Cloud Data Warehouses
While the cloud migration started with specific workloads moving to SaaS services (think Salesforce or Office 365), today the data ecosystem is focused on, well, data. The same advantages of SaaS – low up-front costs, no hardware to maintain, no datacenter to staff and service, no upgrades to track – all apply to the modern cloud data warehouse. In addition, data storage combined with compute enables companies to consolidate data from across the company and make it easily available for analysis and insight. Data-driven companies find this service invaluable.
Snowflake Data Cloud
Snowflake offers a cloud-based data storage and analytics service that allows users to store and analyze data using cloud-based hardware and software. Snowflake’s founders engineered Snowflake to power the Data Cloud, where thousands of organizations have smooth access to explore, share, and unlock the full value of their data. Today, 1300 Snowflake customers have more than 250PB of data managed by the Data Cloud, with more than 515 million data workloads that run each day.
Amazon Redshift
According to the company, tens of thousands of companies rely on Amazon Redshift to analyze exabytes of data with complex analytical queries, making it the most widely used cloud data warehouse. Users can run and scale analytics in seconds on all their data without having to manage a data warehouse infrastructure. Amazon Redshift uses SQL to analyze structured and semi-structured data across data warehouses, operational databases, and data lakes. With AWS-designed hardware and machine learning, the service can deliver the best price performance at any scale. The company also offers a Free Tier.
Databricks
The Databricks Lakehouse Platform combines the best elements of data lakes and data warehouses to deliver the reliability, strong governance and performance of data warehouses with the openness, flexibility and machine learning support of data lakes.
This unified approach simplifies your modern data stack by eliminating the data silos that traditionally separate and complicate data engineering, analytics, BI, data science and machine learning. It’s built on open source and open standards to maximize flexibility. And, its common approach to data management, security and governance helps you operate more efficiently and innovate faster.
ETL and ELT Providers
Another significant piece of the data ecosystem puzzle are ETL and ELT providers. Consolidating business data in cloud data warehouses like Snowflake is a smart move that can open up new doors of innovation and value. All your data in one place makes it easier to connect the dots in ways that were impossible or unimaginable before. For instance, a retail chain can optimize sales projections by analyzing weather patterns, or a logistics company can more accurately predict costs by accounting for the salaries of all the people involved in a shipment.
Getting to those insights is a process that starts with moving the data. An extract, transform, and load (ETL) migration technology partner simplifies moving or loading the data from each of your company’s locations into a cloud data warehouse to make it analytics-ready in no time. Moving data is what these companies do best.
Matillion
Matillion’s complete data integration and transformation solution is purpose-built for the cloud and cloud data warehouses. The company’s flagship tool, Matillion ETL, is specifically for cloud database platforms including Amazon Redshift, Google BigQuery, Snowflake and Azure Synapse. It is a modern, browser-based UI, with powerful, push-down ETL/ELT functionality. Matillion ETL pushes down data transformations to your data warehouse and process millions of rows in seconds, with real-time feedback. The browser-based environment includes collaboration, version control, full-featured graphical job development, and more than 20 data read, write, join, and transform components. Users can launch and be developing ETL jobs within minutes. Matillion offers a free trial.
Fivetran
Focused on automated data integration, Fivetran delivers ready-to-use connectors that automatically adapt as schemas and APIs change, ensuring consistent, reliable access to data. In fact, the company says it offers the industry’s best selection of fully managed connectors. Their pipelines automatically and continuously update, freeing users up to focus on game-changing insights instead of ETL. They improve the accuracy of data-driven decisions by continuously synchronizing data from source applications to any destination, allowing analysts to work with the freshest possible data. To accelerate analytics, Fivetran automates in-warehouse transformations and programmatically manages ready-to-query schemas. Fivetran offers a free trial.
Talend
According to Talend integrating your data doesn't have to be complicated or expensive. Talend Cloud Integration Platform simplifies your ETL or ELT process, so your team can focus on other priorities. With over 900 components, you can move data from virtually any source to your data warehouse more quickly and efficiently than by hand-coding alone. Talent helps reduce spend, accelerate time to value, and deliver data you can trust.
