BLOG SPOTLIGHT
ALTR’s Format-Preserving Encryption, powered by FF3-1 algorithm and ALTR’s trusted policies, offers a comprehensive solution for securing sensitive data.
Read more
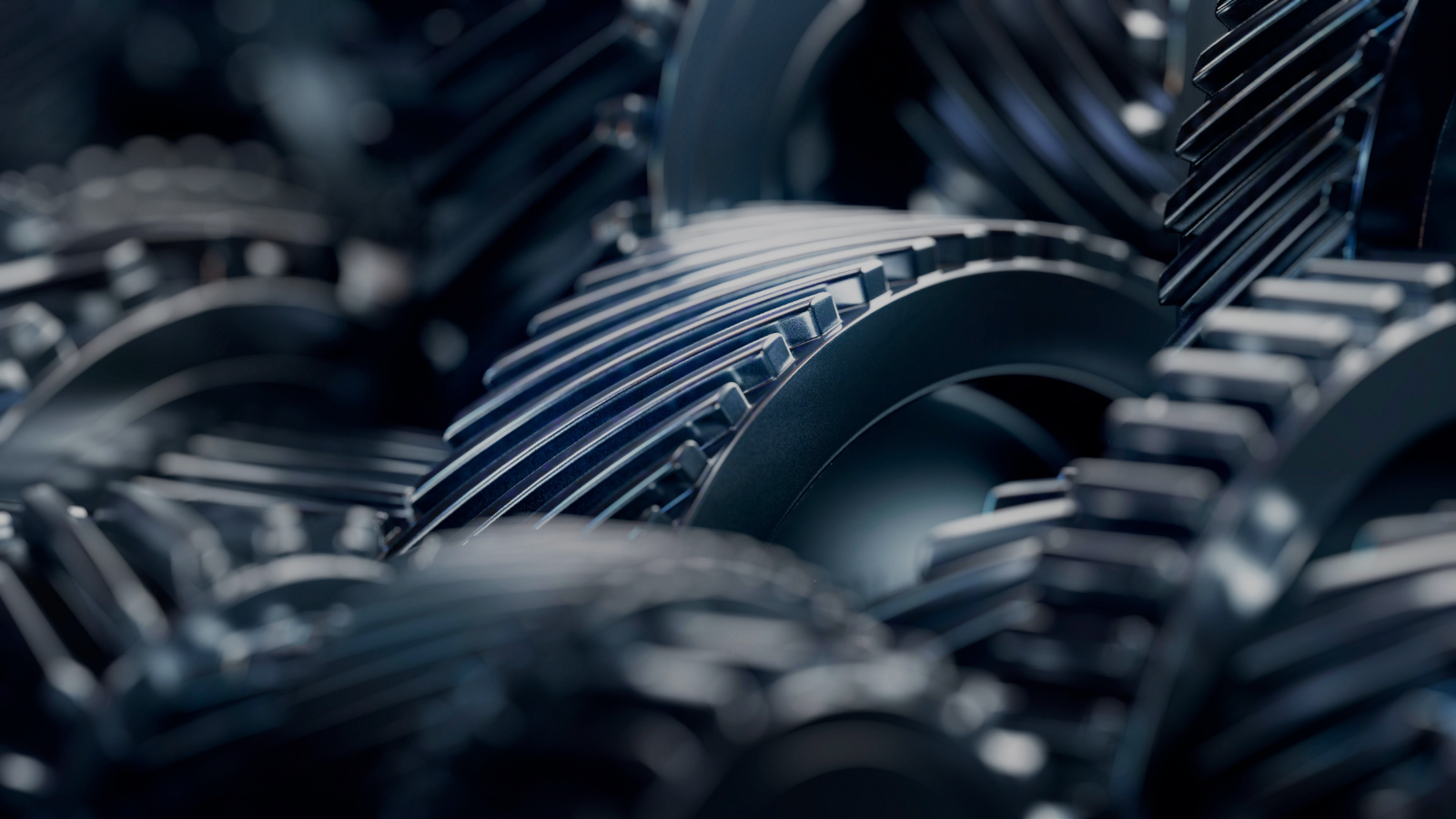
Popular
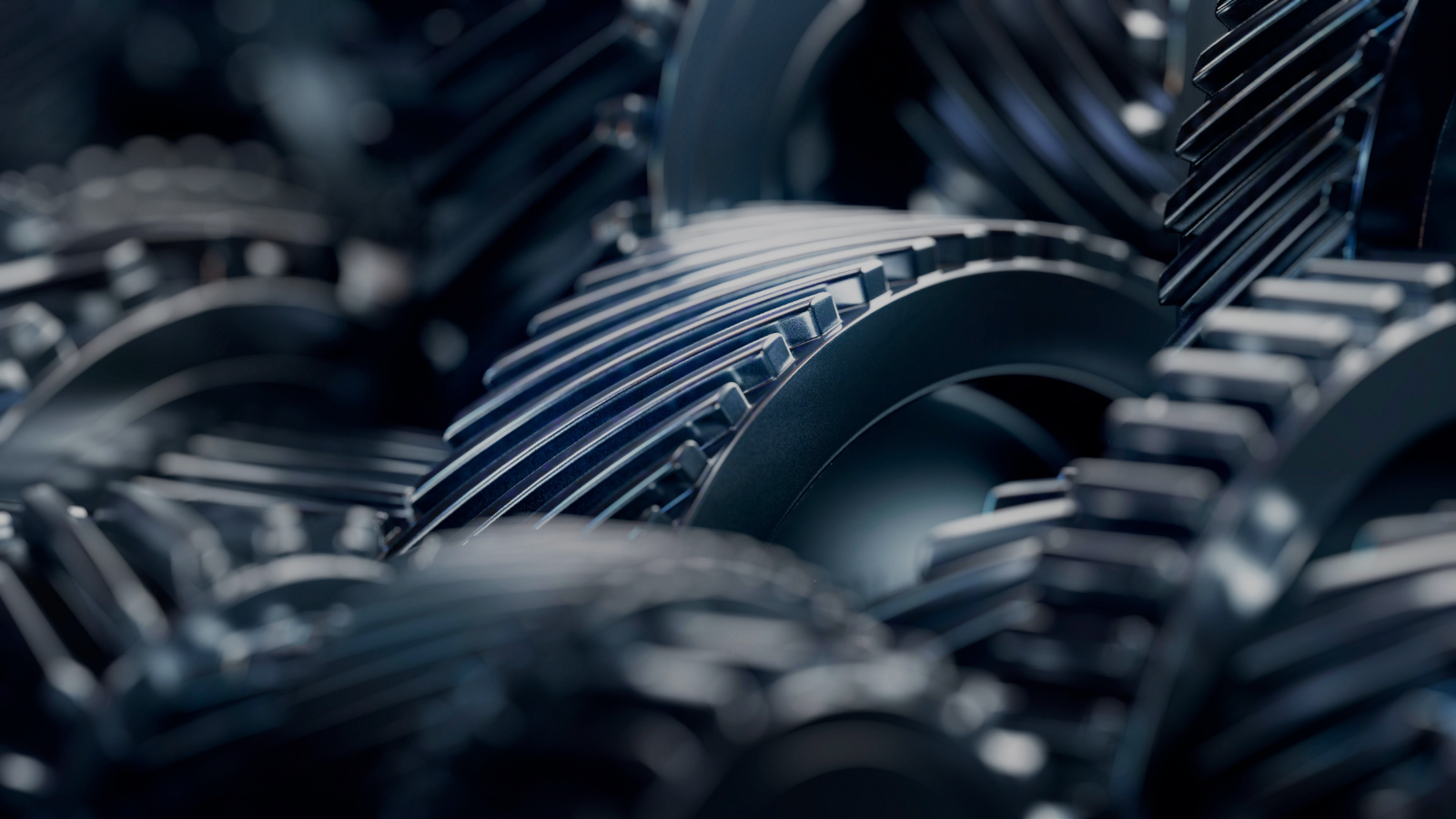
Oct 30
0
min
Format-Preserving Encryption: A Deep Dive into FF3-1 Encryption Algorithm
In the ever-evolving landscape of data security, protecting sensitive information while maintaining its usability is crucial. ALTR’s Format Preserving Encryption (FPE) is an industry disrupting solution designed to address this need. FPE ensures that encrypted data retains the same format as the original plaintext, which is vital for maintaining compatibility with existing systems and applications. This post explores ALTR's FPE, the technical details of the FF3-1 encryption algorithm, and the benefits and challenges associated with using padding in FPE.
What is Format Preserving Encryption?
Format Preserving Encryption is a cryptographic technique that encrypts data while preserving its original format. This means that if the plaintext data is a 16-digit credit card number, the ciphertext will also be a 16-digit number. This property is essential for systems where data format consistency is critical, such as databases, legacy applications, and regulatory compliance scenarios.
Technical Overview of the FF3-1 Encryption Algorithm
The FF3-1 encryption algorithm is a format-preserving encryption method that follows the guidelines established by the National Institute of Standards and Technology (NIST). It is part of the NIST Special Publication 800-38G and is a variant of the Feistel network, which is widely used in various cryptographic applications. Here’s a technical breakdown of how FF3-1 works:
Structure of FF3-1
1. Feistel Network: FF3-1 is based on a Feistel network, a symmetric structure used in many block cipher designs. A Feistel network divides the plaintext into two halves and processes them through multiple rounds of encryption, using a subkey derived from the main key in each round.
2. Rounds: FF3-1 typically uses 8 rounds of encryption, where each round applies a round function to one half of the data and then combines it with the other half using an XOR operation. This process is repeated, alternating between the halves.
3. Key Scheduling: FF3-1 uses a key scheduling algorithm to generate a series of subkeys from the main encryption key. These subkeys are used in each round of the Feistel network to ensure security.
4. Tweakable Block Cipher: FF3-1 includes a tweakable block cipher mechanism, where a tweak (an additional input parameter) is used along with the key to add an extra layer of security. This makes it resistant to certain types of cryptographic attacks.
5. Format Preservation: The algorithm ensures that the ciphertext retains the same format as the plaintext. For example, if the input is a numeric string like a phone number, the output will also be a numeric string of the same length, also appearing like a phone number.
How FF3-1 Works
1. Initialization: The plaintext is divided into two halves, and an initial tweak is applied. The tweak is often derived from additional data, such as the position of the data within a larger dataset, to ensure uniqueness.
2. Round Function: In each round, the round function takes one half of the data and a subkey as inputs. The round function typically includes modular addition, bitwise operations, and table lookups to produce a pseudorandom output.
3. Combining Halves: The output of the round function is XORed with the other half of the data. The halves are then swapped, and the process repeats for the specified number of rounds.
4. Finalization: After the final round, the halves are recombined to form the final ciphertext, which maintains the same format as the original plaintext.
Benefits of Format Preserving Encryption
Implementing FPE provides numerous benefits to organizations:
1. Compatibility with Existing Systems: Since FPE maintains the original data format, it can be integrated into existing systems without requiring significant changes. This reduces the risk of errors and system disruptions.
2. Improved Performance: FPE algorithms like FF3-1 are designed to be efficient, ensuring minimal impact on system performance. This is crucial for applications where speed and responsiveness are critical.
3. Simplified Data Migration: FPE allows for the secure migration of data between systems while preserving its format, simplifying the process and ensuring compatibility and functionality.
4. Enhanced Data Security: By encrypting sensitive data, FPE protects it from unauthorized access, reducing the risk of data breaches and ensuring compliance with data protection regulations.
5. Creation of production-like data for lower trust environments: Using a product like ALTR’s FPE, data engineers can use the cipher-text of production data to create useful mock datasets for consumption by developers in lower-trust development and test environments.
Security Challenges and Benefits of Using Padding in FPE
Padding is a technique used in encryption to ensure that the plaintext data meets the required minimum length for the encryption algorithm. While padding is beneficial in maintaining data structure, it presents both advantages and challenges in the context of FPE:
Benefits of Padding
1. Consistency in Data Length: Padding ensures that the data conforms to the required minimum length, which is necessary for the encryption algorithm to function correctly.
2. Preservation of Data Format: Padding helps maintain the original data format, which is crucial for systems that rely on specific data structures.
3. Enhanced Security: By adding extra data, padding can make it more difficult for attackers to infer information about the original data from the ciphertext.
Security Challenges of Padding
1. Increased Complexity: The use of padding adds complexity to the encryption and decryption processes, which can increase the risk of implementation errors.
2. Potential Information Leakage: If not implemented correctly, padding schemes can potentially leak information about the original data, compromising security.
3. Handling of Padding in Decryption: Ensuring that the padding is correctly handled during decryption is crucial to avoid errors and data corruption.
Wrapping Up
ALTR's Format Preserving Encryption, powered by the technically robust FF3-1 algorithm and married with legendary ALTR policy, offers a comprehensive solution for encrypting sensitive data while maintaining its usability and format. This approach ensures compatibility with existing systems, enhances data security, and supports regulatory compliance. However, the use of padding in FPE, while beneficial in preserving data structure, introduces additional complexity and potential security challenges that must be carefully managed. By leveraging ALTR’s FPE, organizations can effectively protect their sensitive data without sacrificing functionality or performance.
For more information about ALTR’s Format Preserving Encryption and other data security solutions, visit the ALTR documentation
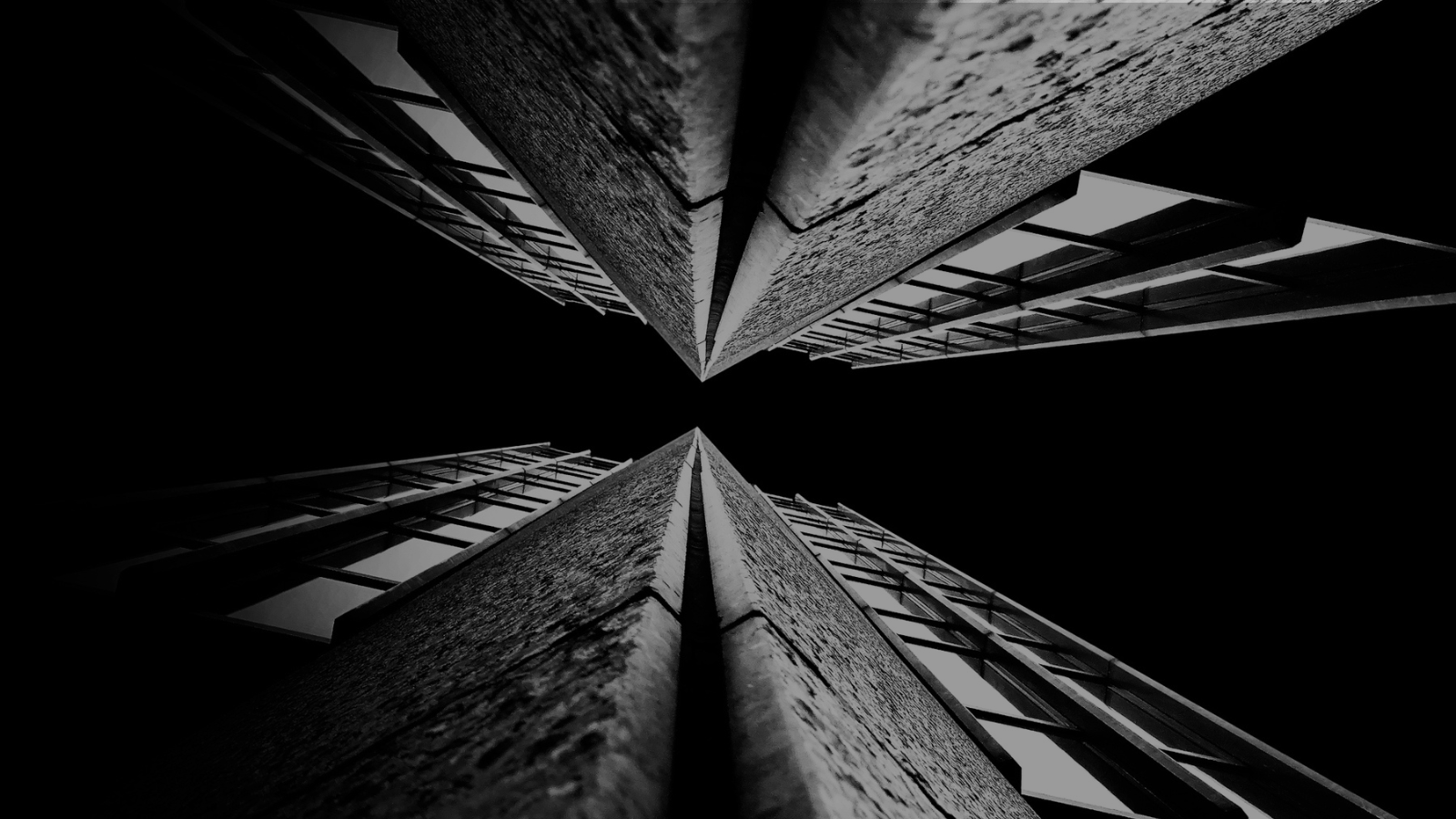
Oct 22
0
min
The CISO's Dilemma: Securing Snowflake's ACCOUNTADMIN Role
For years (even decades) sensitive information has lived in transactional and analytical databases in the data center. Firewalls, VPNs, Database Activity Monitors, Encryption solutions, Access Control solutions, Privileged Access Management and Data Loss Prevention tools were all purchased and assembled to sit in front of, and around, the databases housing this sensitive information.
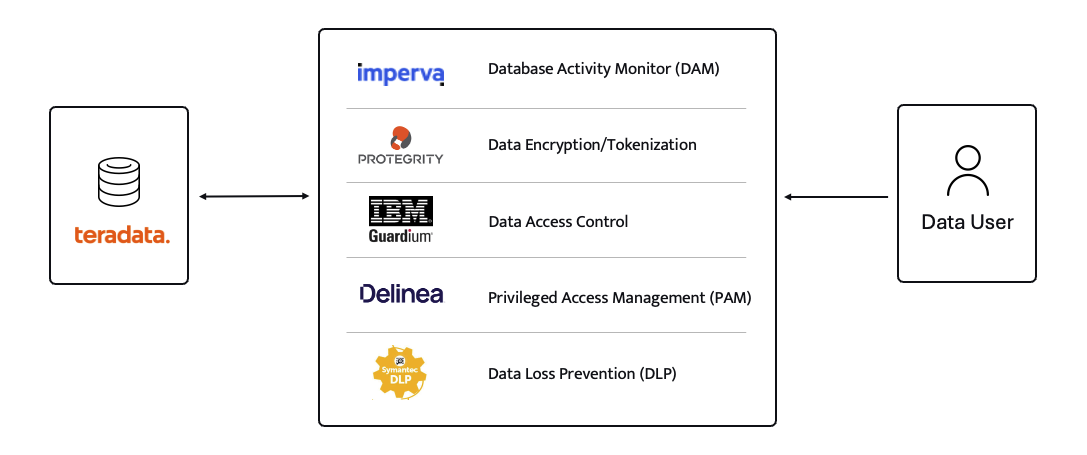
Even with all of the above solutions in place, CISO’s and security teams were still a nervous wreck. The goal of delivering data to the business was met, but that does not mean the teams were happy with their solutions. But we got by.
The advent of Big Data and now Generative AI are causing businesses to come to terms with the limitations of these on-prem analytical data stores. It’s hard to scale these systems when the compute and storage are tightly coupled. Sharing data with trusted parties outside the walls of the data center securely is clunky at best, downright dangerous in most cases. And forget running your own GenAI models in your datacenter unless you can outbid Larry, Sam, Satya, and Elon at the Nvidia store. These limits have brought on the era of cloud data platforms. These cloud platforms address the business needs and operational challenges, but they also present whole new security and compliance challenges.
ALTR’s platform has been purpose-built to recreate and enhance these protections required to use Teradata for Snowflake. Our cutting-edge SaaS architecture is revolutionizing data migrations from Teradata to Snowflake, making it seamless for organizations of all sizes, across industries, to unlock the full potential of their data.
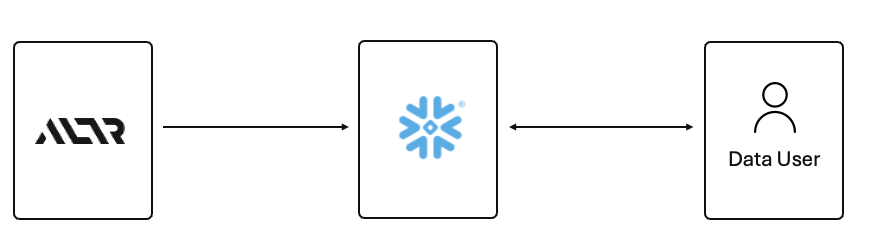
What spurred this blog is that a company reached out to ALTR to help them with data security on Snowflake. Cool! A member of the Data & Analytics team who tried our product and found love at first sight. The features were exactly what was needed to control access to sensitive data. Our Format-Preserving Encryption sets the standard for securing data at rest, offering unmatched protection with pricing that's accessible for businesses of any size. Win-win, which is the way it should be.
Our team collaborated closely with this person on use cases, identifying time and cost savings, and mapping out a plan to prove the solution’s value to their organization. Typically, we engage with the CISO at this stage, and those conversations are highly successful. However, this was not the case this time. The CISO did not want to meet with our team and practically stalled our progress.
The CISO’s point of view was that ALTR’s security solution could be completely disabled, removed, and would not be helpful in the case of a compromised ACCOUNTADMIN account in Snowflake. I agree with the CISO, all of those things are possible. Here is what I wanted to say to the CISO if they had given me the chance to meet with them!
The ACCOUNTADMIN role has a very simple definition, yet powerful and long-reaching implications of its use:

