BLOG SPOTLIGHT
ALTR’s Format-Preserving Encryption, powered by FF3-1 algorithm and ALTR’s trusted policies, offers a comprehensive solution for securing sensitive data.
Read more
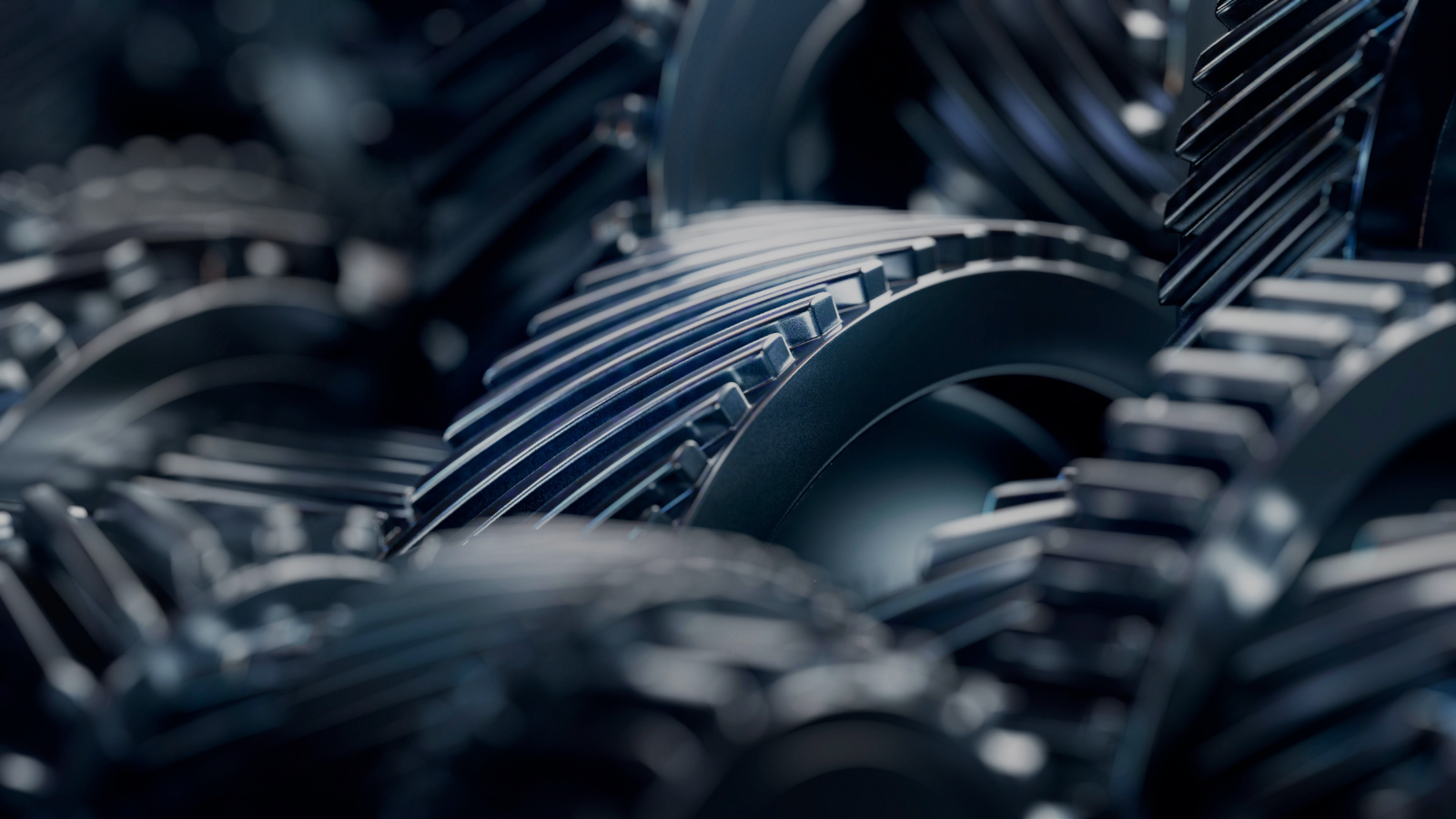
Popular
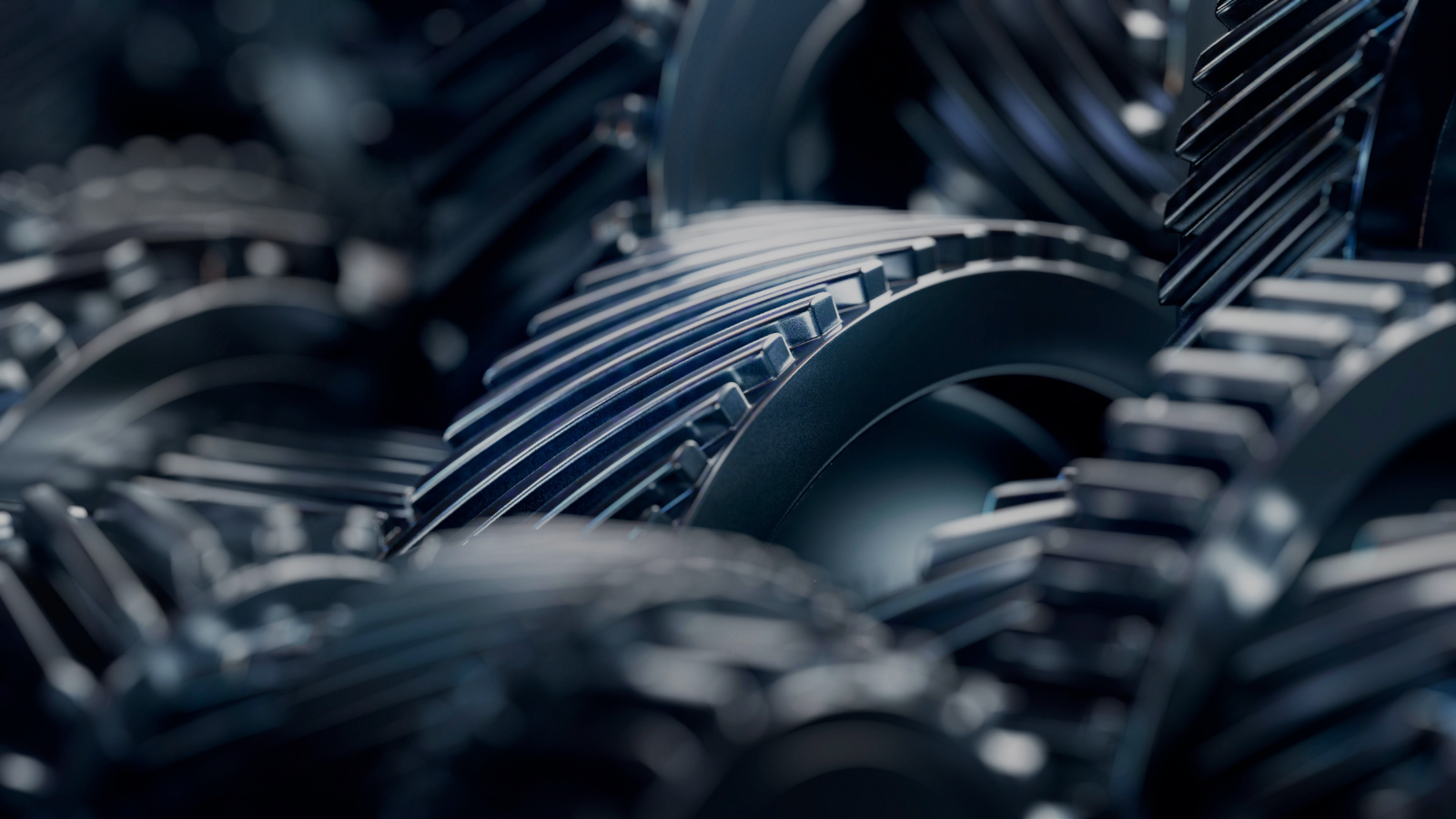
Oct 30
0
min
Format-Preserving Encryption: A Deep Dive into FF3-1 Encryption Algorithm
In the ever-evolving landscape of data security, protecting sensitive information while maintaining its usability is crucial. ALTR’s Format Preserving Encryption (FPE) is an industry disrupting solution designed to address this need. FPE ensures that encrypted data retains the same format as the original plaintext, which is vital for maintaining compatibility with existing systems and applications. This post explores ALTR's FPE, the technical details of the FF3-1 encryption algorithm, and the benefits and challenges associated with using padding in FPE.
What is Format Preserving Encryption?
Format Preserving Encryption is a cryptographic technique that encrypts data while preserving its original format. This means that if the plaintext data is a 16-digit credit card number, the ciphertext will also be a 16-digit number. This property is essential for systems where data format consistency is critical, such as databases, legacy applications, and regulatory compliance scenarios.