You can download a free trial of Talend Cloud Integration.
Business Intelligence (BI) and Analytics Tools
Most business data users aren’t running database queries but accessing data and gaining insights via business intelligence tools (BI) that provide services including reporting, online analytical processing, analytics, dashboard , data mining, complex event processing, business performance management, benchmarking, text mining, predictive analytics, and prescriptive analytics. As the front door to data for technical and line-of-business users throughout the company, finding a friendly, flexible, accessible BI solution is key.
Tableau
Tableau is an interactive data visualization software company focused on business intelligence. Tableau products query relational databases, online analytical processing cubes, cloud databases, and spreadsheets to generate graph-type data visualizations. The software can also extract, store, and retrieve data from an in-memory data engine. Tableau allows organizations to ensure the responsible use of data and drive better business outcomes with fully-integrated data management and governance, visual analytics and data storytelling, and collaboration—all with Salesforce’s industry-leading Einstein built right in. Companies can lower the barrier to entry for users to engage and interact by building visualizations with drag and drop, employing AI-driven statistical modeling with a few clicks, and asking questions using natural language. Tableau provides efficiencies of scale to streamline governance, security, compliance, maintenance, and support with solutions for the entire lifecycle as the trusted environment for your data and analytics—from connection, preparation, and exploration to insights, decision-making, and action.
ThoughtSpot
ThoughtSpot believes the world would be a better place if everyone had quicker, easier access to facts. Their search and AI-driven analytics platform makes it simple for anyone across the organization to ask and answer questions with data. It empowers colleagues, partners, and customers to turn data into actionable insights via the ThoughtSpot application, embedding insights into apps like Salesforce and Slack, or building entirely new data products. The consumer-grade search and AI technology delivers true self-service analytics that anyone can use, while the developer-friendly platform ThoughtSpot Everywhere makes it easy to build interactive data apps that integrate with users’ existing cloud ecosystem.
Looker
Looker Data & Analytics is business intelligence software and big data analytics platform that helps users explore, analyze and share real-time business analytics easily. Now part of Google Cloud, it offers a wide variety of tools for relational database work, business intelligence, and other related services. Looker utilizes a simple modeling language called LookML that lets data teams define the relationships in their database so business users can explore, save, and download data with only a basic understanding of SQL.[2] The product was the first commercially available business intelligence platform built for and aimed at scalable or massively parallel relational database management systems like Amazon Redshift, Google BigQuery and more.
Data Access Control and Data Security
ALTR is the only automated data access control and security solution that allows organizations to easily govern and protect sensitive data – enabling users to distribute more data to more end users more securely, more quickly. Hundreds of companies and thousands of users leverage ALTR’s platform to gain unparalleled visibility into data usage, automate data access controls and policy enforcement, and secure data with patented rate-limiting and tokenization-as-a-service. ALTR’s partner data ecosystem integrations with data catalogs, ETL, cloud data warehouses and BI services enable scalable on-premises-to-cloud protection. Our free integration with Snowflake allows admins to get started in minutes instead of months and scale up as you expand your data use, user base and databases.
The Evolving Data Ecosystem
ALTR continues to develop relationships with cloud data leaders across the industry. Our goal is to help our customers to get the most from their data by enabling a secure cloud data ecosystem that allows users to safely share and analyze sensitive data. Our scalable cloud platform acts as the foundation by enabling seamless integration with a wide variety of enterprise tools used to ingest, transform, store, govern, secure, and analyze data. ALTR has expanded how we interact with data ecosystem leaders via open-source integrations that allow users to freely and easily extend ALTR's data control and security to data catalogs like Alation and ETL tools like Matillion. Building a modern data ecosystem stack will set you firmly on the path to secure data-driven leadership.
Get the latest from ALTR
Subscribe below to stay up to date with our team, upcoming events, new feature releases, and more.
Thank you! Your submission has been received!
Oops! Something went wrong while submitting the form.