One of the main points I would have liked to make to the CISO is that as a user of Snowflake, their responsibility to secure that ACCOUNTADMIN role is squarely in their court. By now I’m sure you have all seen the news and responses to the Snowflake compromised accounts that happened earlier this year. It is proven that unsecured accounts by Snowflake customers caused the data theft. There have been dozens of articles and recommendations on how to secure your accounts with Snowflake and even a mandate of minimum authentication standards going forward for Snowflake accounts. You can read more information here, around securing the ACCOUNTADMIN role in Snowflake.
I felt the CISO was missing the point of the ALTR solution, and I wanted the chance to explain my perspective.
ALTR is not meant to secure the ACCOUNTADMIN account in Snowflake. That’s not where the real risk lies when using Snowflake (and yes, I know—“tell that to Ticketmaster.” Well, I did. Check out my write-up on how ALTR could have mitigated or even reduced the data theft, even with compromised accounts). The risk to data in Snowflake comes from all the OTHER accounts that are created and given access to data.
The ACCOUNTADMIN role is limited to one or two people in an organization. These are trusted folks who are smart and don’t want to get in trouble (99% of the time). On the other hand, you will have potentially thousands of non-ACCOUNTADMIN users accessing data, sharing data, screensharing dashboards, re-using passwords, etc. This is the purpose of ALTR’s Data Security Platform, to help you get a handle on part of the problem which is so large it can cause companies to abandon the benefits of Snowflake entirely.
There are three major issues outside of the ACCOUNTADMIN role that companies have to address when using Snowflake:
1. You must understand where your sensitive is inside of Snowflake. Data changes rapidly. You must keep up.
2. You must be able to prove to the business that you have a least privileged access mechanism. Data is accessed only when there is a valid business purpose.
3. You must be able to protect data at rest and in motion within Snowflake. This means cell level encryption using a BYOK approach, near-real-time data activity monitoring, and data theft prevention in the form of DLP.
The three issues mentioned above are incredibly difficult for 95% of businesses to solve, largely due to the sheer scale and complexity of these challenges. Terabytes of data and growing daily, more users with more applications, trusted third parties who want to collaborate with your data. All of this leads to an unmanageable set of internal processes that slow down the business and provide risk.
ALTR’s easy-to-use solution allows Virgin Pulse Data, Reporting, and Analytics teams to automatically apply data masking to thousands of tagged columns across multiple Snowflake databases. We’re able to store PII/PHI data securely and privately with a complete audit trail. Our internal users gain insight from this masked data and change lives for good.
- Andrew Bartley, Director of Data Governance
I believed the CISO at this company was either too focused on the ACCOUNTADMIN problem to understand their other risks, or felt he had control over the other non-admin accounts. In either case I would have liked to learn more!
There was a reason someone from the Data & Analytics team sought out a product like ALTR. Data teams are afraid of screwing up. People are scared to store and use sensitive data in Snowflake. That is what ALTR solves for, not the task of ACCOUNTADMIN security. I wanted to be able to walk the CISO through the risks and how others have solved for them using ALTR.
The tools that Snowflake provides to secure and lock down the ACCOUNTADMIN role are robust and simple to use. Ensure network policies are in place. Ensure MFA is enabled. Ensure you have logging of ACCOUNTADMIN activity to watch all access.
I wish I could have been on the conversation with the CISO to ask a simple question, “If I show you how to control the ACCOUNTADMIN role on your own, would that change your tone on your teams use of ALTR?” I don’t know the answer they would have given, but I know the answer most CISO’s would give.
Nothing will ever be 100% secure and I am by no means saying ALTR can protect your Snowflake data 100% by using our platform. Data security is all about reducing risk. Control the things you can, monitor closely and respond to the things you cannot control. That is what ALTR provides day in and day out to our customers. You can control your ACCOUNTADMIN on your own. Let us control and monitor the things you cannot do on your own.

Oct 14
0
min
How Cloud Data Security Enables Business Outcomes without Sacrificing Compliance
Since 2015 the migration of corporate data to the cloud has rapidly accelerated. At the time it was estimated that 30% of the corporate data was in the cloud compared to 2022 where it doubled to 60% in a mere seven years. Here we are in 2024, and this trend has not slowed down.
Over time, as more and more data has moved to the cloud, new challenges have presented themselves to organizations. New vendor onboarding, spend analysis, and new units of measure for billing. This brought on different cloud computer-related cost structures and new skillsets with new job titles. Vendor lock-in, skill gaps, performance and latency and data governance all became more intricate paired with the move to the cloud. Both operational and transactional data were in scope to reap the benefits promised by cloud computing, organizational cost savings, data analytics and, of course, AI.
The most critical of these new challenges revolve around a focus on Data Security and Privacy. The migration of on-premises data workloads to the Cloud Data Warehouses included sensitive, confidential, and personal information. Corporations like Microsoft, Google, Meta, Apple, Amazon were capturing every movement, purchase, keystroke, conversation and what feels like thought we ever made. These same cloud service providers made this easier for their enterprise customers to do the same. Along came Big Data and the need for it to be cataloged, analyzed, and used with the promise of making our personal lives better for a cost. The world's population readily sacrificed privacy for convenience.
The moral and ethical conversation would then begin, and world governments responded with regulations such as GDPR, CCPA and now most recently the European Union’s AI Act. The risk and fines have been in the billions. This is a story we already know well. Thus, Data Security and Privacy have become a critical function primarily for the obvious use case, compliance, and regulation. Yet only 11% of organizations have encrypted over 80% of their sensitive data.
With new challenges also came new capabilities and business opportunities. Real time analytics across distributed data sources (IoT, social media, transactional systems) enabling real time supply chain visibility, dynamic changes to pricing strategies, and enabling organizations to launch products to market faster than ever. On premise applications could not handle the volume of data that exists in today’s economy.
Data sharing between partners and customers became a strategic capability. Without having to copy or move data, organizations were enabled to build data monetization strategies leading to new business models. Now building and training Machine Learning models on demand is faster and easier than ever before.
To reap the benefits of the new data world, while remaining compliant, effective organizations have been prioritizing Data Security as a business enabler. Format Preserving Encryption (FPE) has become an accepted encryption option to enforce security and privacy policies. It is increasingly popular as it can address many of the challenges of the cloud while enabling new business capabilities. Let’s look at a few examples now:
Real Time Analytics - Because FPE is an encryption method that returns data in the original format, the data remains useful in the same length, structure, so that more data engineers, scientists and analysts can work with the data without being exposed to sensitive information.
Data Sharing – FPE enables data sharing of sensitive information both personal and confidential, enabling secure information, collaboration, and innovation alike.
Proactive Data Security– FPE allows for the anonymization of sensitive information, proactively protecting against data breaches and bad actors. Good holding to ransom a company that takes a more proactive approach using FPE and other Data Security Platform features in combination.
Empowered Data Engineering – with FPE data engineers can still build, test and deploy data transformations as user defined functions and logic in stored procedures or complied code will run without failure. Data validations and data quality checks for formats, lengths and more can be written and tested without exposing sensitive information. Federated, aggregation and range queries can still run without fail without the need for decryption. Dynamic ABAC and RBAC controls can be combined to decrypt at runtime for users with proper rights to see the original values of data.
Cost Management – While FPE does not come close to solving Cost Management in its entirety, it can definitely contribute. We are seeing a need for FPE as an option instead of replicating data in the cloud to development, test, and production support environments. With data transfer, storage and compute costs, moving data across regions and environments can be really expensive. With FPE, data can be encrypted and decrypted with compute that is a less expensive option than organizations' current antiquated data replication jobs. Thus, making FPE a viable cost savings option for producing production ready data in non-production environments. Look for a future blog on this topic and all the benefits that come along.
FPE is not a silver bullet for protecting sensitive information or enabled these business use cases. There are well documented challenges in the FF1 and FF3-1 algorithms (another blog on that to come). A blend of features including data discovery, dynamic data masking, tokenization, role and attribute-based access controls and data activity monitoring will be needed to have a proactive approach towards security within your modern data stack. This is why Gartner considers a Data Security Platform, like ALTR, to be one of the most advanced and proactive solutions for Data security leaders in your industry.