Technical Overview of the FF3-1 Encryption Algorithm
The FF3-1 encryption algorithm is a format-preserving encryption method that follows the guidelines established by the National Institute of Standards and Technology (NIST). It is part of the NIST Special Publication 800-38G and is a variant of the Feistel network, which is widely used in various cryptographic applications. Here’s a technical breakdown of how FF3-1 works:
Structure of FF3-1
1. Feistel Network: FF3-1 is based on a Feistel network, a symmetric structure used in many block cipher designs. A Feistel network divides the plaintext into two halves and processes them through multiple rounds of encryption, using a subkey derived from the main key in each round.
2. Rounds: FF3-1 typically uses 8 rounds of encryption, where each round applies a round function to one half of the data and then combines it with the other half using an XOR operation. This process is repeated, alternating between the halves.
3. Key Scheduling: FF3-1 uses a key scheduling algorithm to generate a series of subkeys from the main encryption key. These subkeys are used in each round of the Feistel network to ensure security.
4. Tweakable Block Cipher: FF3-1 includes a tweakable block cipher mechanism, where a tweak (an additional input parameter) is used along with the key to add an extra layer of security. This makes it resistant to certain types of cryptographic attacks.
5. Format Preservation: The algorithm ensures that the ciphertext retains the same format as the plaintext. For example, if the input is a numeric string like a phone number, the output will also be a numeric string of the same length, also appearing like a phone number.
How FF3-1 Works
1. Initialization: The plaintext is divided into two halves, and an initial tweak is applied. The tweak is often derived from additional data, such as the position of the data within a larger dataset, to ensure uniqueness.
2. Round Function: In each round, the round function takes one half of the data and a subkey as inputs. The round function typically includes modular addition, bitwise operations, and table lookups to produce a pseudorandom output.
3. Combining Halves: The output of the round function is XORed with the other half of the data. The halves are then swapped, and the process repeats for the specified number of rounds.
4. Finalization: After the final round, the halves are recombined to form the final ciphertext, which maintains the same format as the original plaintext.
Benefits of Format Preserving Encryption
Implementing FPE provides numerous benefits to organizations:
1. Compatibility with Existing Systems: Since FPE maintains the original data format, it can be integrated into existing systems without requiring significant changes. This reduces the risk of errors and system disruptions.
2. Improved Performance: FPE algorithms like FF3-1 are designed to be efficient, ensuring minimal impact on system performance. This is crucial for applications where speed and responsiveness are critical.
3. Simplified Data Migration: FPE allows for the secure migration of data between systems while preserving its format, simplifying the process and ensuring compatibility and functionality.
4. Enhanced Data Security: By encrypting sensitive data, FPE protects it from unauthorized access, reducing the risk of data breaches and ensuring compliance with data protection regulations.
5. Creation of production-like data for lower trust environments: Using a product like ALTR’s FPE, data engineers can use the cipher-text of production data to create useful mock datasets for consumption by developers in lower-trust development and test environments.
Security Challenges and Benefits of Using Padding in FPE
Padding is a technique used in encryption to ensure that the plaintext data meets the required minimum length for the encryption algorithm. While padding is beneficial in maintaining data structure, it presents both advantages and challenges in the context of FPE:
Benefits of Padding
1. Consistency in Data Length: Padding ensures that the data conforms to the required minimum length, which is necessary for the encryption algorithm to function correctly.
2. Preservation of Data Format: Padding helps maintain the original data format, which is crucial for systems that rely on specific data structures.
3. Enhanced Security: By adding extra data, padding can make it more difficult for attackers to infer information about the original data from the ciphertext.
Security Challenges of Padding
1. Increased Complexity: The use of padding adds complexity to the encryption and decryption processes, which can increase the risk of implementation errors.
2. Potential Information Leakage: If not implemented correctly, padding schemes can potentially leak information about the original data, compromising security.
3. Handling of Padding in Decryption: Ensuring that the padding is correctly handled during decryption is crucial to avoid errors and data corruption.
Wrapping Up
ALTR's Format Preserving Encryption, powered by the technically robust FF3-1 algorithm and married with legendary ALTR policy, offers a comprehensive solution for encrypting sensitive data while maintaining its usability and format. This approach ensures compatibility with existing systems, enhances data security, and supports regulatory compliance. However, the use of padding in FPE, while beneficial in preserving data structure, introduces additional complexity and potential security challenges that must be carefully managed. By leveraging ALTR’s FPE, organizations can effectively protect their sensitive data without sacrificing functionality or performance.
For more information about ALTR’s Format Preserving Encryption and other data security solutions, visit the ALTR documentation
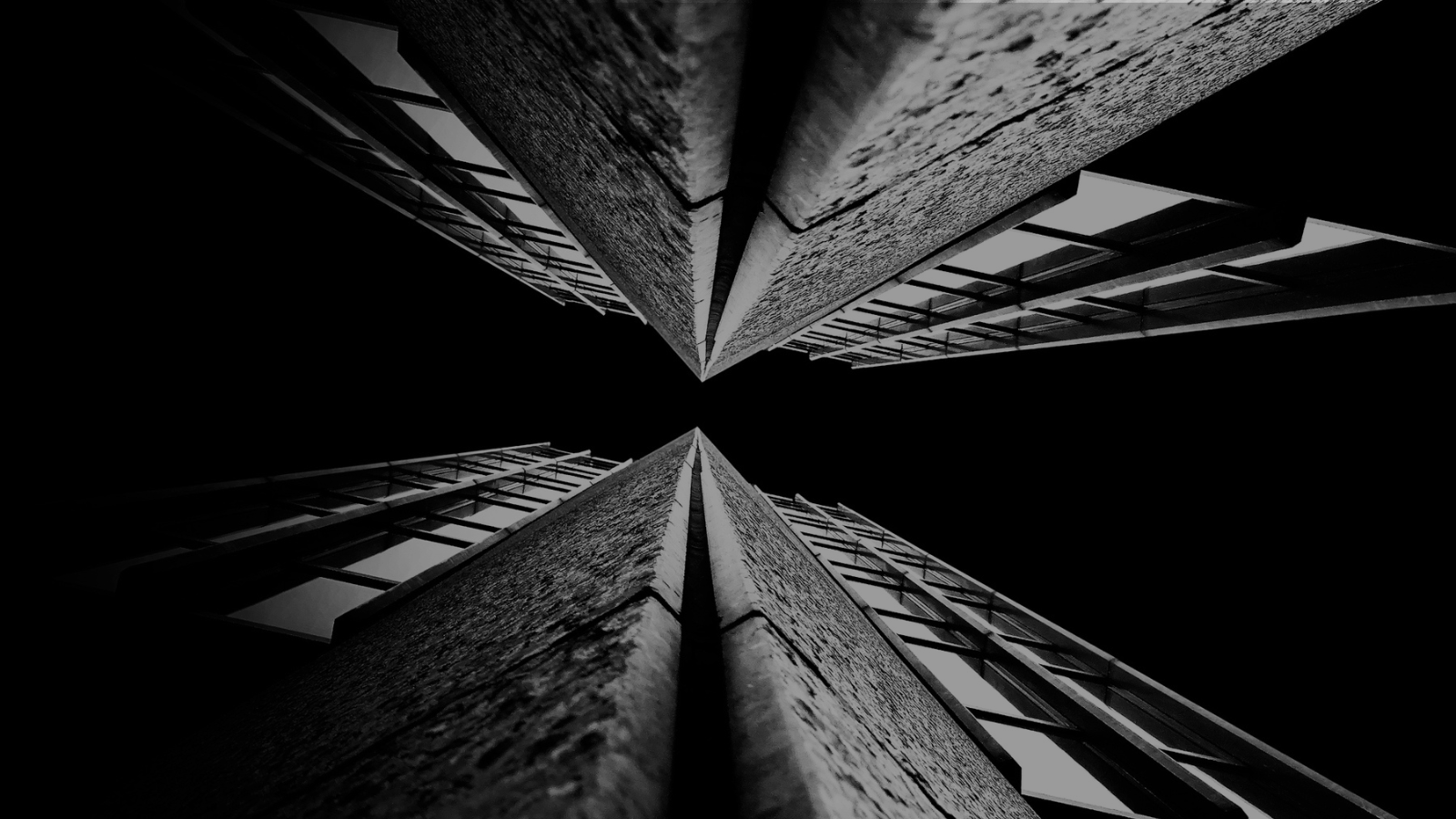
Oct 22
0
min
The CISO's Dilemma: Securing Snowflake's ACCOUNTADMIN Role
For years (even decades) sensitive information has lived in transactional and analytical databases in the data center. Firewalls, VPNs, Database Activity Monitors, Encryption solutions, Access Control solutions, Privileged Access Management and Data Loss Prevention tools were all purchased and assembled to sit in front of, and around, the databases housing this sensitive information.
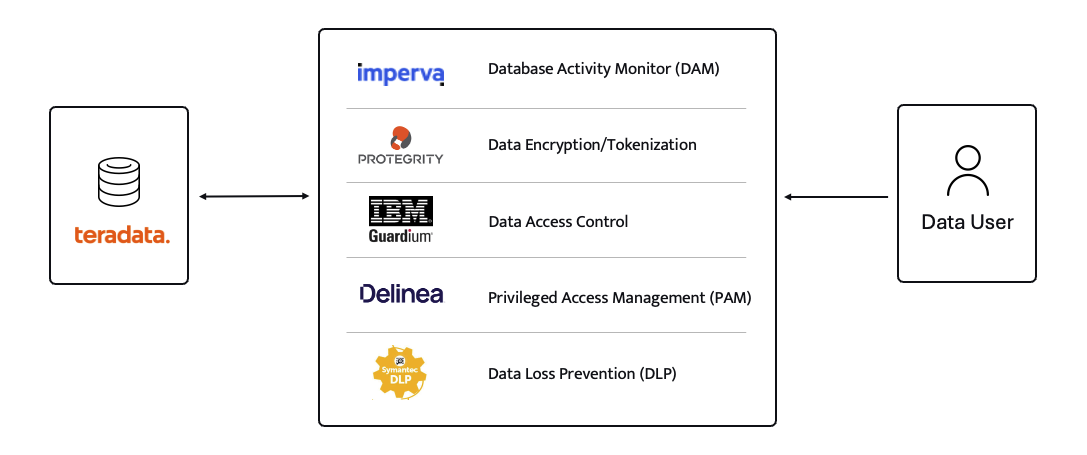
Even with all of the above solutions in place, CISO’s and security teams were still a nervous wreck. The goal of delivering data to the business was met, but that does not mean the teams were happy with their solutions. But we got by.
The advent of Big Data and now Generative AI are causing businesses to come to terms with the limitations of these on-prem analytical data stores. It’s hard to scale these systems when the compute and storage are tightly coupled. Sharing data with trusted parties outside the walls of the data center securely is clunky at best, downright dangerous in most cases. And forget running your own GenAI models in your datacenter unless you can outbid Larry, Sam, Satya, and Elon at the Nvidia store. These limits have brought on the era of cloud data platforms. These cloud platforms address the business needs and operational challenges, but they also present whole new security and compliance challenges.
ALTR’s platform has been purpose-built to recreate and enhance these protections required to use Teradata for Snowflake. Our cutting-edge SaaS architecture is revolutionizing data migrations from Teradata to Snowflake, making it seamless for organizations of all sizes, across industries, to unlock the full potential of their data.
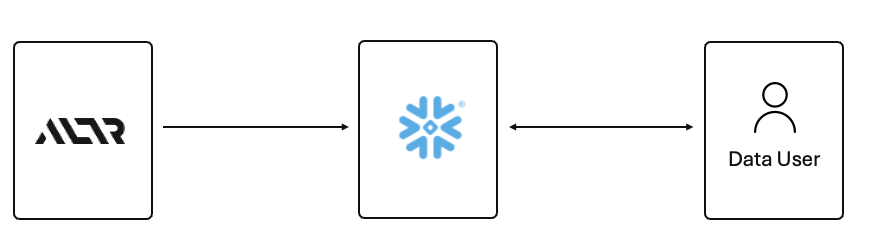
What spurred this blog is that a company reached out to ALTR to help them with data security on Snowflake. Cool! A member of the Data & Analytics team who tried our product and found love at first sight. The features were exactly what was needed to control access to sensitive data. Our Format-Preserving Encryption sets the standard for securing data at rest, offering unmatched protection with pricing that's accessible for businesses of any size. Win-win, which is the way it should be.
Our team collaborated closely with this person on use cases, identifying time and cost savings, and mapping out a plan to prove the solution’s value to their organization. Typically, we engage with the CISO at this stage, and those conversations are highly successful. However, this was not the case this time. The CISO did not want to meet with our team and practically stalled our progress.
The CISO’s point of view was that ALTR’s security solution could be completely disabled, removed, and would not be helpful in the case of a compromised ACCOUNTADMIN account in Snowflake. I agree with the CISO, all of those things are possible. Here is what I wanted to say to the CISO if they had given me the chance to meet with them!
The ACCOUNTADMIN role has a very simple definition, yet powerful and long-reaching implications of its use:

One of the main points I would have liked to make to the CISO is that as a user of Snowflake, their responsibility to secure that ACCOUNTADMIN role is squarely in their court. By now I’m sure you have all seen the news and responses to the Snowflake compromised accounts that happened earlier this year. It is proven that unsecured accounts by Snowflake customers caused the data theft. There have been dozens of articles and recommendations on how to secure your accounts with Snowflake and even a mandate of minimum authentication standards going forward for Snowflake accounts. You can read more information here, around securing the ACCOUNTADMIN role in Snowflake.
I felt the CISO was missing the point of the ALTR solution, and I wanted the chance to explain my perspective.
ALTR is not meant to secure the ACCOUNTADMIN account in Snowflake. That’s not where the real risk lies when using Snowflake (and yes, I know—“tell that to Ticketmaster.” Well, I did. Check out my write-up on how ALTR could have mitigated or even reduced the data theft, even with compromised accounts). The risk to data in Snowflake comes from all the OTHER accounts that are created and given access to data.
The ACCOUNTADMIN role is limited to one or two people in an organization. These are trusted folks who are smart and don’t want to get in trouble (99% of the time). On the other hand, you will have potentially thousands of non-ACCOUNTADMIN users accessing data, sharing data, screensharing dashboards, re-using passwords, etc. This is the purpose of ALTR’s Data Security Platform, to help you get a handle on part of the problem which is so large it can cause companies to abandon the benefits of Snowflake entirely.
There are three major issues outside of the ACCOUNTADMIN role that companies have to address when using Snowflake:
1. You must understand where your sensitive is inside of Snowflake. Data changes rapidly. You must keep up.
2. You must be able to prove to the business that you have a least privileged access mechanism. Data is accessed only when there is a valid business purpose.
3. You must be able to protect data at rest and in motion within Snowflake. This means cell level encryption using a BYOK approach, near-real-time data activity monitoring, and data theft prevention in the form of DLP.
The three issues mentioned above are incredibly difficult for 95% of businesses to solve, largely due to the sheer scale and complexity of these challenges. Terabytes of data and growing daily, more users with more applications, trusted third parties who want to collaborate with your data. All of this leads to an unmanageable set of internal processes that slow down the business and provide risk.
ALTR’s easy-to-use solution allows Virgin Pulse Data, Reporting, and Analytics teams to automatically apply data masking to thousands of tagged columns across multiple Snowflake databases. We’re able to store PII/PHI data securely and privately with a complete audit trail. Our internal users gain insight from this masked data and change lives for good.
- Andrew Bartley, Director of Data Governance
I believed the CISO at this company was either too focused on the ACCOUNTADMIN problem to understand their other risks, or felt he had control over the other non-admin accounts. In either case I would have liked to learn more!
There was a reason someone from the Data & Analytics team sought out a product like ALTR. Data teams are afraid of screwing up. People are scared to store and use sensitive data in Snowflake. That is what ALTR solves for, not the task of ACCOUNTADMIN security. I wanted to be able to walk the CISO through the risks and how others have solved for them using ALTR.
The tools that Snowflake provides to secure and lock down the ACCOUNTADMIN role are robust and simple to use. Ensure network policies are in place. Ensure MFA is enabled. Ensure you have logging of ACCOUNTADMIN activity to watch all access.
I wish I could have been on the conversation with the CISO to ask a simple question, “If I show you how to control the ACCOUNTADMIN role on your own, would that change your tone on your teams use of ALTR?” I don’t know the answer they would have given, but I know the answer most CISO’s would give.
Nothing will ever be 100% secure and I am by no means saying ALTR can protect your Snowflake data 100% by using our platform. Data security is all about reducing risk. Control the things you can, monitor closely and respond to the things you cannot control. That is what ALTR provides day in and day out to our customers. You can control your ACCOUNTADMIN on your own. Let us control and monitor the things you cannot do on your own.

Oct 14
0
min
How Cloud Data Security Enables Business Outcomes without Sacrificing Compliance
Since 2015 the migration of corporate data to the cloud has rapidly accelerated. At the time it was estimated that 30% of the corporate data was in the cloud compared to 2022 where it doubled to 60% in a mere seven years. Here we are in 2024, and this trend has not slowed down.
Over time, as more and more data has moved to the cloud, new challenges have presented themselves to organizations. New vendor onboarding, spend analysis, and new units of measure for billing. This brought on different cloud computer-related cost structures and new skillsets with new job titles. Vendor lock-in, skill gaps, performance and latency and data governance all became more intricate paired with the move to the cloud. Both operational and transactional data were in scope to reap the benefits promised by cloud computing, organizational cost savings, data analytics and, of course, AI.
The most critical of these new challenges revolve around a focus on Data Security and Privacy. The migration of on-premises data workloads to the Cloud Data Warehouses included sensitive, confidential, and personal information. Corporations like Microsoft, Google, Meta, Apple, Amazon were capturing every movement, purchase, keystroke, conversation and what feels like thought we ever made. These same cloud service providers made this easier for their enterprise customers to do the same. Along came Big Data and the need for it to be cataloged, analyzed, and used with the promise of making our personal lives better for a cost. The world's population readily sacrificed privacy for convenience.