Oct 2
0
min
What is Format-Preserving Encryption & Why It’s the Missing Piece in Your Security Strategy
Securing sensitive information is now more critical than ever for all types of organizations as there have been many high-profile data breaches recently. There are several ways to secure the data including restricting access, masking, encrypting or tokenization. These can pose some challenges when using the data downstream. This is where Format Preserving Encryption (FPE) helps.
This blog will cover what Format Preserving Encryption is, how it works and where it is useful.
What is Format Preserving Encryption?
Whereas traditional encryption methods generate ciphertext that doesn't look like the original data, Format Preserving Encryption (FPE) encrypts data whilst maintaining the original data format. Changing the format can be an issue for systems or humans that expect data in a specific format. Let's look at an example of encrypting a 16-digit credit card number:

As you can see with a Standard Encryption type the result is a completely different output. This may result in it being incompatible with systems which require or expect a 16-digit numerical format. Using FPE the encrypted data still looks like a valid 16-digit number. This is extremely useful for where data must stay in a specific format for compatibility, compliance, or usability reasons.
>>>You Might Also Like: FPE vs Tokenization vs TSS
How does Format Preserving Encryption work?
Format Preserving Encryption in ALTR works by first analyzing the column to understand the input format and length. Next the NIST algorithm is applied to encrypt the data with the given key and tweak. ALTR applies regular key rotation to maximize security. We also support customers bringing their own keys (BYOK). Data can then selectively be decrypted using ALTR’s access policies.
Why use Format Preserving Encryption
FPE offers several benefits for organizations that deal with structured data:
1. Adds extra layer of protection: Even if a system or database is breached the encryption makes sensitive data harder to access.
2. Original Data Format Maintained: FPE preserves the original data structure. This is critical when the data format cannot be changed due to system limitations or compliance regulations.
3. Improves Usability: Encrypted data in an expected format is easier to use, display and transform.
4. Simplifies Compliance: Many regulations like PCI-DSS, HIPAA, and GDPR will mandate safeguarding, such as encryption, of sensitive data. FPE allows you to apply encryption without disrupting data flows or reporting, all while still meeting regulatory requirements.
When to use Format Preserving Encryption?
FPE is widely adopted in industries that regularly handle sensitive data. Here are a few common use cases:
- Healthcare: Hospitals and healthcare providers could use FPE to protect Social Security numbers, patient IDs, and medical records. It ensures sensitive information is encrypted while retaining the format needed for billing and reporting.
- Telecoms: Telecom companies can encrypt phone numbers and IMSI (International Mobile Subscriber Identity) numbers with FPE. This allows the data to be securely transmitted and processed in real-time without decryption.
- Government and Defense: Government agencies can use FPE to safeguard data like passport numbers and classified information. Preserving the format ensures seamless data exchange across systems without breaking functionality.
- Data Sharing: In this blog we talk about how FPE can help with Snowflake Data Sharing use cases.
Wrapping Up
ALTR offers various masking, tokenization and encryption options to keep all your Snowflake data secure. Our customers are seeing the benefit of Format Preserving Encryption to enhance their data protection efforts while maintaining operational efficiency and compliance. For more information, schedule a product tour or visit the Snowflake Marketplace.
Browse All
Thank you! Your submission has been received!
Oops! Something went wrong while submitting the form.

Aug 24
0
min
Snowflake Masking Policy: DIY vs ALTR
ALTR Blog
There’s nothing worse than when you lose the remote to your TV. All you want to do is sit on the couch and change the channel or the volume at your leisure — but when you don’t have a remote you have to get up, walk over to the tv, click the “next channel” button twenty-five times until you get to the channel you want, then walk all the way back to the couch to sit down, exhausted. Oh, then you realize it’s too loud, and now you have to do the whole thing all over again. It’s downright infuriating.
But if you didn’t know that a remote existed, you probably wouldn’t mind it so much, right? If that’s all you ever had, it would seem normal. This is a good way to think about how ALTR works when it comes to Snowflake Masking Policy. You can do dynamic data masking in Snowflake without us, but it's a heck of a lot easier to do it with us.
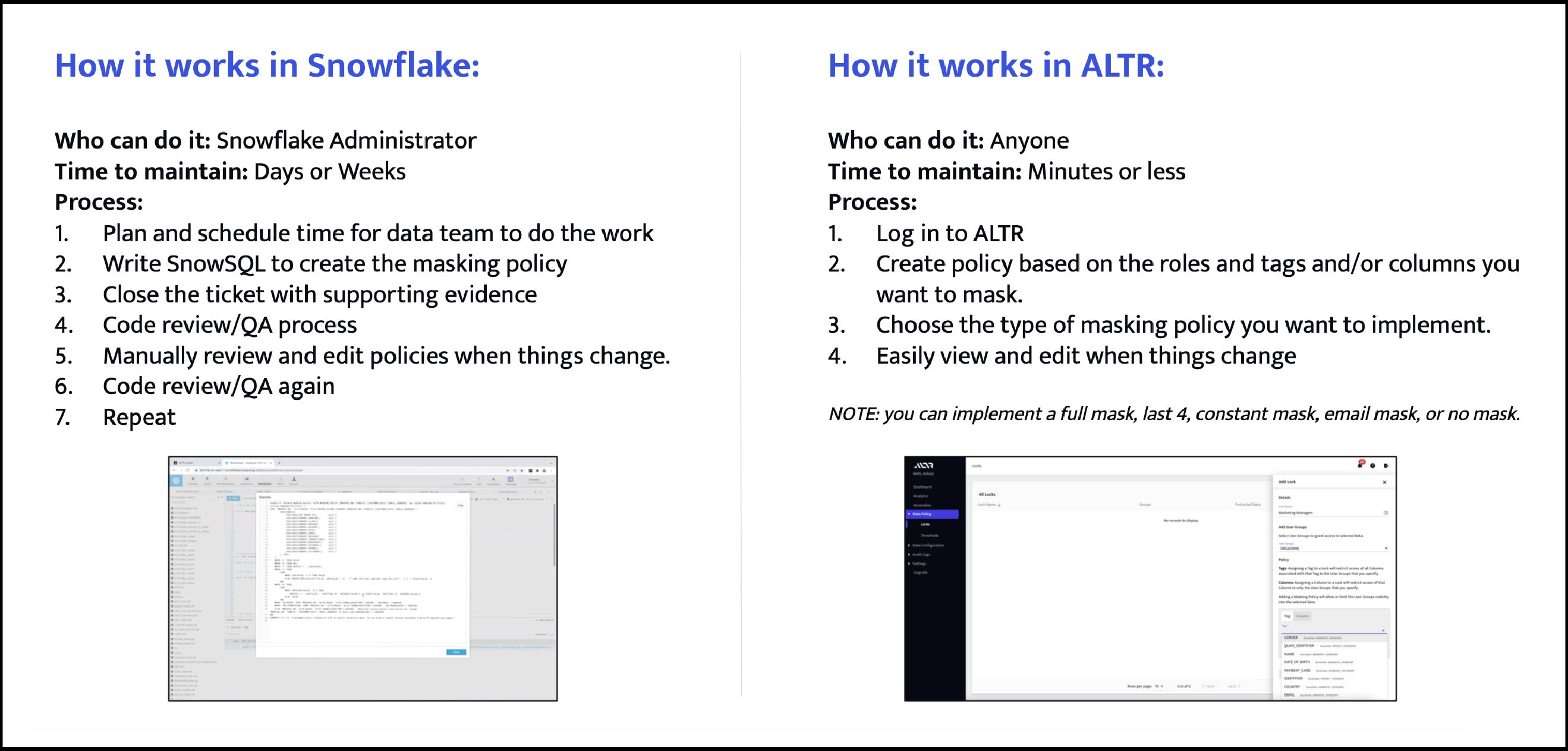
What you do now: write your Snowflake masking policy using SnowSQL
Generally, writing a Snowflake masking policy requires roughly 40 lines of SnowSQL per policy. Depending on your business, that can turn into 4,000 lines real quick. And then you have to test to make sure it works as intended. And then you have to go through QA. And then you have to update it and start the process all over. The process can feel endless. Just like going from channel 12 to channel 209 without a remote, it’s exhausting and tedious.
If you look at Snowflake’s documentation, you’ll see that creating a Snowflake masking policy requires 5 steps:
1. Grant Snowflake masking policy privileges to custom role
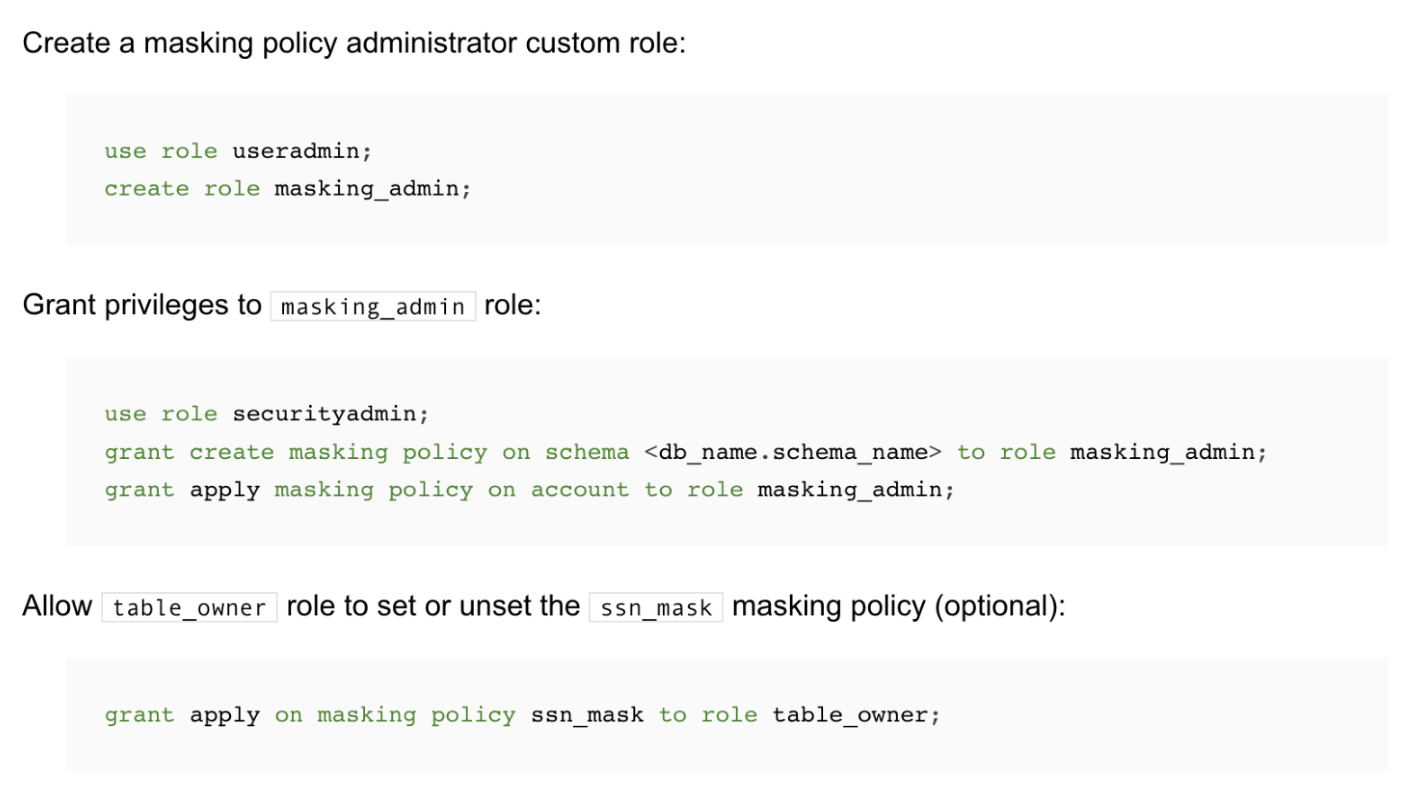
2. Grant the custom role to a user