The moral and ethical conversation would then begin, and world governments responded with regulations such as GDPR, CCPA and now most recently the European Union’s AI Act. The risk and fines have been in the billions. This is a story we already know well. Thus, Data Security and Privacy have become a critical function primarily for the obvious use case, compliance, and regulation. Yet only 11% of organizations have encrypted over 80% of their sensitive data.
With new challenges also came new capabilities and business opportunities. Real time analytics across distributed data sources (IoT, social media, transactional systems) enabling real time supply chain visibility, dynamic changes to pricing strategies, and enabling organizations to launch products to market faster than ever. On premise applications could not handle the volume of data that exists in today’s economy.
Data sharing between partners and customers became a strategic capability. Without having to copy or move data, organizations were enabled to build data monetization strategies leading to new business models. Now building and training Machine Learning models on demand is faster and easier than ever before.
To reap the benefits of the new data world, while remaining compliant, effective organizations have been prioritizing Data Security as a business enabler. Format Preserving Encryption (FPE) has become an accepted encryption option to enforce security and privacy policies. It is increasingly popular as it can address many of the challenges of the cloud while enabling new business capabilities. Let’s look at a few examples now:
Real Time Analytics - Because FPE is an encryption method that returns data in the original format, the data remains useful in the same length, structure, so that more data engineers, scientists and analysts can work with the data without being exposed to sensitive information.
Data Sharing – FPE enables data sharing of sensitive information both personal and confidential, enabling secure information, collaboration, and innovation alike.
Proactive Data Security– FPE allows for the anonymization of sensitive information, proactively protecting against data breaches and bad actors. Good holding to ransom a company that takes a more proactive approach using FPE and other Data Security Platform features in combination.
Empowered Data Engineering – with FPE data engineers can still build, test and deploy data transformations as user defined functions and logic in stored procedures or complied code will run without failure. Data validations and data quality checks for formats, lengths and more can be written and tested without exposing sensitive information. Federated, aggregation and range queries can still run without fail without the need for decryption. Dynamic ABAC and RBAC controls can be combined to decrypt at runtime for users with proper rights to see the original values of data.
Cost Management – While FPE does not come close to solving Cost Management in its entirety, it can definitely contribute. We are seeing a need for FPE as an option instead of replicating data in the cloud to development, test, and production support environments. With data transfer, storage and compute costs, moving data across regions and environments can be really expensive. With FPE, data can be encrypted and decrypted with compute that is a less expensive option than organizations' current antiquated data replication jobs. Thus, making FPE a viable cost savings option for producing production ready data in non-production environments. Look for a future blog on this topic and all the benefits that come along.
FPE is not a silver bullet for protecting sensitive information or enabled these business use cases. There are well documented challenges in the FF1 and FF3-1 algorithms (another blog on that to come). A blend of features including data discovery, dynamic data masking, tokenization, role and attribute-based access controls and data activity monitoring will be needed to have a proactive approach towards security within your modern data stack. This is why Gartner considers a Data Security Platform, like ALTR, to be one of the most advanced and proactive solutions for Data security leaders in your industry.

Oct 2
0
min
What is Format-Preserving Encryption & Why It’s the Missing Piece in Your Security Strategy
Securing sensitive information is now more critical than ever for all types of organizations as there have been many high-profile data breaches recently. There are several ways to secure the data including restricting access, masking, encrypting or tokenization. These can pose some challenges when using the data downstream. This is where Format Preserving Encryption (FPE) helps.
This blog will cover what Format Preserving Encryption is, how it works and where it is useful.
What is Format Preserving Encryption?
Whereas traditional encryption methods generate ciphertext that doesn't look like the original data, Format Preserving Encryption (FPE) encrypts data whilst maintaining the original data format. Changing the format can be an issue for systems or humans that expect data in a specific format. Let's look at an example of encrypting a 16-digit credit card number:

As you can see with a Standard Encryption type the result is a completely different output. This may result in it being incompatible with systems which require or expect a 16-digit numerical format. Using FPE the encrypted data still looks like a valid 16-digit number. This is extremely useful for where data must stay in a specific format for compatibility, compliance, or usability reasons.
>>>You Might Also Like: FPE vs Tokenization vs TSS
How does Format Preserving Encryption work?
Format Preserving Encryption in ALTR works by first analyzing the column to understand the input format and length. Next the NIST algorithm is applied to encrypt the data with the given key and tweak. ALTR applies regular key rotation to maximize security. We also support customers bringing their own keys (BYOK). Data can then selectively be decrypted using ALTR’s access policies.
Why use Format Preserving Encryption
FPE offers several benefits for organizations that deal with structured data:
1. Adds extra layer of protection: Even if a system or database is breached the encryption makes sensitive data harder to access.
2. Original Data Format Maintained: FPE preserves the original data structure. This is critical when the data format cannot be changed due to system limitations or compliance regulations.
3. Improves Usability: Encrypted data in an expected format is easier to use, display and transform.
4. Simplifies Compliance: Many regulations like PCI-DSS, HIPAA, and GDPR will mandate safeguarding, such as encryption, of sensitive data. FPE allows you to apply encryption without disrupting data flows or reporting, all while still meeting regulatory requirements.
When to use Format Preserving Encryption?
FPE is widely adopted in industries that regularly handle sensitive data. Here are a few common use cases:
- Healthcare: Hospitals and healthcare providers could use FPE to protect Social Security numbers, patient IDs, and medical records. It ensures sensitive information is encrypted while retaining the format needed for billing and reporting.
- Telecoms: Telecom companies can encrypt phone numbers and IMSI (International Mobile Subscriber Identity) numbers with FPE. This allows the data to be securely transmitted and processed in real-time without decryption.
- Government and Defense: Government agencies can use FPE to safeguard data like passport numbers and classified information. Preserving the format ensures seamless data exchange across systems without breaking functionality.
- Data Sharing: In this blog we talk about how FPE can help with Snowflake Data Sharing use cases.
Wrapping Up
ALTR offers various masking, tokenization and encryption options to keep all your Snowflake data secure. Our customers are seeing the benefit of Format Preserving Encryption to enhance their data protection efforts while maintaining operational efficiency and compliance. For more information, schedule a product tour or visit the Snowflake Marketplace.
Browse All
Thank you! Your submission has been received!
Oops! Something went wrong while submitting the form.
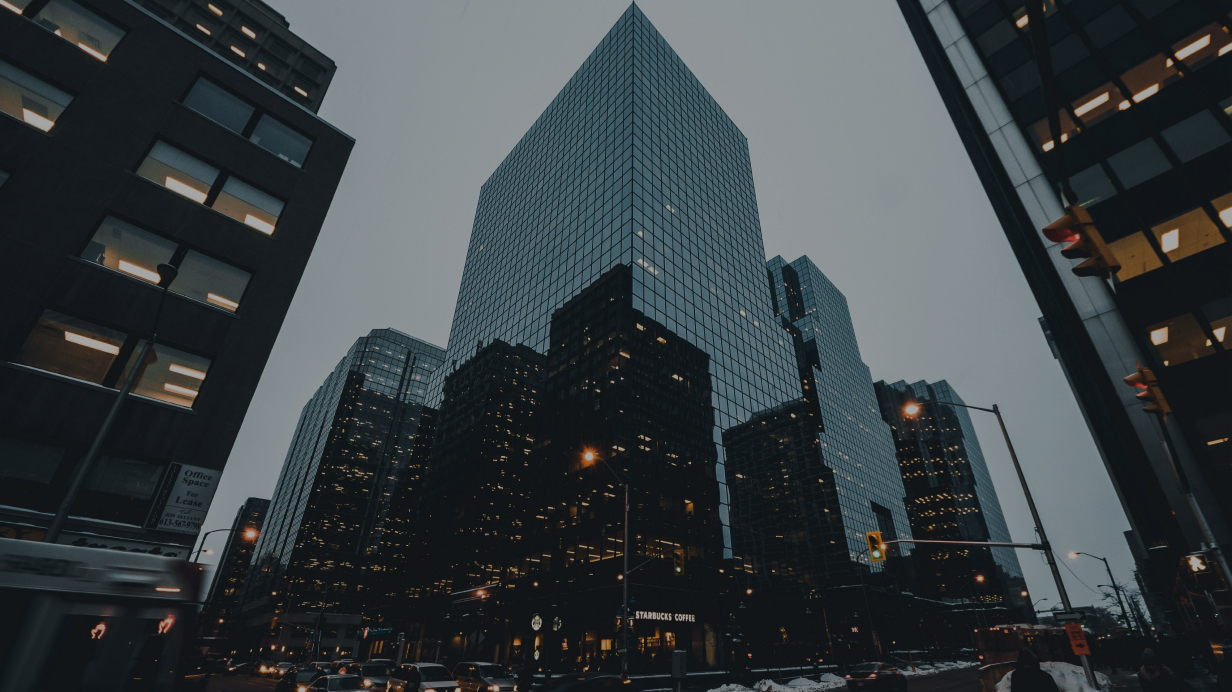
Jan 10
0
min
How to Build a Data Governance-Centric Company Culture
ALTR Blog
Prioritizing data governance can provide organizations with a significant competitive advantage. However, according to a Gartner survey, more than 90% of data governance projects struggle to achieve their objectives. From lack of support from senior executives to confusion surrounding roles and responsibilities, this underperformance can be attributed to various factors. Consequently, cultivating a data governance-centric company culture is more critical than ever. Such a culture is indispensable for ensuring data accuracy, security, and compliance while unlocking the full potential of data to inform strategic decisions. In this blog, we will delve into the key strategies for establishing a data governance-centric company culture that empowers employees and maximizes the value derived from data.
Ensure Leadership Commitment
Building a data governance-centric culture begins with solid leadership at the helm. Leadership commitment is the cornerstone of shaping an organizational culture that places a premium on data governance. It encompasses leaders at every level, from the CEO to the CDO and CISO, who need to grasp the strategic significance of data and actively champion its governance within the company. This commitment should be evident not only in their words but also in their actions, serving as a guiding principle that permeates throughout the organization.
Leaders should:
- Clearly articulate the importance of data governance in achieving business goals
- Allocate resources and budget for data governance initiatives
- Lead by example by adhering to data governance policies themselves
Define Clear Roles and Responsibilities
In a data governance-centric culture, everyone in the organization should understand their roles and responsibilities related to data management. Define clear job descriptions and expectations. Include roles such as:
Data Owners: Data owners are accountable for the overall governance and decision-making related to specific datasets or data assets.
Data stewards: Data stewards are individuals responsible for the quality, integrity, and overall management of specific sets of data or data domains.
Data Custodians: Data custodians are responsible for the technical aspects of data management, including storage, maintenance, and protection.
These roles should collaborate closely to ensure comprehensive data governance within an organization.
Establish Data Governance Policies and Procedures
Establish clear policies and procedures to ensure consistency and adherence to data governance principles. These should cover data classification, access controls, retention, privacy, and security. Ensure these policies are easily accessible to all employees and regularly updated to reflect evolving regulatory requirements and industry best practices.
Consider Data Utilization
Data governance should complement, not complicate, the daily activities of its members. Access to data is pivotal for informed decision-making and analytical insights. So, when employees encounter obstacles in obtaining the required data, it impedes their ability to perform their roles effectively and undermines the credibility and perceived value of data governance initiatives. To establish a compelling case for data governance, organizations must prioritize data accessibility by refining policies, promoting data democratization, and ensuring that data is readily available for those who need it. This approach enhances data utilization and cultivates a culture where data governance is seen as an essential enabler of data-driven success.
Provide the Best Data Governance Technologies
Equipping teams with cutting-edge tools and technologies empowers them to effectively manage, protect, and extract insights from data. From automated data access control platforms to advanced business intelligence and analytics tools, by staying at the forefront of technology, organizations can streamline data governance processes, enhance data quality, and bolster data security.
Offer Training and Education
A well-informed workforce is essential for a successful data governance-centric culture. Provide comprehensive training and educational resources to help employees understand the importance of data governance and how it applies to their roles. Offer ongoing training to keep everyone updated on new policies, procedures, and emerging data-related threats.
Training initiatives can include:
- Workshops and seminars on data governance best practices
- Data privacy and security awareness programs
- Certification programs for data professional
- Accessible online resources and documentation
Assure Data Quality
Data governance goes beyond policy implementation; it involves continuous monitoring and data quality assurance. According to Gartner, poor data quality costs organizations an average of $12.9 million. However, when employees actively preserve data integrity, their collective efforts contribute to improved data quality. This, in turn, strengthens trust in the data, as stakeholders can depend on established processes and systems to deliver reliable and consistent information. Organizations should establish data quality assurance processes that encompass regular audits, data profiling, and validation checks to achieve this. Additionally, it is essential to encourage employees to report any data quality issues and establish accessible channels to do so seamlessly.
Communicate and Collaborate
Effective communication and collaboration are critical for fostering a data governance-centric culture. Encourage cross-functional teams to work together on data-related initiatives and problem-solving. Use collaboration tools and platforms to facilitate communication and information sharing.
Regularly scheduled meetings and reports can help:
- Share data governance updates and progress
- Discuss data-related challenges and solutions
- Celebrate successes and recognize contributions
Measure and Monitor
To ensure the effectiveness of your data governance efforts, establish key performance indicators (KPIs) and metrics to measure progress. Regularly monitor these metrics and use them to identify areas for improvement. Some essential data governance metrics include data accuracy rates, data quality scores, compliance levels, and the number of data-related incidents.
Continuously Adapt and Improve
The data landscape is continually evolving. A data governance-centric culture must be adaptable and open to change. Encourage employees to suggest improvements to data governance policies and procedures. Foster a culture of continuous learning and improvement.
Reward and Recognize
Recognize and reward employees who demonstrate a commitment to data governance. Acknowledge their contributions and the positive impact of their efforts on the organization. Rewards can include promotions, bonuses, or other forms of recognition that align with your company's culture and values.
Wrapping Up
In today's data-driven business environment, a data governance-centric company culture is not just a nice-to-have; it's a necessity. Companies prioritizing data governance are better equipped to make informed decisions, protect sensitive information, and gain a competitive edge. Remember that creating and maintaining such a culture is an ongoing process, and adaptability and continuous improvement are vital to staying at the forefront of data management excellence.
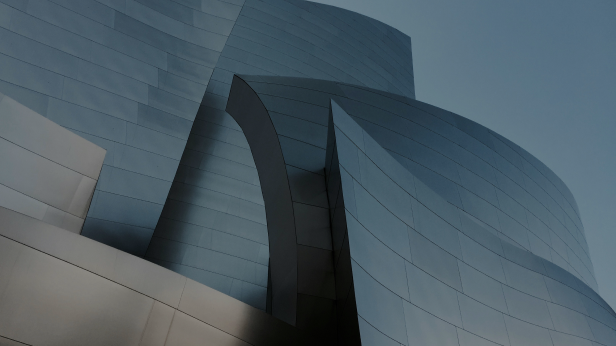
Jan 4
0
min
2024 Data Governance Trends and Predictions
ALTR Blog
As we venture into 2024, data governance is poised to undergo transformative changes. With the rapid advancements in technology, evolving regulations, and the growing need for data-driven decision-making, organizations must stay vigilant and adaptive in their data governance practices to ensure the security, privacy, and quality of their data assets.
In this article, we'll explore the top data governance trends and predictions for 2024, providing valuable insights to help you confidently navigate the evolving data governance landscape.
Data Democratization
Data control has historically been limited to a select few within organizations, leaving most users without access. A new era of data democratization is on the horizon, poised to reshape how organizations operate. The goal is to empower every user within an organization with the tools and information needed to leverage data effectively. Decision-makers across all levels, from executives to frontline employees, will gain the capability to analyze data, extract insights, and make informed decisions. This transformation will not only revolutionize organizational dynamics but also significantly impact data governance.
Data governance must ensure responsible data usage, protect sensitive information, and maintain data quality. Organizations will need to implement robust governance protocols, including access controls, data classification, and tokenization or format-preserving encryption, to strike a balance between accessibility and security. Proper training and education programs will also be essential to promote responsible data practices among employees.
Shift Left Data Governance
In 2024, Shift Left™ Data Governance will seize the spotlight, ushering in a transformative era in data security practices. This paradigm shift revolves around a proactive approach to securing sensitive data. It begins its protection journey right from the moment data departs the source system and continues throughout its voyage to the cloud or data warehouse.
To embrace 'Shift Left Data Governance,' organizations will leverage cutting-edge technologies such as ALTR, empowering them to extend data governance measures upstream into data pipelines, ETL/ELT processes, and data catalogs. Data governance policies encompassing data classification, access controls, encryption, and anonymization will seamlessly intertwine with these early-stage processes. As a result, data becomes subject to governance and protection from the very inception of its journey, effectively addressing security vulnerabilities that may exist before data reaches its intended destination.
The Shift Left™ approach will evolve into an indispensable capability for modern data enterprises, significantly reducing the risks associated with unauthorized access, data breaches, and privacy infringements. Simultaneously, it fortifies data security throughout the entire data journey, ensuring comprehensive safeguarding.
AI Governance
As organizations increasingly embrace AI, effective AI governance becomes paramount in sustaining success and managing risks. In 2024, AI governance will revolve around foundational principles encompassing regulatory compliance, ethics, transparency, and privacy.
A central tenet of AI governance will focus on the reliability of data. In the era of AI-driven transformation, trustworthy data is the cornerstone of successful AI, facilitating innovation while adhering to ethical and regulatory standards. Organizations will prioritize data quality to mitigate risks related to biased decision-making, inaccuracies, and security, privacy, and legal compliance concerns.