3. Create a Snowflake masking policy
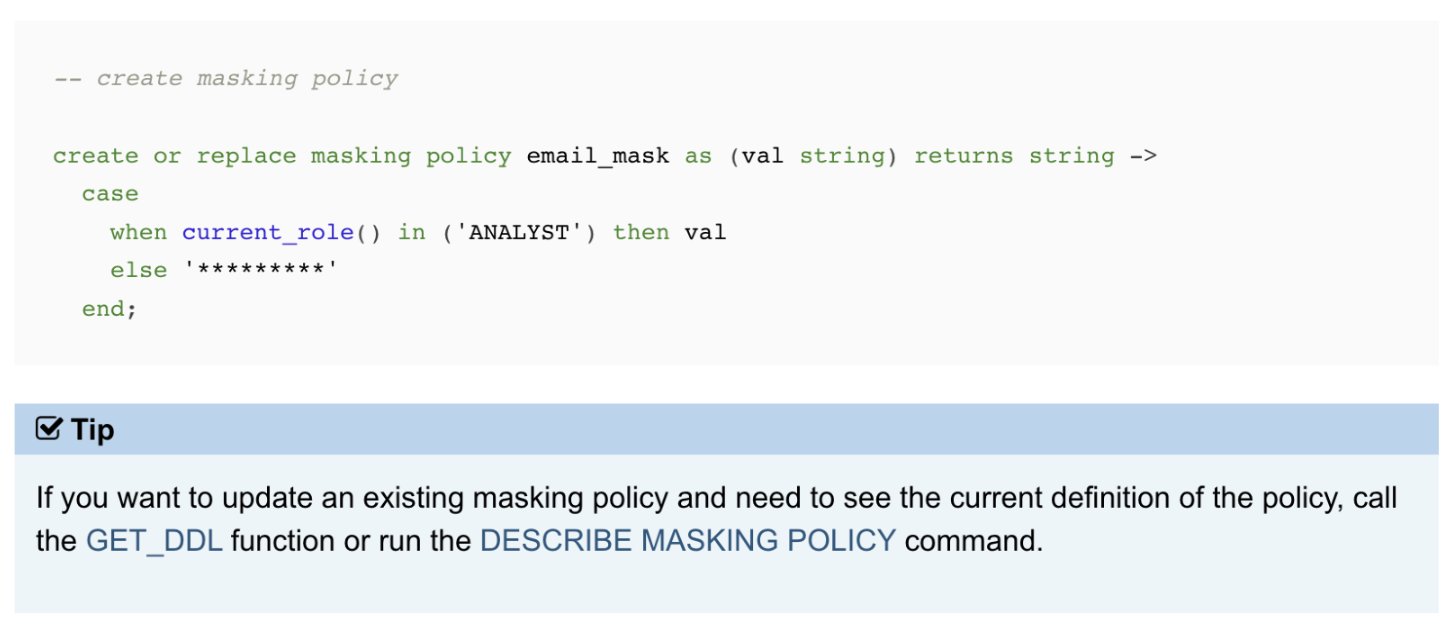
4. Apply the Snowflake masking policy to a table or view column

5. Query data in Snowflake
That’s just to get started with a basic Snowflake Masking Policy! If you want to apply different types, like a partial mask, time stamp, UDF, etc. then you’ll need to refer back to the documentation again. To get more advanced with Snowflake tag-based or row-level policy, you’ll need another deep dive.
The big kicker here is the amount of time it takes to code not only the initial policies, but to update them and test them over time. No matter how good anyone is at SnowSQL, there’s always room for human error that can lead to frustration at best and at worst to dangerous levels of data access.
So, what if you could automate the Snowflake masking policy process? What if you could use a remote to do it for you to save time and keep things streamlined for your business?
What you could be doing: automating Snowflake masking policy with ALTR
Setting a sensitive data masking policy in ALTR is like clicking “2-0-9" on your remote when a commercial comes on channel 12; you log in, head to the Locks tab, and use ALTR’s interface to set a Snowflake masking policy that has already been tested for you. And when something changes in your org, you log back in and update your data masking policy or add a new one with just a few clicks.
Here’s exactly how that works:
1. Navigate to Data Policy --> Locks --> Add new
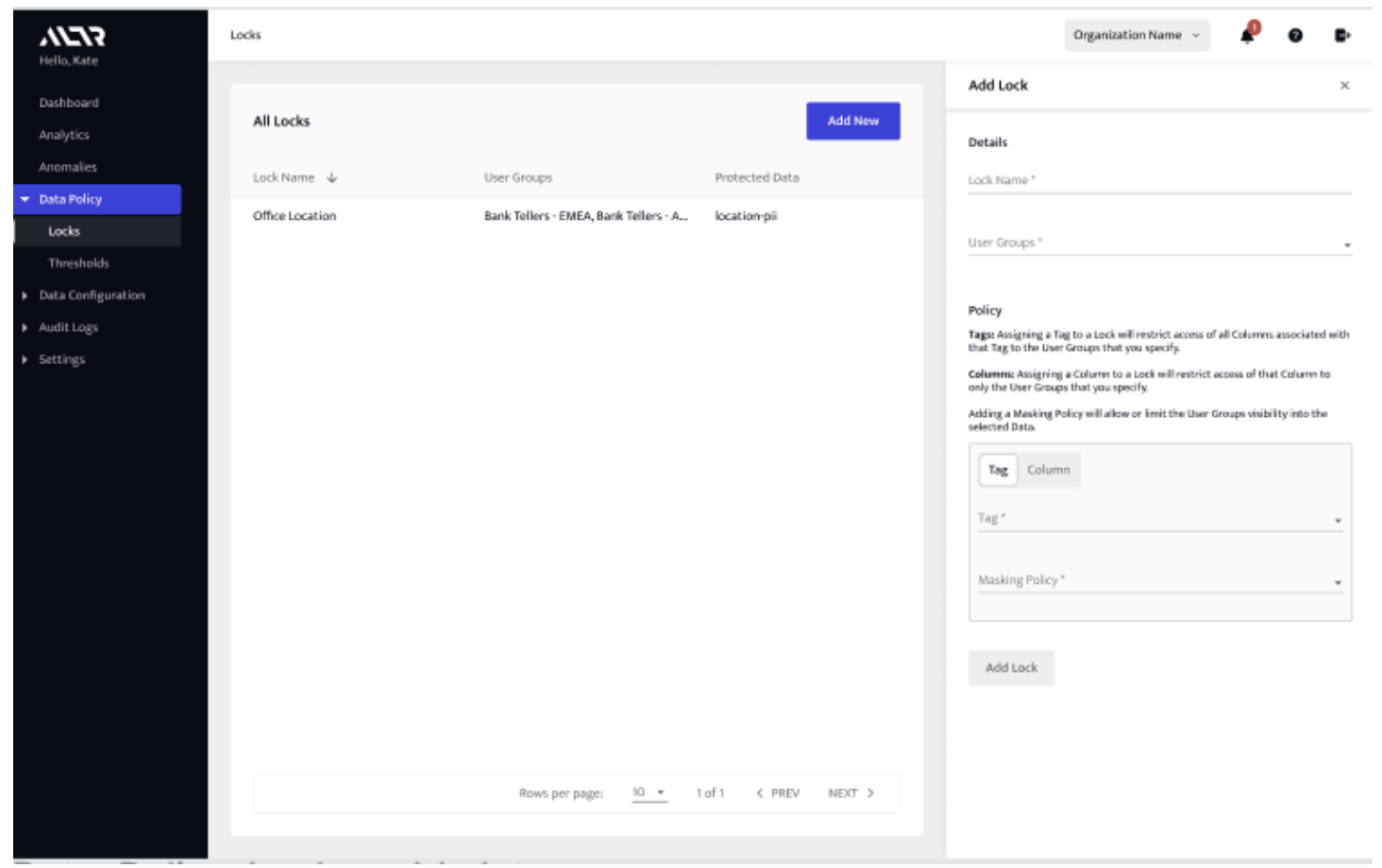
2. Fill out the lock details: name, user groups affected.
3. Choose which data to apply policy to, then choose the dynamic data masking type you’d like to use (full mask, email, show last four of SSN, no mask, or constant mask).
a. Column-based data masking (sensitive columns have been classified and added for ALTR to govern)
b. Tag-based data masking (tags are defined either by Google DLP, Snowflake classification, Snowflake object tags, or tags imported from a data catalog integration).
4. (Optional) Add another data masking policy.
5. Click “Add Lock”
That’s it; there’s no code required, and anyone in the business can set up a Snowflake masking policy if they have the right Snowflake permissions. To update or remove a lock, all you have to do is edit the existing policy using the same interface.
ALTR’s data masking in Snowflake policies are not only easy to implement, but they leverage Snowflake’s native capabilities, like Snowflake tag-based masking. That means that ALTR is not only the most cost-effective method, but it ensures that your policy works best with Snowflake.
Check out this video below to see what it looks like to set Snowflake Masking Policy manually versus doing it in ALTR:
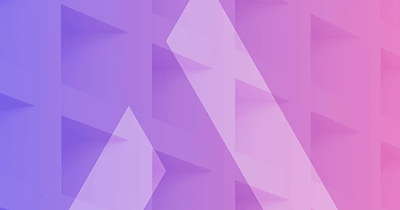
Sep 14
0
min
Why You Need an “Okta” for Cloud Data Access
ALTR Blog
SaaS platforms have exploded in the last few years for good reason: they offer unprecedented scalability, cost, accessibility, flexibility. But like any explosion, it left some messes in its wake. For IT and security teams in particular, the increasing number of solutions used by teams throughout the company created a seemingly never-ending need to add users, remove users, or change permissions every time some joined, changed roles, shifted responsibilities, or left the company altogether. As is often the case, IT and security teams took up the slack managing and maintaining user permissions manually – going into each platform, adding each new user, setting permissions and doing it over and over again, each time a change occurred.
This led to delays, risk of error or even users skipping the authorization process altogether. According to Gigaom research, 81% of employees admitted to using unauthorized SaaS applications, and in an IDG report 73% of IT leaders agreed that keeping track of identity and permissions across environments is a primary challenge. If onboarding new employees was painful, off-boarding was even worse. If IT forgot a service, then a past employee could still have access they shouldn’t. Talk about a security issue!
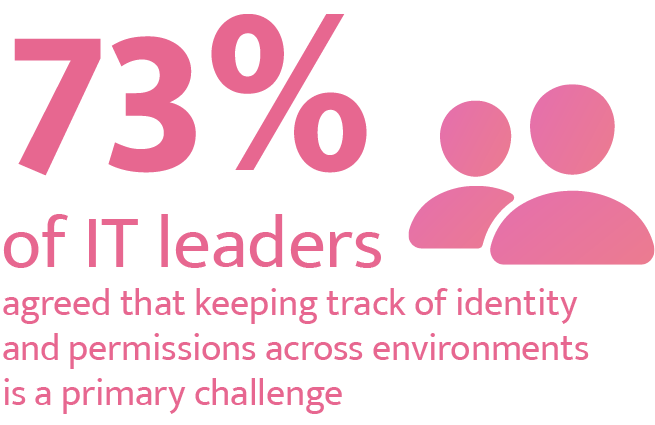
Okta Automates User Account Management
Then in 2009, along came Okta. Built on top of the Amazon Web Services cloud, Okta’s single sign-on service allows users to log into multiple systems using one central process. Okta automatically creates all your user accounts when an employee comes on, then automatically disables or deactivates them when an employee leaves. You can still always go into each service and make changes, but why? Okta is SaaS-based, you can start for free and then it’s just a couple of dollars per user per month after that. Okta also expanded to integrate with other solutions to simplify the overall onboarding process. For example, using ServiceNow when a new employee is hired triggers the building manager to generate a new badge, Okta to generate user accounts, and HR to generate payroll forms.
At a certain point, it became stupid not to use Okta, and today the service has more than 300 million users and 15k+ customers. So that takes care of the first wave of cloud migration: users moving to SaaS platforms. But what about the next migration: data moving to cloud platforms?
Why Shouldn’t We Have Okta for Cloud Data Access Control?
If the Okta model worked for software permission provisioning, why couldn’t something similar be the answer for cloud data access control and security? Setting individual or role-based user data access policies correctly is critical, but perhaps even more critical is the confidence that access is revoked when needed – all automated, all error-free. In addition, Okta’s ease of use allowed it to be utilized by groups outside IT, like marketing and sales teams who were early SaaS adopters. Since data, just like software, is often owned, controlled and migrated by groups outside IT, shouldn’t managing data access and security be just as flexible and user-friendly?
From DIY Cloud Data Access Control to D-I...Why?
Okta’s (and many automated solutions’) biggest early competitor was “do-it-yourself.” If you’ve always been able to handle users and data access control manually, it can seem like making the shift to a new process would just add more work. But it’s a little like the frog in the pot – the temp is rising but you don’t realize you’re boiling until it’s too late. Maybe setting up a new data user took 10 minutes just a year ago, but today you’re dealing with hundreds of requests a week; and something that was a snap to do manually on a small scale is now taking up hours of your time. It’s when you realize that your data projects have moved from minimal viable product/beta stage to full production with hundreds of users across the enterprise, that you may wake up one day and realize you no longer have any time to enable data projects because you’re so busy enabling data users.
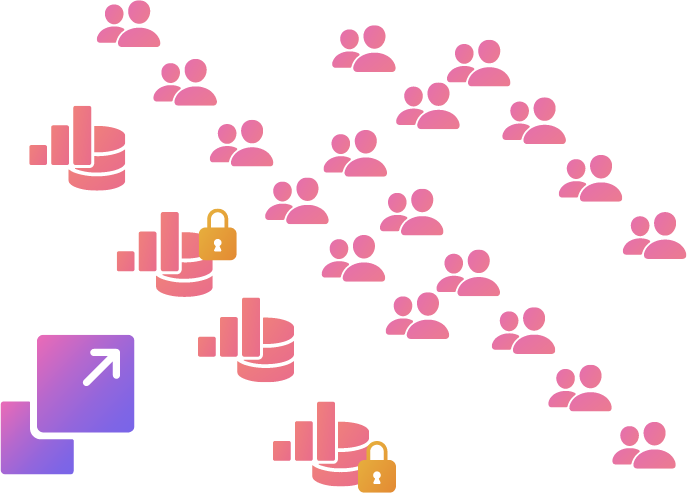
ALTR Automates Cloud Data Access Control
Okta is a low lift, SaaS-delivered, zero-up pricing solution that eliminates burdensome manual provisioning of user access to software and integrates with multiple systems to automate the onboarding process. Sound familiar? We believe that ALTR is the “Okta for data.” We massively simplify provisioning data access controls at scale and integrate with the top-to-bottom modern data stack to reduce error and risk and increase efficiency.
And if you don’t think you need it today, just look back at the journey from manual software permissions to Okta. It’s only a matter of time before data access follows the same path. Wouldn’t it be great to get out of the pot BEFORE it’s boiling?
See how easy and scalable automated data access control can be in Snowflake with ALTR. Try ALTR Free!
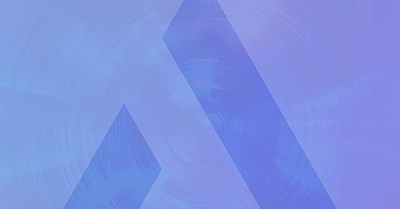
Aug 17
0
min
Tokenization vs Encryption: Which is Best for Cloud Data Security?
ALTR Blog
ALTR CEO James Beecham has compared encryption to duct tape. Duct tape is great - it comes in handy when you need a quick fix for a thousand different things or even...to seal a duct. But when it comes to security, you need powerful tools that are fit for purpose.
Today, let’s compare some different methods you could use to secure data - including tokenization vs encryption - to see which is the best fit for your cloud data security.
Tokenization vs Encryption: 3 Reasons to Choose Tokenization
As a data security company, ALTR uses encryption for some things, but when we looked at encryption vs tokenization, we found tokenization far superior for two key data security needs:
- Defeating data thieves
- Enabling data analysis
Companies that want to transform data into business value need both security and analytics. Tokenization delivers the best of both worlds: the strong at-rest protection of encryption and the analysis opportunity provided by similar solutions like anonymization.
3 ways tokenization is superior to encryption:
1. Tokenization is more secure.
It actually replaces the original data with a token, so if someone successfully obtains the digital token, they have nothing of value. There’s no key and no relationship to the original data. The actual data remains secure in a separate token vault.
This is important because we now collect all kinds of information as a society. Companies want to analyze the customer data they hold, whether it’s Netflix, a hospital or a bank. If you’re using encryption to protect the data, you must first decrypt it all to make any use of it or any sense of it. And decrypting leads to data risk.
2. Tokenization enables analytics.
Because tokenization offers determinism, which which maintains the same relationship between a token and the source data every time, accurate analytics can be performed on data in the cloud.
If you provide a particular set of inputs, you get the same outputs every time. Deterministic tokens represent a piece of data in an obfuscated way and give you back the same token or representation when you need it. The token can be a mashup of numbers, letters and symbols, just like an encrypted piece of data, but tokens preserve relationships. The real benefit of deterministic tokenization is allowing analysts to connect two datasets or databases securely, protecting PII privacy while allowing analysts to run their data operations.
3. Tokenization maintains the source data.
Because the connection is two way – tokenization and de-tokenization - you can retrieve the original data in the event if you need it.
Let’s say you’ve collected instrument readings from a personal medical device that I own. If you detect something in that data, like performance degradation, you and I both would appreciate my getting a phone call, an email or a letter informing me I need to replace the device. Encryption would not allow this because once data is encrypted, such as my name or phone number, it disappears forever from the database.