Integrating AI governance into existing processes will be both challenging and essential. This integration will comprehensively evaluate current data management and governance practices, policy development and refinement, workflow alignment, technology integration, and risk management. Organizations may establish AI governance steering committees or working groups to oversee this process, ensuring comprehensive coverage and creating a culture of curiosity and learning to foster broader organizational engagement.
Automated Data Governance
In 2024, the rise of automation in data governance and security is poised to become a dominant trend within the dynamic realm of data management. Although some data systems and platforms offer inherent features for data governance and access control, harnessing these capabilities often demands substantial SQL scripting and extensive involvement from DBAs or data engineers for implementation and upkeep. Alternatively, certain platforms necessitate a separate layer of data governance and security, leading to cumbersome processes. Consequently, orchestrating intricate data governance rules and policies can consume weeks, if not months, before new data sets and workloads become accessible to users. As companies intensify their utilization of data resources, this challenge compounds, with the only effective remedy being automation.
Key capabilities such as data classification, role-based access controls, data masking, rate limiting, real-time alerting, and tokenization are now readily available and scalable through user-friendly, point-and-click interfaces or direct API integration. These automation tools have the transformative power to significantly truncate the time required for new data and workloads to be provisioned for users. By eliminating weeks, and often months, from this process, companies will substantially expedite their time-to-value, providing a decisive edge in the rapidly evolving data landscape.
Wrapping Up
In 2024, data governance is not just a strategy; it's a strategic imperative. It's the driving force behind secure data access, compliance with stringent regulations, and the ability to derive actionable insights from the vast sea of data. The seamless integration of data governance into an organization's DNA fosters a culture of data-driven decision-making, empowers users at all levels, and positions them to navigate the complexities of a data-centric world.
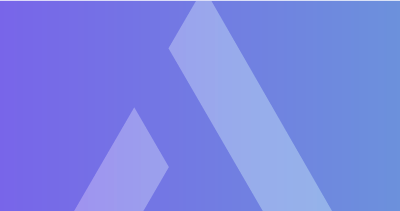
Jan 3
0
min
ALTR Featured as a Partner of the Snowflake Horizon Ecosystem
ALTR Blog
With more and more businesses opting to derive valuable insights from their data on the Snowflake Data Cloud, safely managing sensitive data has emerged as a top priority for data driven organizations. ALTR has worked closely with Snowflake since our partnership began in 2020, building and continuing to foster our SaaS-based, cloud-native integration. ALTR's SaaS solution has been recognized as a Snowflake Premier Technology Partner with a Snowflake Financial Services Competency badge. ALTR’s primary focus is on delivering best-in-class data access governance and integrated data security over data in Snowflake, designed to make customers more successful on Snowflake, more quickly.
ALTR takes Snowflake’s powerful native data governance capabilities and automates them at scale to deliver real-time data access monitoring and analytics, point-and-click policy-based access controls, and advanced data protection. These features are all delivered as pure SaaS with no code required to implement, scale, and maintain. By automating Snowflake’s native capabilities with ALTR, customers maximize the value of their Snowflake investment, enhance their data governance maturity, and solidify their data security posture.
ALTR + the Snowflake Horizon Partner Ecosystem
ALTR is proud to be a part of the Snowflake Horizon Partner Ecosystem, Snowflake’s built-in governance solution with a unified set of compliance, security, privacy, interoperability, and access capabilities. ALTR’s partnership with Snowflake will help further extend the Snowflake Data Cloud across customers’ data stacks. ALTR continuously integrates the latest features and capabilities offered by Snowflake into our SaaS solution, enabling joint customers to take advantage of Snowflake's native capabilities easily and efficiently, with immediate time to value.
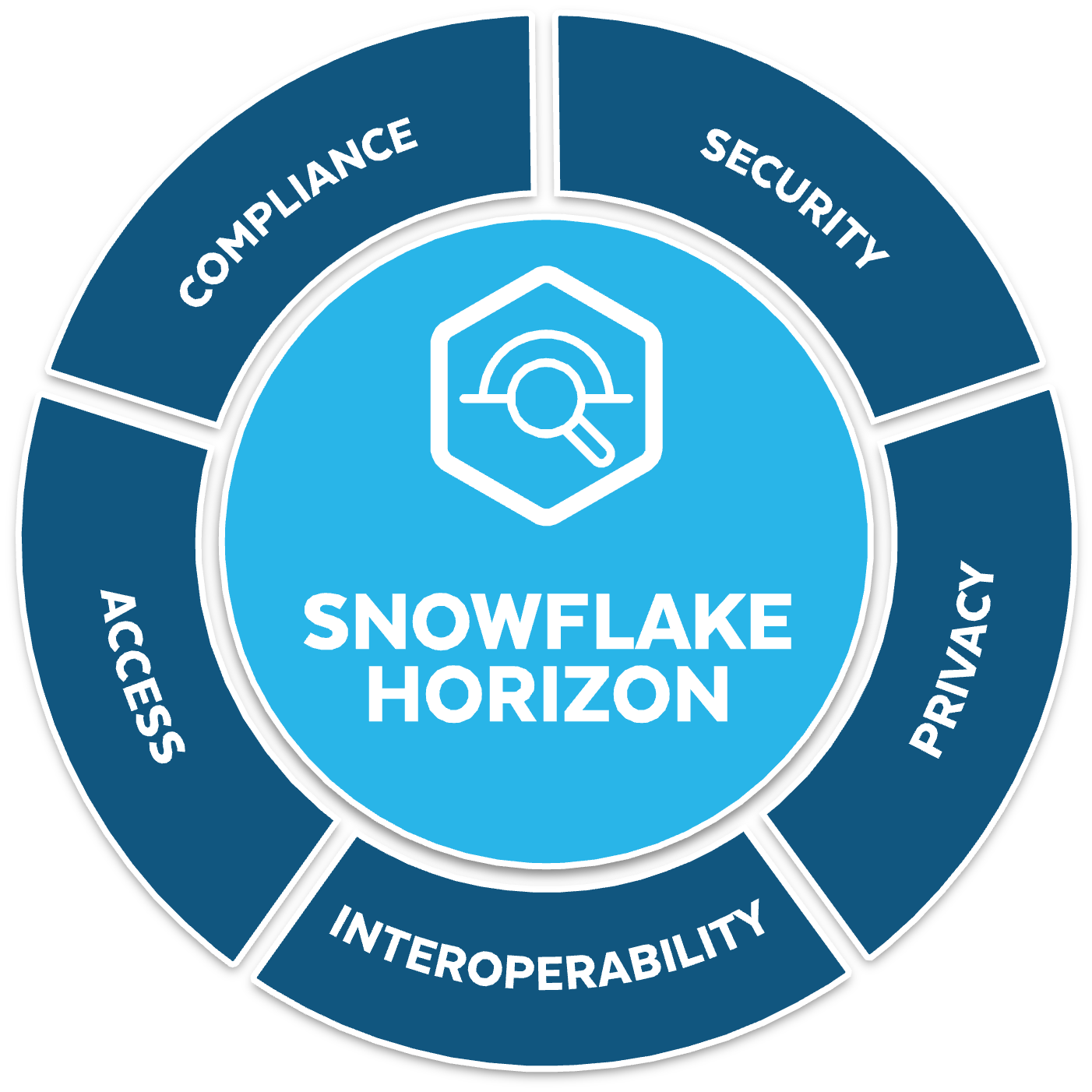
How ALTR Helps Customers Safeguard Data within Snowflake Horizon
Data Classification
With ALTR and Snowflake, data users can automatically classify their data and receive classification results in minutes. Snowflake clients can select from multiple methods for data classification: Snowflake Native, any third-party classification engine, or a productized GDLP plug-in integrated in ALTR. Together, customers can automate the discovery and classification process without writing any code, allowing businesses to derive business critical insights from their data in a matter of minutes. Using ALTR’s Shift Left data governance capabilities, data classification can be moved upstream in to ETL/ELT pipelines to classify and tag data before it lands in Snowflake.
Real-time Observability over Sensitive Data Access
With ALTR, customers can achieve real-time observability over how users access sensitive data in Snowflake, regardless of access point. ALTR logs all data access into an easy-to-consume query log, which can be published in real-time to a client owned S3 bucket, enabling any SIEM tool to ingest real-time data access telemetry for analysis and visualization. These access logs are visualized directly in ALTR’s product in heatmap format. This feature helps data users analyze and report on data access, ensure that governance policies are being correctly enforced, and pinpoint areas where new policies can be implemented. ALTR records the metadata over each query for governed data, along with user, time, and the number of values returned, providing visibility to understand normal patterns, and easily spot abnormalities that could indicate risk. With every query recorded, compliance audits become simplified and streamlined, giving customers complete and real-time transparency to all attempted access requests of their sensitive data.
Dynamic Data Masking & Automated Access Controls
ALTR greatly simplifies the implementation and maintenance of complex and granular data masking policies to safeguard confidential information in Snowflake. Using ALTR’s point and click UI, customers can effortlessly view the data and roles to which their policies apply, easily create new policies and modify existing ones, all without requiring any SQL coding. Further, all policy orchestration and management can be fully automated, at scale, using ALTR’s Management API. Data Masking with ALTR and Snowflake helps organizations meet regulatory requirements, such as GDPR, HIPPA, and PCI DSS by protecting sensitive data and ensuring privacy. Snowflake clients rapidly realize the enormous value of ALTR’s policy automation capabilities through eliminating the reliance on data engineering resources to manage access control changes. ALTR democratizes access policy management to non-technical users, frees up Data Engineering to focus on higher value tasks, and enables access control changes in minutes versus days or weeks.
Advanced Data Protection – Purpose Built for Snowflake
With ALTR, customers gain access to a wide range of techniques for obfuscating and anonymizing data, such as Format Preserving Encryption and External Tokenization, giving data users the freedom to choose an advanced data protection model that best fits their business needs.
ALTR combines advanced data protection with policy to ensure no sensitive data can be accessed outside of approved policy. ALTR sits in the critical path of data and creates a compliance-ready, audit rich query log of all requests for data subject to ALTR’s advanced data protection. Any data that is subject to compliance regulations like HIPAA, GDPR, PCI, or any forthcoming privacy rules, is protected within ALTR’s SaaS based product. Further, ALTR’s query audits perfect the chain of custody over sensitive data and reflect any time protected values are de-tokenized or decrypted.
ALTR Delivers Real-Time Alerting & Notifications
ALTR’s unique, pure SaaS solution offers a distinctive set of features that ensure the security of data in Snowflake, such as data tokenization, format preserving encryption, user access controls, and real-time alerting. Only ALTR can ensure that your sensitive data is accessible only to the appropriate people, at the appropriate time, and in the appropriate amounts. ALTR’s patent-issued Data Rate Limiting means that out of policy requests for data can be blocked in real-time and single users can be quarantined without impacting other users with the same role. Data Owners and InfoSec Teams can trust that notifications will be delivered immediately through their preferred communication channel, like Slack, Teams, or email, anytime anyone attempts to access sensitive data without authorization. Only ALTR offers active security for your most sensitive data assets in Snowflake that can stop credentialed access threats before they can happen.
Get Started for Free Today
With ALTR’s native integrations in Snowflake Data Cloud, we’re proud to be a part of the Snowflake Horizon Partner Ecosystem and are thrilled to continue our extensive partnership with Snowflake. ALTR's free integration in Snowflake Partner Connect allows data users to drastically reduce manual tasks to deliver more data value, more quickly.
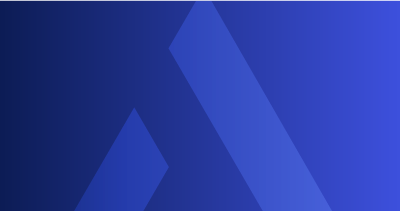
Dec 20
0
min
Harnessing Automation for Effective Data Access Control
ALTR Blog
In our hyper-connected, data-rich landscape, safeguarding and prudent data management have emerged as paramount concerns for organizations of all sizes. The digital age has ushered in an era where data is a strategic asset and a potential liability. Enter data access control, a robust sentinel in the realm of data governance, and a standing guard to ensure that the gates of sensitive information are opened only to those with the proper credentials.
What is Data Access Control
Data access control is a multifaceted security mechanism designed to manage and regulate access to data resources within an organization. It encompasses a set of policies, procedures, and technologies that ensure data is only accessible to authorized individuals or systems while preventing unauthorized access or manipulation. Access control defines who can access specific data, what actions they can perform (such as viewing, editing, or deleting), and under what circumstances. This fine-grained control helps organizations maintain data confidentiality, integrity, and availability while ensuring compliance with regulatory requirements. It is a critical component of data governance, protecting sensitive information from breaches, unauthorized disclosures, or alterations.
Types of Data Access Control
Access controls encompass various mechanisms and strategies to regulate and manage access to data and resources. Here are some of the primary types of access controls:
Role-Based Access Control (RBAC)
RBAC assigns permissions based on predefined roles within an organization. Users are assigned to specific roles, each associated with a set of permissions. This approach simplifies access management, as administrators can grant or revoke permissions at the role level rather than for individual users.
>>> You Might Also Like: Determining the Right Role-based Access Controls
Attribute-Based Access Control (ABAC)
ABAC is a dynamic access control model that takes into account various attributes, such as user attributes (e.g., department, job title), resource attributes (e.g., data classification, sensitivity), and environmental attributes (e.g., time of day, location). Access decisions are based on complex rules considering these attributes, providing fine-grained and context-aware access control.
Mandatory Access Control (MAC)
MAC enforces access controls based on security labels and user and data classifications. This model is commonly used in highly secure environments, such as government or military sectors, to ensure strict data confidentiality. Users have limited control over access, and security administrators typically make access decisions.
Discretionary Access Control (DAC)
DAC allows data owners to determine access permissions for their resources. In this model, data owners have discretion over who can access, modify, or delete their data. While it offers flexibility, DAC can lead to inconsistent access management and potential security risks if not carefully administered.
Rule-Based Access Control (RUBAC)
RUBAC enforces access controls based on predefined rules or policies. These rules can incorporate various conditions and factors, such as user attributes, resource characteristics, or contextual information. Access is granted or denied based on whether the conditions defined in the rules are met.
The Significance of Automation in Data Access Control
Automation is a linchpin in the modern data access control landscape, revolutionizing how organizations manage and safeguard their data. It leverages scripts, policies, and specialized tools to streamline the intricate management of data access permissions and processes.
Reducing Human Error
Imagine a large financial institution managing thousands of employees' access rights across multiple systems and databases. In a manual access control scenario, the likelihood of human error, such as accidentally granting excessive privileges or failing to revoke access promptly upon an employee's departure, is significant. However, with automated data access control, permissions are consistently and accurately applied. For instance, when employees change roles, this automated platform can promptly adjust their access privileges, minimizing the chances of data breaches and compliance violations.
Enhancing Efficiency
Picture an e-commerce giant during a holiday season sales rush, where millions of customers are accessing its online platform simultaneously. Manually updating access permissions for each user or resource to accommodate this surge would be an insurmountable task. Yet, automated data access control comes to the rescue. The organization efficiently scales its operations by automating the provision of temporary access privileges based on predefined criteria (e.g., high website traffic). This not only saves precious time but also empowers security teams to focus on strategic initiatives, such as identifying emerging threats or refining access policies.
Consistency and Standardization
In a sprawling multinational corporation, data access control sprawls across multiple departments, each managing its resources and user permissions. Maintaining consistency and standardization in access control policies would be a Herculean task without automation. Consider an employee who moves between departments or regions. Automation ensures that access policies are predefined and uniformly applied across the organization. When this employee transitions, automated processes swiftly and accurately adjust their access rights, minimizing confusion and ensuring data security across the board.
Rapid Response to Changes
Access requirements can change at a moment's notice in the fast-paced realm of cybersecurity. Consider an e-commerce retailer responding to a sudden surge in cyberattacks targeting customer data. Automation shines as a dynamic responder to such threats. It enables organizations to adapt swiftly to changing access needs by provisioning or revoking access in real time based on predefined criteria. For instance, in response to a detected breach, an automated system can instantly suspend access privileges, isolating the affected data and averting further security incidents.
Auditing and Compliance
In an environment governed by strict regulatory frameworks, the importance of auditability cannot be overstated. A healthcare provider, for instance, must meticulously track and report who accessed patient records and when. Maintaining comprehensive audit logs would be manual and error-prone without automation. Automation systematically generates detailed audit logs and reports, serving as an invaluable regulatory compliance and security monitoring resource. Organizations can swiftly respond to compliance queries, detect suspicious activities, and conduct thorough incident investigations by tracking who accessed what data and when.
Wrapping Up
Data access control is a fundamental component of data governance, ensuring that data remains secure, compliant, and accessible only to authorized users. By understanding the types of data access control, implementing best practices, and leveraging tools like ALTR for advanced automated data access control, organizations can safeguard their data assets and maintain the trust of their customers and stakeholders in today's data-driven world.
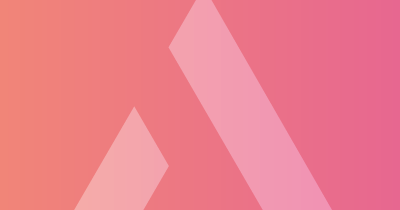
Dec 8
0
min
Privacy by Design: The Paradigm Shift That Secures Our Digital Future
ALTR Blog
In the last two years, we have witnessed a remarkable transformation in how companies approach data protection. The era of privacy breaches and data mishandling scandals has compelled a profound change in mindset, putting Privacy by Design at the forefront of our digital landscape. This evolving approach not only aligns businesses with the shifting legal landscape but also fosters consumer trust and reduces risks, ushering in a new era of responsible data management. Indeed, the recognition that data protection is here to stay is dawning upon everyone - from corporations and legislators to conscientious consumers.