Tokenization vs Anonymization: Limited Analytics Today and Tomorrow
Unlike encryption, anonymization offers some ability to perform fundamental analysis, but is limited by the anonymization data design and intent. Anonymization removes all the PII by grouping data into ranges, like age range or zip code while removing their birthdate and street address. This means you can perform a level of analysis on anonymized data, say on your 18 to 25 years old customers. But what if you wanted a different group or associate that age range with another data set?
Anonymization is permanent and inflexible. The process cannot be reversed to re-identify individuals, which might not give you enough options. If your team wants to follow an initial data run to invite a group of customers to an event or send them an offer, you’re stuck without the phone number or mailing address available. There’s no relationship to the original PII of the individual.
Tokenization vs Hashing: A One-Way Trip
Another data security tool is one-way hashing. This is a form of cryptographic security that uses an algorithm to convert source data into an anonymized piece of data of a specific length. Unlike encryption, because the data is a fixed length and the same hash means the same data, it can be operated on with joins. But a big downside is that it’s (virtually) irreversible. So, like anonymization, once the data is converted, it cannot be turned back into plain text or source data for further analysis. Hashing is most often used to protect passwords stored in databases. You may also hear the term “salting” applied to password hashing. This is the practice of adding additional values to the end of the hashed password to differentiate the value, making the password cracking process much harder. Hashing works very well for password protection but is not ideal for PII that needs to be used.
Encryption, anonymization and one-way hashing, therefore, can be shortsighted moves. Your organization’s success depends on allowing authorized users to access the original data now and in the future, as long as you can track and report on the usage. At the same time, you must also ensure that sensitive data is useless to everyone else.
.png)
Tokenization: The Clear Cloud Data Security Winner
When looking at tokenization vs encryption, it's clear that tokenization overcomes the challenges other data security solutions face by preserving the connections and relationships between data columns and sets. However, tokenization isn’t just a simple mathematical scramble of the original data like encryption or a group of ranges with anonymized data. Authorized analysts can query tokenized data for insights without having access to the underlying PII. The more secure token remains meaningless to any unauthorized user or hacker.
With modern tokenization techniques, you can apply policies and authorize access at scale for thousands of users. You can also track and report on the secure access of sensitive data to ensure compliance with privacy regulations worldwide. You can’t do this with anonymization, hashing or encryption.
When it comes to tokenization vs encryption, tokenization is the more flexible tool for secure access and privacy compliance. This is critical for organizations quickly moving from storing gigabytes to petabytes of data in the cloud. You can feed tokenized data directly from cloud data warehouses like Snowflake into any application. You can do this with complete confidence that all the data, including sensitive PII, will be protected even from the database admin while making it easy for authorized data end-users to collaborate and deliver valuable insight quickly. Isn’t that the whole point?
See how ALTR can integrate with leading data catalog and ETL solutions to deliver automated tokenization from on-premises to the cloud. Get a demo.
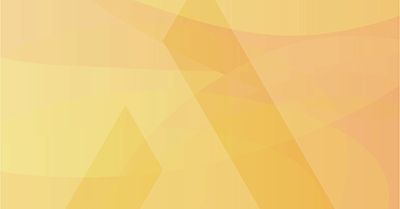
Jul 27
0
min
What is Data Governance - a Complete Guide
ALTR Blog
Most of us know that data creation and collection has accelerated over the last few years. Along with that has come an increase in data privacy regulations and the prominence of the idea of “data governance” as something companies should be focused on and concerned with. Let’s see what’s driving the focus on data governance, define what “data governance” actually is, look at some of the challenges, and how companies can implement data governance best practices to build a modern enterprise data governance strategy.
Data Governance History
The financial services industry was one of the first to face regulations around data privacy. The Gramm–Leach–Bliley Act (GLBA) of 1996 requires all kinds of financial institutions to protect customer data and be transparent about data sharing of customer information. This was followed by the Payment Card Industry Data Security Standard (PCI DSS) in 2006. Then the Financial Industry Regulatory Authority (FINRA), founded in 2007, established rules institutions must follow to protect customer data from breach or theft.
Perhaps not surprisingly, healthcare was another industry to face early data regulations. The first sensitive data to be covered in the US was private health data – the Health Insurance Portability and Accountability Act of 1996 (HIPAA) required national standards to protect sensitive patient health information from being disclosed without the patient’s consent or knowledge. More recently, data privacy regulations like the European Union’s GDPR and California’s CCPA privacy regulation have expanded coverage to all variety of “personal data” or Personal Identifiable Information (PII). These laws put specific rules around what companies can do with sensitive personal data, how it must be tracked and protected. And US data privacy guidelines have not stopped there – Colorado, Connecticut, Virginia and Utah have all followed their own state-level privacy regulations. So today, just about every company deals with some form of sensitive or regulated data. Hence the search for data governance solutions that can help companies comply.
What is Data Governance? - a Definition
Google searches for “data governance” have doubled over the last five years, but what is "data governance” really? There are a few different definitions depending on where you look:
- The Data Governance Institute defines data governance as “a system of decision rights and accountabilities for information-related processes, executed according to agreed-upon models which describe who can take what actions with what information, and when, under what circumstances, using what methods.”
- The Data Management Association (DAMA) International says it is “planning, oversight, and control over the management of data and the use of data and data-related sources.”
- According to the Gartner Glossary, it’s “the specification of decision rights and accountability framework to ensure the appropriate behavior in the valuation, creation, consumption, and control of data and analytics.”
You could probably find a hundred more data governance definitions, but these are pretty representative. Interestingly, it’s either called a “system” or a “framework,” – which are very process-oriented terms.
At a high level, “data governance” is about understanding and managing your data. Enterprise data governance projects are often led by data governance teams, security teams or even cross-functional data governance councils who map out a process and assign data stewards to be responsible for various data sets or types of data. They’re often focused on data quality and data flows – both internally and externally.
As you can see, data governance is not technology. Still, technologies can enable the enterprise data governance model at various stages. And due to increased regulatory pressures, more and more software companies offer “data governance” solutions. Unfortunately, many of these solutions are narrowly focused on the initial steps of the data governance strategy—data discovery, classification or lineage. However, data governance can’t just be about data discovery, cataloguing or metadata management. While many regulations start with the requirement that companies “know” their data, they’ll never be fully in compliance if organizations stop there. In addition, fines and fees are associated with allowing data to be misused or exfiltrated, and the only way to avoid those is by ensuring data is used securely.
Data Governance Challenges
Companies can run into many data governance challenges – from knowing what data they have to where data is to understanding where the data comes from and if they can trust it or not. You can solve many of these challenges with the various data catalog solutions mentioned above. These data catalogs do a great job at helping companies discover, classify, organize and present a variety of data in a way that makes it understandable to data professionals and potential data users. You can think of the result as a data “card catalog” that provides a lot of context about the data but does not provide the data itself. Some catalog solutions even offer a shopping cart feature that makes it very easy for users to select the data they want to use.
That leads to the following data governance challenge: controlling access to data to ensure that only the people who should have access to specific data have access to that data.
This goes beyond the scope of most data catalog solutions – it’s like having a shopping cart with no ability to check out and receive your item. Managing these requests is often done manually via SQL or other database code. It can become a time-consuming and error-prone process for DBAs, data architects and data engineers as requests for access to data pile up. This happens very quickly once the data catalog is available – as soon as users within the organization can easily see what data is available, the next step is undoubtedly wanting access to it. In no time, those tasked with making data available to the company spend more time managing users and maintaining policies than they do developing new data projects.
Data Governance Benefits
While data governance can be a challenging task, there would not be so much focus on it if the benefits didn’t outweigh the effort. With a thoughtful and effective data governance strategy, enterprises can achieve these benefits:
1. Avoid hefty fines and stringent sanctions on leaked PII
As mentioned above, every company that deals with PII is subject to regulations regarding data handling. In the US, the regulatory landscape is still patchy but targeting the most stringent requirements is the easiest path. A robust data governance practice can ensure companies meet their obligations and avoid fines across all their spheres of operation.
2. Leverage data-driven decisions for competitive advantage
A key reason there are growing regulations around collecting and using personal and sensitive data is that companies would like to use this data to understand their customers better gain insight into optimization opportunities, and increase their competitive advantages.
In a Splunk survey of data-focused IT and business managers, 60 percent said both the value and amount of data collected by their organizations will continue to increase. Most respondents also rate the data they’re collecting as extremely or very valuable to their organization’s overall success and innovation. In a recent Snowflake survey with the Economist, 87% say that data is the most important competitive differentiator in the business landscape today, and 86% agree that the winners in their industry will be those organizations that can use data to create innovative products and services. A data governance strategy gives companies insight into what data is available to gather insight from, ensures the data is reliable and sets a standard and a practice for maintaining that data in the future, allowing the value of the data to grow.
3. Improve customer trust and relationships
In a 2019 Pew Research Center study, 81% of Americans said that the potential risks they face because of data collection by companies outweigh the benefits. This might be because 72% say they personally benefit very little or not at all from the data companies gather about them. However, a recent McKinsey survey showed that consumers are more likely to trust companies that only ask for information relevant to the transaction and react quickly to hacks and breaches or actively disclose incidents. Coincidentally, these are some of the requirements of data privacy regulations – only gather the information you need and be upfront, timely and transparent about leaks.
What is data governance in healthcare?
Data governance in healthcare is very focused on complying with federal regulations around keeping personal health information (PHI) private. The US Health Insurance Portability and Accountability Act of 1996 (HIPAA) modernized the flow of healthcare information. It stipulates how personally identifiable information maintained by the healthcare and healthcare insurance industries should be protected from fraud and theft, and addressed some limitations on healthcare insurance coverage. It generally prohibits healthcare providers and healthcare businesses, called covered entities, from disclosing protected information to anyone other than a patient and the patient's authorized representatives without their consent. With limited exceptions, it does not restrict patients from receiving information about themselves. It does not prohibit patients from voluntarily sharing their health information however they choose, nor does it require confidentiality where a patient discloses medical information to family members, friends, or other individuals not a part of a covered entity. Any entity that has access to or holds personal health information on an individual is required to comply with HIPAA.

Data Governance Best Practices
Today, organizations utilize massive amounts of data across the enterprise to keep up with the pace of innovation and stay ahead of the competition. But making data available to users throughout the business also increases the risk of loss and the potential costs of a breach. It seems like an impossible choice: use data or protect it. But unfortunately, it’s not a choice; organizations must protect data before sharing it.
This requires a solution that includes these enterprise data governance best practices:
- Data discovery, classification and lineage – to ensure regulated data governance, companies must be able to identify, locate and trust it.
- Automated data access controls – as the need for data across the business grows, manual granting of access requests becomes infeasible. Manual controls slow down access to data and introduce the possibility of human error, potentially creating compliance issues instead of avoiding them. Role-based access controls are more efficient in ensuring that only authorized users get access to the data they need.
- Data usage visibility and tracking – once data has been logged and access granted, there must be visibility into who is using what data, when and how much. This helps companies prepare for an audit while ensuring appropriate data usage. It can also provide valuable insight into normal usage patterns to identify out-of-normal areas for concern more easily
- Automated policy enforcement - after data access has been granted, there must still be the ability to automatically alert, slow or stop any out-of-policy activity to prevent or halt credentialed access threats.
In addition, a solution must make the implementation of data governance easy for groups across the company. It’s not just data, security or governance teams responsible for keeping data safe – it’s everyone’s job.

Data Governance: the Future
There’s zero chance that data collection, use and regulation will decrease in the coming years. IDC predicts that the global datasphere will double in size from 2022 to 2026. Regulations also show no sign of slowing – a US federal privacy bill was making its way through approvals as of July 2022.
Both of these trends mean that if companies don’t have a data governance strategy in place now, they will soon need to. As a result, the number of data governance solutions will continue to increase rapidly. Some of these will come from legacy players seemingly offering soup to nuts; some from energetic new startups providing a fix for a single task with very little expertise. We expect the industry to move toward an enterprise data governance solution that helps companies meet global privacy requirements while being easy to use, manageable and scalable to keep up with growing data and regulations.

Aug 3
0
min
Data Catalogs and Data Governance: 4 Steps to Control and Protect Sensitive Data
ALTR Blog
A data catalog is a tool that puts metadata at your fingertips. Remember libraries? The card catalog puts all the information about a book in a physical or virtual index, such as its author, location, category, size (in pages), and the date published. You can find a similar search tool or index in an online music or video service. The catalog gives you all the essentials about the thing or data, but it is not the data itself. Some catalogs do not provide any measure of protection other than passive alerts and logs. Even basic access controls and data masking can shift the burden to data owners and operators. Coding access controls in a database puts more stress on the DBAs. Solutions requiring copying sensitive data into a proprietary database still expose the original data. These steps also don’t stop credentialed access threats: system admins can still access sensitive customer data. They can accidentally delete the asset. If credentials get lost or stolen, anyone can steal the data or cause other harm to your business. Data classifiers and catalogs are valuable, no doubt about it. But they’re not governance. They can’t fulfill requests for access, track, or constrain them. When it comes to data catalogs and data governance, you must address a broad spectrum of access and security issues, including:
Access:
You can’t give everyone the skeleton key to your valuable data; you must limit access to sensitive data for specific users.
Compliance:
If you cannot track individual data consumption, it will be nearly impossible to maintain an audit trail and share it for compliance.
Automation:
How do you ensure that the policies you set up are implemented correctly? Do you have to hand them off to another team to execute? Or do you have to write and maintain the code-based controls yourself?
Scale:
As data grows in volume and value, you’ll see more demand from users to access it. You must also ensure the governance doesn’t impede efficiency, performance, or the user experience. Controlling access can’t grind everything to a halt.
Protection:
Sensitive data must be secure; it’s the law virtually everywhere. Governance must ensure confidential data receives the maximum security available wherever it is. Companies need visibility into who consumes the data, when, and how much. They must see both baseline activity and out-of-the-norm spikes. And they must take the next crucial step into holistic data security that limits the potential damage of credentialed access threats.
Data Catalogs and Data Governance: 4 Steps to Control and Protect Sensitive Data
When it’s all said and done, data governance must be easy to implement and scale for companies as part of their responsibility to collect, store, and protect sensitive data. Bridging the gap in security and access can help you comply with applicable regulations worldwide while ensuring protection for the most valuable assets. When it comes to data catalogs and data governance you can follow these four steps to control access and deliver protection over sensitive data:
1. Integrate your data governance tools with an automated policy enforcement engine with patented security.
The data governance solution should provide security that can be hands-free, require no code to implement, and focus on the original data (not a copy) to ensure only the people who should have access do. This means consumption limits and thresholds where abnormal usage triggers an alert to halt access in real-time. Tokenizing the most critical and valuable data prevents theft and misuse. These controls help admins stop insider threats and allow continued access to sensitive data without risking it.

2. Set your policies once and automate implementation to reduce manual errors and risk.
You can eliminate tedious and manual configuration of access policies to save time and ensure consistent enforcement. Automation lets you control access by user role or database row and audit every instance. These policies restrict access and limit what users can see and analyze within the database. The ability to track and report reporting on every model of access makes it easy to comply with regulatory requests.
3. Enable self-service data requests to speed up data access.
Automated access controls let admins provide continued access to sensitive data, apply masking policies, and stop credentialed access threats for thousands of end users without putting the data at risk. Data teams can move at speed required by the business yet be restricted to accessing only the data sets they’re authorized to view. For instance, you can prevent an employee based in France from seeing local data meant only for Germans. You can also avoid commingling data that originated from multiple sources or regions. This allows you to foster collaboration and sharing with greater confidence in security and privacy measures.
4. Scale your data access control and policy enforcement as the use and uses of data grow throughout your business.
The scope of data access requests today within enterprises has reached a level that requires advanced automation. Some enterprises may have scanned and catalogued thousands of databases, even more. Data governance solutions should quickly implement and manage access for thousands of users to match. Features like rate-limiting stipulate the length or amount of access, such as seeing a small sample for a brief period for anyone who isn’t the intended consumer, like the catalog admin—scaling policy thresholds as needed allows you to optimize collaboration while stopping data theft or accidental exposure. You can limit access regardless of the user group size or data set.
Modern and Simple Data Governance
Modern data organizations are moving to simplify data governance by bringing visibility to their data and seeking to understand what they have. However, data governance doesn’t stop once you catalog your data. That’s like indexing a vast collection of books or songs but letting no one read or listen to the greatest hits. You should grant access to sensitive data but do so efficiently to not interfere with your day job and effectively comply with regulations and policy. Integrating a data catalog with an automated policy enforcement engine is the right strategy. You’ll gain the complete package, with a governance policy that is easy to implement and enforce, access controls that focus on the original sensitive data, and detailed records of every data request and usage. Managing enterprise data governance at scale lets, you use data securely to add value faster, turning the proverbial oil into jet fuel for your organization’s growth.

Jun 24
0
min
Clearing Up the Clouded Data Governance Solution Space
ALTR Blog
If we learned anything at Snowflake Summit (and we did – a lot!) it’s that the data governance space is as confusing as it is frenzied. Nearly every company is at some stage of moving to capitalize on cloud data analytics, while the regulatory environment around data continues to increase the urgency for privacy and security. Every single data governance, control, security session we saw was completely packed, indicating that many companies are now ready to focus on protecting sensitive data. Yet, some of the options in the market are misnamed, confusing and frustrating for buyers. There are many focused providers and even other adjacent software markets like data catalogs are starting to offer basic features. Also, Snowflake itself continues to roll out very powerful, albeit very manual-to-implement, governance features.
We’re hoping to not only clear up some of the FUD around data governance solutions, but also set the bar for how easy, functional and cost-effective data governance can and should be.
A new paradigm for controlling data access and security at scale
Last week we announced our new policy automation engine which combines governance features like access control and dynamic data masking with security controls like data usage limiting and tokenization, leveraging metadata like Snowflake Object Tagging and implemented and managed without code. With this new data governance solution, we’ve maintained our commitment to cloud-native delivery that supports best-in-category time to value and zero cost of ownership beyond our very reasonable, by user by month subscription.
For ALTR this is the realization of our vision for data privacy and security driven by people who can best accomplish it – the people who know the data – by assembling disparate tools into a single engine and single POV across the enterprise.
Built on a flexible, secure cloud foundation that leverages and automates Snowflake’s own features
ALTR is a true cloud-native SaaS offering that can be added to Snowflake using Partner Connect or a Snowflake Native App in just minutes. It integrates seamlessly with Snowflake without the need to install and maintain a proxy or other agent. Our microservices infrastructure takes full advantage of the scalability and resilience of the cloud, offering extremely high availability with multi-region support by default, because your data governance solution simply cannot go down.
Importantly, our service is built the same way as Snowflake itself and leverages Snowflake’s native features whenever possible. Those powerful features all involve writing SnowSQL to implement, and we automate them so that you don’t have to scale them yourself and you can go completely no-code.
We have also been Soc 2 Type 2 and PCI DSS Level 1 certified for years, and we maintain a highly disciplined security culture in our technical teams. Across various data sources we offer multiple integration types, including Cloud-to-Cloud Integration, Smart Database Drivers, and Proxy solutions. They all connect and use the same ALTR cloud service.
A data governance solution to both scale and safeguard
All of this comes together in an easy-to-use solution that delivers combined data governance and security for thousands of users across on premises and in cloud data storage. Automation of access policy can unlock months of person-hours per year in writing and maintaining policy, in the same way that ETL/ELT providers automating data pipelines saved data teams huge amounts of time in provisioning data.
In addition, unlike all the other providers in the data governance space, the ALTR solution moves beyond traditional data access policy tools like RBAC and dynamic masking into data security functionality like data usage limits and tokenization. We feel that your policy around data should contemplate your credentialed users and also extend to use cases where credentials might have been compromised or privileged access is an issue. For us, data policy is about both control and protection, and those policies should be developed and enforced with both in mind.
The future is bright – for data governance solution buyers
We’ll continue to extend our solution by deepening our policy engine’s capabilities with new policy types, expanding our support for a greater variety of data sources and data integrations, and building out more seamless integrations with like-minded players in the data ecosystem (such as more ELT and Catalog providers). All of this is driven and directed by a growing community of customers who are innovating in data and showing us where they need us the most. As the technology space moves forward, the options available to those still searching for a data governance solution will come into focus and the best choice will be clear.
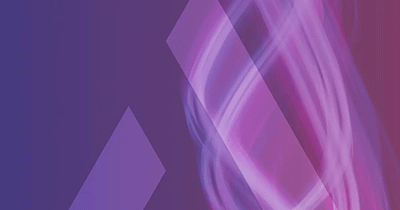
Jul 6
0
min
Data Modernization, Regulation, Democratization and the CDO
ALTR Blog
It was amazing to soak up the data ecosystem at Snowflake Summit a few weeks ago, but until we meet again, one way to stay up to date on all the last industry topics is by watching what our partners are talking about...virtually. Below are some of the most interesting recent blog posts…
Matillion + Snowflake: Increase Your Speed to Insight with Data Modernization
Today’s insight is tomorrow’s old news so getting there quickly is critical. This blog post shares how data modernization preparation through automation allows enterprises to spend less time getting data ready for the ETL process and more time finding those nuggets of knowledge. Snowflake and Matillion get users there faster. (Snowflake also clearly sees the power of the combination...)
BigID: Utah Pushes Privacy Legislation to the Forefront
Another state, another privacy act. While a new federal privacy regulation is making the rounds, the Utah legislature unanimously passed the Utah Consumer Privacy Act (S.B. 227) on March 3, 2022 — one day before it adjourned for its 64th session. The Governor who will have twenty days to decide whether to sign, not sign, or veto the bill. If signed, this would become the fourth state privacy act in the United States following California, Virginia, and Colorado. BigID explains how they can help customers comply with the new regulation – and all state, federal and international privacy laws.
Alation: How Fifth Third Bank Democratizes Data Access via a Data Mesh with Alation and Snowflake
Despite being one of the largest and well known US consumer financial services institutions, Fifth Third Bank did not have the proper structure to support their data growth needs. Alation stepped in to help the bank democratize data usage by creating a more self-serve structure ¾ delivering the right people the right data to do their jobs. We heard a lot about the idea of “data mesh” at Snowflake Summit so it’s interesting to see it in the wild.
Snowflake: A CDO’s Field Guide to Finding Value in Data
The rise of the Chief Data Officer (CDO) was one of our top 2022 predictions, and it looks like Snowflake is seeing it come true. CDOs are taking the lead on modernization, creating the data value chain and delivering data-driven insights that require collaboration across the organization. This blog post explains how the other “D” in “CDO” is “diplomacy” and provides some tips for demonstrating the value of data and its impact across the business.
After Summit, we’re curious to see the next hot industry topics! Don’t worry, we’ll keep you posted.

Jul 26
0
min
ALTR's View from Snowflake Summit 2023
ALTR Blog
Q2 was a busy and inspiring quarter for ALTR. Between the excitement of day-to-day operations, our bi-annual GTM kickoff at our Florida office, and multiple events attended, we did not fall short of excitement the past three months.
Notably, many members of our team enjoyed a full week in Las Vegas, NV at Snowflake Summit 2023. We had the great honor of sharing a speaking session with Matillion, co-hosting two after-hours events with Passerelle and with Matillion, and enjoying a week of learning as much as we could from our partners, customers, and friends in the data ecosystem. We’ve compiled the big announcements our partners made this year and some of our highlights of the event below.
Snowflake - Industry Recap
Snowflake shared a Snowflake Summit recap blog post, in which they dive into the data landscape and data forecast across multiple business sectors: Financial Services, Retail & Consumer Packaged Goods, Healthcare & Life Sciences, and Manufacturing, Telecom, and Advertising, Media, and Entertainment.
They write, “As we continue to revolutionize the way businesses operate, allowing [data users] to solve their most pressing problems and drive revenue through the Data Cloud, the insights, expertise, and experiences we offer at Summit have continued to grow. And this year was no different! Powered by our latest product offerings and the ways in which we’re enabling companies to leverage AI as a competitive advantage, this year’s event was electrifying, with tons of fantastic product demos, customer sessions, keynotes, and more. […] This recap offers the high points of how the Data Cloud is reshaping the data landscape for a large number of industries.”
Exciting News in the Data Ecosystem
Matillion Announces the End of Slow, Fragmented, Expensive Data Pipelines
Matillion launched a new productivity platform for data teams this year at Snowflake Summit. This platform is built with the intention to empower the full data team to move, transform, and orchestrate data pipelines as day-to-day business users — regardless of technical knowledge.
“Matillion makes data work more productive by empowering the entire data team – coders and non-coders alike – to move, transform, and orchestrate data pipelines faster. Its Data Productivity Cloud empowers the whole team to deliver quality data at a speed and scale that matches the business’s data ambitions.”
Read More About the Data Productivity Cloud here
Alation Launches Connected Sheets in Snowflake
Alation announced a new way to access data from Excel and Sheets directly in Snowflake using their new product offering: Alation Connected Sheets. This offering from Alation is another step in the right direction of streamlining data productivity using the platforms business users use most frequently.
“With Alation Connected Sheets for Snowflake, business users can now instantly find, understand, and trust the data they need – without leaving their spreadsheet. This product enables business users to access Snowflake source data directly from the spreadsheets in which they work without the need to understand SQL or rely on central data teams. Alation Connected Sheets also enable data governance teams to set access policies on which users can access various data objects.”
Tacos, Margaritas, and Good Data
Passerelle’s Data Oasis
One highlight of Snowflake Summit 2023 were the two after-hours events ALTR had the honor of co-hosting. Alongside Passerelle, Talend, SqlDBM, Equifax and GrowthLoop, we enjoyed a fajita bar, custom ALTR-ita’s, and fantastic conversations with our partners in the data ecosystem. Nearly every stage in the data lifecycle was represented at this event, allowing attendees a full scope of data productivity and data security.
Carolyn Fernald, the Marketing and Event Coordinator behind Data Oasis, wrote, “We hosted a really fun event with Talend, SqlDBM, ALTR, Equifax, and GrowthLoop (Flywheel) — the room was abuzz while folks completed a scavenger hunt and learned about new offerings from the partners.”
Thanks for hosting a fantastic event, Passerelle!
Matillion’s Fiesta in the Clouds
The other after-hours event ALTR co-hosted was Fiesta in the Clouds alongside Matillion, Amazon Web Services, Dataiku, Deloitte, and ThoughtSpot. This event took over both floors of the Chayo Restaurant on the LINQ Promenade in Vegas, offering attendees the opportunity to connect over good data, good tacos, and great margaritas. Each of the co-hosts of this event showed up offering a great time to be had by all hosts and attendees!
Kathy O’Neil, Director, Customer and Partner Programs at Matillion, wrote, “HUGE THANK YOU to the 900+ people that joined Matillion for Tuesday night’s Fiesta in the Clouds at Snowflake Summit and to our sponsors, Amazon Web Services (AWS), ALTR, Dataiku, Deloitte, ThoughtSpot - it was an incredible evening!”
We are already counting down the days to Snowflake Summit 2024 in San Francisco where ALTR will be a Blue Square sponsor!
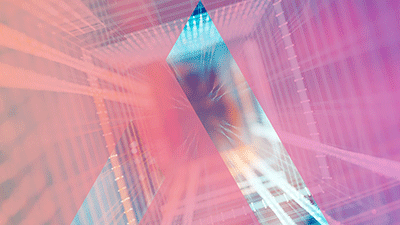
Jul 13
0
min
4 Steps to a Successful BI Governance Strategy
ALTR Blog
The famous motorcycle stunt rider Evel Knievel, who holds the world record for the most broken bones in his lifetime, once said, “I did everything by the seat of my pants. That's why I got hurt so much.” He was talking about daring feats, like jumping his red-white-and-blue motorcycle across the Snake River Canyon. But he could have been talking about business intelligence (BI) and data security.
Performing daredevil feats takes careful planning and a team. Knievel did everything when he started, from setting up the jumps to writing his promotional press releases. But it was a painful process and took time and energy away from his primary focus, performing. So he recruited a team who could handle myriad tasks while he focused on executing the stunt.
The same goes for unlocking business intelligence to have a complete 360° view of your organization. You may have been initially successful with data analysis using BI and data platforms themselves, like Tableau and Snowflake. That’s fine for business data, but what about regulated data? Not including sensitive information like personally identifiable information (PII) leaves essential data on the table and obscures the view of your business operations.
Attempting BI projects in the cloud without a holistic approach for BI governance is like trying to jump a motorbike across a canyon all by yourself. You need a methodical process to seamlessly integrate BI, data governance, and the data warehouse.
Here are four steps to a successful analytics governance strategy:.
- Implement a data control and protection solution that integrates with your cloud data warehouse. The governance solution should employ contextual info provided by the BI tool to distinguish users from each other. Sending through information on the specific BI user making the request simplifies things. The database admin needs only to configure and manage a single, shared BI service account, yet gain per-user visibility and governance as though every data end-user had their own account.
- Set up the appropriate policies in the governance tool. You should be able to apply policies that restrict access at a granular level that includes by user role or by database row and then audit every instance. BI admins gain flexibility and controls to dial in security policies for sensitive data.
- Use governance tool / BI tool integration that allows you to a) split out the service account access by individual users and b) place thresholds/rate limit policies by individual users or roles. Operators can adjust the policy thresholds to optimize collaboration while preventing data theft or accidental exposure.
- Set up alerts to collaboration tools like email and Slack to give you a heads up when a user’s access is out of compliance. As part of the auditing trail, you want to know exactly when and who is trying to access sensitive data and if any patterns are abnormal.
BI and analytics governance creates accountability and enables access to secure and trusted sensitive content for users, so you don’t have to fly by the seat of your pants to deliver a complete view of your organization to business leaders.
Want to jump ahead? Consider ALTR, Tableau and Snowflake Together
Read our eBook to determine the best BI governance strategy for unlocking business value.
If you’ve already selected Tableau and Snowflake, keep in mind that ALTR has developed a unique solution that employs contextual info provided by Tableau to distinguish users and allow you to apply governance policies to the data in Snowflake:
- With a simple, one-time configuration of a SQL database variable in Tableau Server, the service account that Tableau uses to connect to Snowflake can send through information on which a Tableau user is making the request.
- ALTR can then apply governance and security policy on that user as it would on any individual Snowflake account.
ALTR is the only Snowflake provider delivering this high level of integration to solve a vast BI security headache.
The combination of Tableau, Snowflake, and the award-winning ALTR SaaS platform delivers more value from your data in minutes while helping you avoid the headache of managing thousands of user accounts.
See how it works

Jun 29
0
min
Modern Data Ecosystem: Navigating the New Reality
ALTR Blog
Today, most companies understand the significant benefits of the "Age of Data." Fortunately, an ecosystem of technologies has sprouted up to help them take advantage of these new opportunities. But for many companies, building a comprehensive modern data ecosystem to deliver data value from the available offerings can be very confusing and challenging. Ironically, some technologies that have made specific segments easier and faster have made data governance and protection appear more complex.
Big Data and the Modern Data Ecosystem
"Use data to make decisions? What a wild concept!" This thought was common in the 2000s. Unfortunately, IT groups didn't understand the value of data – they treated it like money in the bank. They thought data would gain value when stored in a database. So they prevented people from using it, especially in its granular form. But there is no compound interest on data that is locked up. The food in your freezer is a better analogy. It's in your best interest to use it. Otherwise, the food will go bad. Data is the same – you must use, update, and refresh it, or else it loses value.
Over the past several years, we've better understood how to maximize data's value. With this have come a modern data ecosystem with disruptive technologies enabling and speeding up the process, simplifying complicated tasks and reducing the cost and complexity required to complete tasks.
But looking at the entire modern data ecosystem, it isn't easy to make sense of it all. For example, suppose you try to organize companies into a technology stack. In that case, it's more like "52 card pickup" – no two cards will fall precisely on each other because very few companies present the same offering and very few cards line up side-to-side to offer perfectly complementary technologies. This is one of the biggest challenges of trying to integrate best-of-breed offerings. The integration is challenging, and interstitial spots are difficult to manage.
If we look at Matt Turck's data ecosystem diagrams from 2012 to 2020, we will see a noticeable trend of increasing complexity – both in the number of companies and categorization. It isn't evident even for those of us in the industry. While the organization is done well, pursuing a taxonomy of the analytics industry is not productive. Some technologies are mis-categorized or misrepresented, and some companies should be listed in multiple spots. Unsurprisingly, companies attempting to build their modern stack might be at a loss. No one knows or understands the entire data ecosystem because it's massive. These diagrams have value as a loosely organized catalog but should be taken with a grain of salt.
A Saner, but Still Legacy Approach to the Modern Data Ecosystem
Another way to look at the data ecosystem—one that's based more on the data lifecycle is the "unified data infrastructure architecture," developed by Andreessen Horowitz (a16z). The data ecosystem starts with data sources on the left, ingestion/transformation, storage, historical processing, and predictive processing and on the right is output. At the bottom are data quality, performance, and governance functions pervasive throughout the stack. This model is similar to the linear pipeline architectures of legacy systems.
Like the previous model, many of today's modern data companies don't fit neatly into a single section. Instead, most companies span two adjacent spaces; others will surround"storage," for example, having ETL and visualization capabilities, to give a discontinuous value proposition.

1) Data Sources
On the left side of the modern data ecosystem, data sources are obvious but worth discussing in detail. They are the transactional databases, applications, application data and other datasources mentioned in Big Data infographics and presentations over the past decade. The main takeaway is the three V's of Big Data: Volume, Velocity and Variety. Those Big Data factors had a meaningful impact on the Modern Data Ecosystem because traditional platforms could not handle all V's. Within a given enterprise, data sources are constantly evolving.
2) Ingestion and transformation
Ingestion and transformation are a bit more convoluted. You can break this down into traditional ETL or newer ELT platforms, programming languages for the promise of ultimate flexibility, and event and real-time data streaming. The ETL/ELT space has seen innovation driven by the need to handle semi-structured and JSON data without losing transformations. There are many solutions in this area today because of the variety of data and use cases. Solutions are capitalizing on the ease of use, efficiency, or flexibility, where I would argue you cannot get all three in a single tool. Because data sources are dynamic, ingestion and transformation technologies must follow suit.
3) Data Storage
Storage has recently been a center of innovation in the modern data ecosystem due to the need to meet capacity requirements. Traditionally, databases were designed with computing and storage tightly coupled. As a result, the entire system would have to come down if any upgrades were required, and managing capacity was difficult and expensive. Today innovations are quickly stemming from new cloud-based data warehouses like Snowflake, which has separated compute from storage to allow for improved elasticity and scalability. Snowflake is an interesting and challenging case to categorize. It is a data warehouse, but its Data Marketplace can also be a data source. Furthermore, Snowflake is becoming a transformation engine as ELT gains traction and Snowpark gains capabilities.While there are many solutions in the EDW, data lake, and data lakehouse industries, the critical disruptions are cheap infinite storage and elastic and flexible compute capabilities.
4) Business Intelligence and Data Science
The a16z model breaks down into the Historical, Predictive and Output categories. In my opinion, many software companies in this area occupy multiple categories, if not all three, making these groupings only academic. Challenged to develop abetter way to make sense of an incredibly dynamic industry, I gave up and over simplified. I reduced this to database clients and focused on just two types: Business Intelligence (BI) and Data Science. You can consider BI the historical category, Data Science the predictive category and pretend that each has built-in "Output." Both have created challenges to the data governance space with their ease of use and pervasiveness.
BI has also come along way in the past 15 years. Legacy BI platforms required extensive data modeling and semantic layers to harmonize how the data was viewed and overcome slower OLAP databases' performance issues. Since a few people centrally managed these old platforms, the data was easier to control. In addition, users only had access to aggregated data that was updated infrequently. As a result, the analyses provided in those days were far less sensitive than today. In the modern data ecosystem, BI brought a sea of change. The average office worker can create analyses and reports, the data is more granular (when was the last time you hit an OLAP cube?), and the information is approaching real-time. It is now commonplace for a data-savvy enterprise to get reports updated every 15 minutes. Today, teams across the enterprise can see their performance metrics on current data and enable fast changes in behavior and effectiveness.
While Data Science has been around for a long time, the idea of democratizing has started to gain traction over the past few years. I use the DS term in a general sense of statistical and mathematical methods focusing on complex prediction and classification beyond basic rules-based calculations. These new platforms increased the accessibility of analyzing data in more sophisticated ways without worrying about standing up the compute infrastructure or coding complexity. "Citizen data scientists" (using this term in the most general terms possible) are people who know their domain, have a foundational understanding of what data science algorithms can do, but lack the time, skill, or inclination to deal with the coding and the infrastructure. Unfortunately, this movement also increased the risk of exposure to sensitive data. For example, analysis of PII may be necessary to predict consumer churn, lifetime value or detailed customer segmentation. Still, I argue it doesn't have to be analyzed in raw or plain text form.
Data tokenization—which allows for modeling while keeping data secure—can reduce that risk. For example, users don't need to know who the people are and how to group them to execute cluster analysis without needing exposure to sensitive granular data. Furthermore, utilizing a deterministic tokenization technology, the tokens are predictable yet undecipherable to enable database joins if the sensitive fields are used as keys.
Call it digital transformation, the democratization of data or self-service analytics, the aesthetic for Historical, Predictive, Output – or BI and Data Science – is making the modern data ecosystem more approachable for the domain experts in the business. This also dramatically reduces the reliance on IT outside of the storage tier. However, the dynamics of what data can do requires users to iterate, and iterating is painful when multiple teams, processes, and technology get in the way.
Modern Data Ecosystem Today: a Lighter Lift leads to Other Issues
When looking back at where we came from before the Modern Data Ecosystem, it seems like a different world. Getting data and making sense of it was a heavy lift. Because it was so hard, there was an incentive to “do it once” driving large scale projects with intense requirements and specifications. Today the lift is significantly lighter – customers call it quick wins; vendors call it land and expand. Ease of use and User Experience have become table stakes where the business user is now empowered to make their own data-driven decisions.
But despite all the innovations by the enterprise software industry, there are some areas that have been left behind. Like a car that got an upgrade to its engine but still has its original steering and wheels, the partially increased performance created increased risk.
The production data & analytics pipeline that will remain unchanged for years is dead. Not only that, but there is also no universal data pipeline either. Flexible, easy to create data pipelines and analytics are key to business success.
All the progress over the past decade or so has left some issues in its wake:
A single, stable, and linear data pipeline is dead
I once heard from a friend of mine who is a doctor that said, “If there are many therapies for a single ailment, chances are none of the therapies are good”. I used to draw a parallel of that adage to data pipelines, but I realized that the analogy falls short. It falls short since there are many data pipeline problems that need to be solved with their own needs. Data pipelines can be provisional or production, speedy or slow, structured or unstructured. The problem space where data ingestion and pipelines live is massive to the point where having a purposeful choice is the best play. Taking the 52-card pickup reference from earlier – you need a good hand to play with and in this game, you can choose your own cards.
Lift and shift to the cloud can exacerbate as many problems as it solves
One of the methods of enterprise architecture modernization is obviously moving to the cloud. Taking server by server, workload by workload and moving it to the cloud aka “lift and shift” has seemed to be the fastest and most efficient way to get to the cloud. And I would agree that it does but can inhibit progress if done in a strict mechanical way. Looking back at the data ecosystem models, new analytics companies are solving problems in many ways. So, the lift and shift method has a weakness that can cause one of two problems. Taking the old on-prem application and porting it to the cloud prevents any infrastructural advantages the cloud has to offer (scale and elasticity is the most common one). It also inhibits any improvements from a functional architecture perspective. It could be performing calculations in the BI or Data Science layer where it could perform better or be more flexible elsewhere. The one we will focus on later is governance from an authorization or protection point of view.
Governance needs to move away from the application layer
I touched on this in the last paragraph but deserves to be called out on its own. Traditionally data governance was a last mile issue. Determining who had the correct privileges to see a report or an analysis was executed when it was minted. In my opinion this is a throwback from the printed report era because you were literally delivering the report to an in box or an admin which can have its own inherent governance. The exact processes don’t follow through, but the expectations did. This bore an era of creating software where rudimentary controls were deployed at the database, but the sophisticated controls were in the application. Fast forward back to the present day. Analytics and reporting are closer to a BYOD cell phone strategy than a monolithic single platform for reports. This creates an artificial zero-sum game where analytic choice is at odds with governance. The reality is companies need to be analytically nimble to stay competitive. Knowledge workers want to support their company to stay competitive and will do whatever they can to do so. The culmination of easy-to-use software that is impactful allows users to follow the path of least resistance to get their job done.
How the Modern Data Ecosystem Broke Traditional Governance
While pockets of the data ecosystem have been advancing quickly, data governance has fallen behind when it comes to true innovation. With traditional software, governance was easy because everything else was hard: the data changed slowly, the output was static. The teams executing all this technology were small, highly trained, and usually located in a single office. They had to be because the processes were hard and confusing, and the technology was very difficult to implement and use. The side effect was that data governance was easier to do because data moved slower, was in fewer places and fewer people had access. The modern data ecosystem eliminated each of these challenges one by one. Governance became the legacy process and technology that couldn’t keep up with the speed of modern data.
Does governance need to be hard or partially effective? I would argue not, if you apply it at the right part of the process: the data layer. Determining risk exposure is important. That is where the analytics metadata management platforms have done so well. The modern data ecosystem enabled the creation of a wealth of data, analytics, and content. Management from a governance perspective provides a view into where the high-risk content is. Now we need an easy-to-use platform to execute on a governance strategy.
CTA: Want to find out how you can protect sensitive data in your modern data stack? Get a demo!

Jul 20
0
min
Data Teams! Stop Wasting Time on These Tasks
ALTR Blog
The journey to derive value from data is long, requiring data infrastructure, analysts, scientists, and data consumption management processes, among other things. Even when data operations teams move down this path, growing pains occur as more people demand more data as soon as data operations teams make progress. As a result, problems may arise quickly or develop gradually over time. Some strategies can help with this issue. A data team must recognize that these time-wasting issues are real and have a plan to tackle them.
1. Data Access without Automation
When you create a data catalog or establish a procedure for users to locate and request data, administering access becomes difficult. In conventional data architectures, accessing sensitive data is frequently a complex procedure involving much manual work. For example, creating and updating user accounts for several services can be time-consuming.
Put another way, no plan for data governance survives contact with users. So even if you build your data infrastructure in a legacy data governance model, you will be busy granting access to it. For instance, one global firm I spoke with had developed a data pipeline to move customer information from one on-premise system to their cloud data warehouse. They provided self-service access, but the demand was so significant that they devoted the following three months to granting access to that system.
Solution:
- No-code approaches allow you to quickly access or block access to a data set within a cloud data warehouse, associate that policy with specific users, and apply various masking techniques within minutes.
- You can also see your users, their roles, and the sensitive data they're accessing.
- You can then identify areas where you can apply access policy and make it easy to create an audit trail for governance
- More mature organizations struggling with thousands of data users may already have a data catalog solution. Integrating a control and protection solution with the data catalog allows you to create the policies, manage them in the catalog, and automatically enforce them in connected databases.
2. Manual Data Migration
Once you have established your initial cloud data warehouse and data-consumption schema, you will want to import additional data sets. However, manual data-migration approaches may slow you down and restrict your ability to gain insight from multiple sources. You can gain efficiency by refining your migration approach and tools instead:
Solution:
- Implement an ETL SaaS platform to eliminate manual discovery and migration tasks. It simplifies connection to multiple data sources, collects data from various sites, converts source data into tabular formats to make it easier to perform analytics, and moves it to the cloud warehouse.
- Use a schema manipulation tool like dbt, which transforms data directly in the cloud data warehouse.
- Follow a three-zone pattern for migration—raw, staging, and production.
- Maintain existing access and masking policies even as you add or move data or change the schema in the cloud data platform. For example, every time an email address moves around and gets copied by an automated piece of software, you must apply masking policies. In addition, you'll have to create an auditable trail every time you move data for governance.
3. Complicated Governance Auditing
With more people accessing data, you should set up a data governance framework to guarantee all that data's protection, compliance, and privacy. When data teams strive to identify the location of data, they frequently look at query or access logs and build charts and graphs to determine who has accessed it. When a big data footprint has many users interacting with it, you should not waste time applying for role-based access or creating reports manually.
Solution: To scale auditing, you should simplify it. Doing so will allow you to:
- Visualize and track access to sensitive data across your organization. Have an alerting system to let you know who, where, and how your data is accessed.
- Keep access and masking policies in lockstep with changing schema.
- Understand if data access is normal or out of normal thresholds.
- Create and automate thresholds that block access or allow access with alerts based on rules you can apply quickly.
- Automate classification and reporting to show granular relationships, such as the same user role accessing different data columns.
Should this even be your job?
The most significant time sink is data engineers, and DBAs handle data control and protection. Is this even logical? Since you're taking data from one place to the next and knowhow to write SQL code required by most tools to grant and restrict access, it has fallen to data teams.
But is that the best use of your time and talents? Wouldn't it make more sense for the teams whose jobs focus on data governance and security to be able to manage data governance and security? With the proper no-code control and protection solution, you could transfer these tasks to other teams – invite them to implement the policies, pick which data to mask, download audit trails, and set up alerts.Then, once you get all that off your plate, you can move on to what you were trained to do: extract value from data.
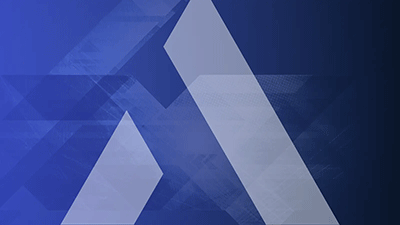
Jun 14
0
min
Data Governance for Financial Services
ALTR Blog
Financial Services was one of the first industries to recognize data's significant potential to understand customers better, improve services and streamline operations. However, the financial services industry faces unique data challenges despite data’s apparent benefits. Financial information is highly sensitive; as such, data governance in financial services is driven by strict regulations to protect it. This has led to conflicting forces: the urge to hold sensitive data in highly secure, on-premises databases owned and managed by the organization’s IT and security teams versus the drive to move critical data to a centralized cloud.
Until now, misconceptions around compromised data protection and privacy and the ability to achieve regulatory compliance have deterred many financial institutions from cloud adoption. However, with the cloud’s undeniable cost, scalability and flexibility benefits, the question for financial institutions is not whether to migrate to the cloud or not but how to transition to the cloud securely.
Virtual Fireside Chat: Secure Cloud Migration for Financial Institutions - Best practices for navigating cloud migration challenges Financial Institutions face with TDECU, Matillion, Wavicle and Snowflake
ALTR and Snowflake make data governance in financial services quick and easy. We help Financial Institutions comply with the strictest financial regulations while also making data available across the business. We also enable firms of all sizes to follow the Cloud Data Management Capabilities (CDMC) framework for protecting sensitive data in a cloud/hybrid cloud environment. ALTR, which seamlessly integrates with Snowflake, automates the configuration and enforcement of access, data masking, usage limits, and tokenization policy so financial institutions can spend less time worrying about data and more time using data.
1. Personalize Customer Experiences with a 360 View
Serving financial customers is about customizing their experience, leveraging a complete view of the customer on a single platform to power analysts, data scientists and applications. Inevitably PII and PFI will be used to provide services and products that lead to customer growth. ALTR helps you automate and scale access to and protection of your customers’ data without having to write, test, and maintain intricate policies.
- By extending ALTR’s security and governance layer to the newly migrated Snowflake Data Cloud, financial institutions can feel comfortable with analysts and data scientists accessing data in Snowflake.
- Without ALTR, financial institutions would have to manually replicate the highest levels of protection using Snowflake’s code-based governance and security features. ALTR automates these tasks away and streamlines data protection wherever it needs to go within the organization.
Case Study: Security Becomes a Business Enabler at this Leading Mortgage Firm - An enterprise-wide data governance and security solution from ALTR lets data users focus on delivering value
2. Automate Enterprise-wide Data Governance
Moving to the Data Cloud can help financial institutions meet regulatory requirements while sharing live access to data through a single platform. Snowflake Data Cloud is with ALTR as a PCI DSS Level 1 Service provider that is Soc 2 Type 2 certified and highly available across multiple regions; you can automate away policy enforcement headaches and make enterprise-wide data governance in financial services once a just a goal, a reality.
- Multiple integration methods allow ALTR to protect customer data from creation to on-premises and cloud data warehouses to multiple banking applications – from start to finish; ALTR covers the data.
- A single pane of glass across all the touch points that store or process sensitive data means there is always a single view of truth about data access and security.
- SaaS-based delivery means data can flow in and out of the company walls, from the data center to Snowflake, without any hiccup in service or protection.
Case Study: TDECU Takes a Data-Driven Approach to Supporting Its Members’ Financial Journeys - Seeking to increase data reliability and accelerate data-driven decision-making, TDECU began its cloud migration with Snowflake. It turned to ALTR to protect member data and ensure regulatory compliance.
3. Monitor Security Controls in Real-Time
Financial institutions can monitor compliance against enterprise security controls like NIST, ISO, CIS or others, using Snowflake with ALTR. Security teams can send ALTR’s rich data usage and event information back into Snowflake to provide the backbone for security analytics and auditing.
“Through their native cloud integration with Snowflake’s platform, ALTR’s approach to providing visibility into data activity in Snowflake’s Data Cloud provides a solution for customers who need to defend against security threats,” said Omer Singer Head of Cybersecurity Strategy, Snowflake.
- A leading mortgage firm loads all alerts and signals to Snowflake Security Data Lake – regardless of source – enabling the security team to bring all enterprise data stores under surveillance.
- According to the firm’s CISO, “With ALTR’s integrations and scalability, I'm not just solving this problem on Snowflake. We're starting with and building on Snowflake, but we’re embracing a solution that can solve this problem across the enterprise for us.”
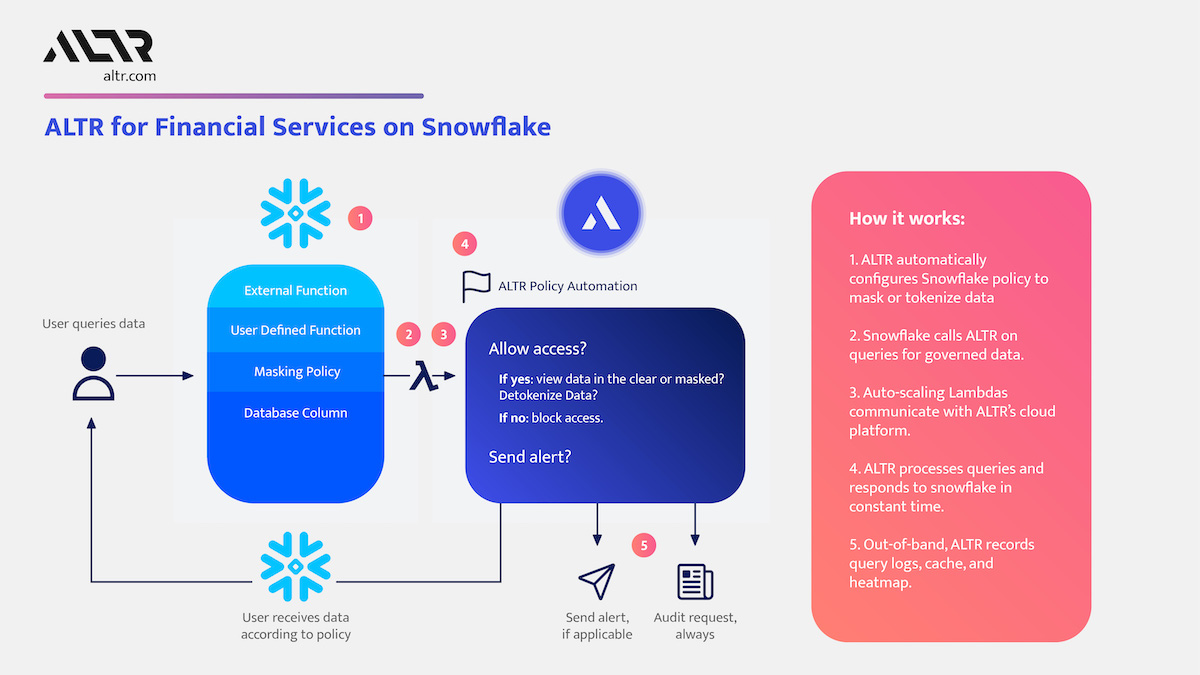
Blog: Moving to the Cloud Doesn't Have to Be Daunting for Small and Mid-size Financial Institutions - Financial Institutions can easily and safely move your enterprise data warehouse to Snowflake + ALTR
Wrapping Up
For any industry, migrating to the cloud can seem daunting. This is especially true in financial services, where extensive regulations have created the illusion that cloud deployments are challenging and risky. ALTR helps remove this risk with automated enterprise-wide data governance that allows financial institutions of all sizes to control and protect regulated financial data in the cloud as securely as in the data center.
See how ALTR can help improve your financial services data governance .
Get the latest from ALTR
Subscribe below to stay up to date with our team, upcoming events, new feature releases, and more.
Thank you! Your submission has been received!
Oops! Something went wrong while submitting the form.