The Evolution of Privacy by Design
Privacy by Design, as a concept, isn't new. Dr. Ann Cavoukian, Ontario's former Information and Privacy Commissioner, coined the term in the 1990s. It emphasizes integrating data protection principles into the fabric of technology and business processes from the outset. However, this approach has gained unprecedented traction and importance in recent years.
The catalyst for this change is evident. High-profile data breaches, such as the Facebook-Cambridge Analytica scandal and countless others, have eroded trust in corporations and exposed the vulnerabilities in our data-driven society. As a result, governments worldwide have responded to stringent data protection regulations, such as the European Union's General Data Protection Regulation (GDPR) and the California Consumer Privacy Act (CCPA).
Companies can no longer afford to view data protection as a mere compliance issue. Instead, it must become a fundamental aspect of their corporate DNA. Privacy by Design does precisely that by infusing privacy principles into every facet of an organization.
The Cost-Efficiency of Privacy by Design
One might argue that integrating privacy into the design process adds an extra layer of complexity and cost to business operations. However, the opposite is true. Privacy by Design is not a cost but an investment.
By proactively building privacy measures into products and services, companies can reduce the potential for costly data breaches and regulatory fines. Under GDPR, for instance, non-compliance can result in fines of up to €20 million or 4% of global annual revenue - a substantial sum far exceeding the cost of implementing strong data protection practices.
Moreover, adopting Privacy by Design from the start eliminates the need for costly retroactive adjustments to comply with new data protection laws. It streamlines the adaptation process and ensures that data privacy is an inherent part of the corporate culture, reducing the risk of legal entanglements.
Building Trust and Fostering Consumer Confidence
In today's digital age, consumer trust is a currency as valuable as any other. Privacy breaches have eroded this trust, leaving consumers skeptical about how their personal data is handled. Companies prioritizing Privacy by Design send a clear message to their customers: "Your privacy matters to us."
This message resonates with consumers, who are increasingly cautious about sharing their data. Organizations that respect privacy build stronger, more lasting customer relationships. They become the preferred choice in a market flooded with options, demonstrating their commitment to safeguarding sensitive information.
Automated Data Governance: A Catalyst for Privacy by Design
In Privacy by Design, automated data governance is a powerful tool to fortify and streamline privacy practices across organizations. As data volumes continue to soar and the complexity of data ecosystems intensifies, manual data management and compliance become increasingly impractical. Automated data governance not only eases the burden but also ensures a proactive and comprehensive approach to privacy.
1. Data Classification and Protection
Automated data governance systems employ advanced algorithms to classify data based on sensitivity and relevance. This categorization ensures that sensitive information, such as personal identifiable information (PII), is treated with the utmost care and is subject to stricter access controls. Organizations can implement granular data protection measures by automatically classifying data and preserving privacy at every data processing stage.
2. Access Control and User Permissions
Privacy by Design demands robust access controls and user permissions to limit data access only to authorized personnel. Automated data governance solutions can enforce role-based access controls, ensuring that individuals can only access data necessary for their job functions. Additionally, they can automate the revocation of access rights when employees change roles or leave the organization, reducing the risk of unauthorized data access.
3. Tokenization
Automated data governance that leverages tokenization is a formidable ally in Privacy by Design, allowing organizations to safeguard sensitive data while maintaining its utility. By replacing sensitive information with unique tokens, tokenization minimizes data exposure and reduces the risk of data breaches. It simplifies compliance with data protection regulations, ensuring that personal information remains secure and private. This technique also fosters secure data sharing and analytics, enabling organizations to extract insights while preserving individual privacy. With its scalability, flexibility, and rapid response capabilities in the face of data breaches, tokenization is a pivotal tool for organizations committed to weaving privacy into the fabric of their data processes and systems.
4. Data Retention and Deletion Policies
Another critical component of Privacy by Design is the establishment of data retention and deletion policies. Automated data governance systems can track data lifecycle events, such as when data was created, accessed, and modified, to enforce data retention policies consistently. When data reaches the end of its useful life, automated processes can facilitate its secure and irreversible deletion, aligning with privacy principles of data minimization.
5. Data Impact Assessments
Privacy Impact Assessments (PIAs) are essential in evaluating the potential privacy risks associated with data processing activities. Automated data governance solutions can streamline the PIA process by providing a structured framework to identify, assess, and mitigate privacy risks. This automation ensures that privacy considerations are integrated into the design of new projects, products, or services.
6. Incident Response and Reporting
In the event of a data breach or privacy incident, time is of the essence. Automated data governance systems can expedite incident detection and response by triggering alerts and notifications when suspicious activities occur. Moreover, they facilitate the generation of comprehensive incident reports, which are invaluable for compliance reporting and communication with regulatory authorities.
Wrapping Up
Privacy by Design has emerged as the cornerstone of a responsible digital future, reducing costs, building trust, and mitigating risks. Automated data governance acts as a force multiplier, reducing compliance's administrative burden and enhancing the effectiveness and consistency of privacy practices within organizations. By seamlessly integrating automated data governance into the design and management of data, companies can achieve the delicate balance of innovation and privacy protection, fostering trust with consumers and regulators alike.

Dec 11
0
min
Q4 2023 ALTR Product: Helping Data Teams Ensure Data Security Earlier in the Data Lifecycle
ALTR Blog
ALTR’s final product release of 2023 is now live and helps data and security teams increase data utility and decrease data complexity by:
- Offering Detokenization on Snowflake for policy to be applied automatically on data.
- Increase data operability earlier in the data lifecycle, while remaining secure.
- Shifting data governance and data security left so data is protected in motion and at rest.
In order to ensure the protection of sensitive, highly regulated data, in motion and at rest, it is no longer enough to create security policies after data lands in Snowflake. Data tokenization must occur as early as possible in the data lifecycle, available only through ALTR’s SaaS based data security solution, so that the data lands in Snowflake already in a protected state.
Data tokenization can provide unique data security benefits across your entire data pipeline. ALTR’s SaaS-based approach to data tokenization-as-a-service means data can be tokenized at any stage of the data lifecycle. Tokenization is an incredibly powerful tool to have included in your arsenal, and we are thrilled to be announcing the extension of this offering.
ALTR Detokenization
We are proud to announce the launch of Detokenization on Snowflake. ALTR’s detokenization, when combined with Snowflake governance policy, is a powerful and highly innovative solution designed specifically to help Security teams and Data teams ensure data privacy and security as early as possible in the data lifecycle all the way through to data consumption.
Detokenization allows for data that exists in Snowflake to remain operational while policy can be applied automatically, furthering the ability to shift data governance and data security operations left in the data pipeline.
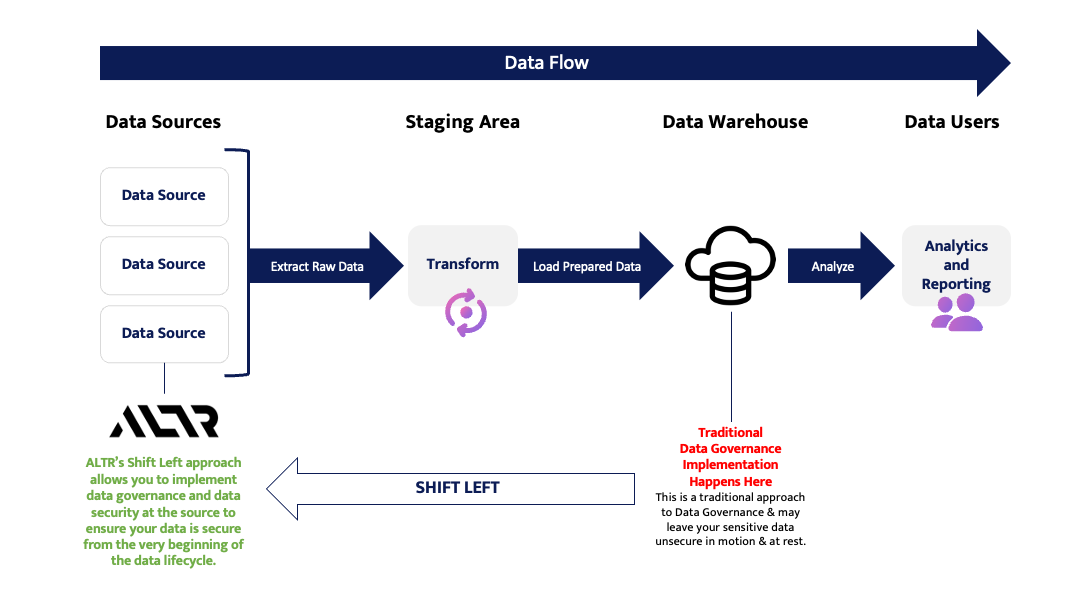
What is Detokenization on Snowflake?
Detokenization is the process of converting tokenized data back into its original, unmasked, form. Detokenization, combined with Snowflake’s governance policy, is a critical step in ensuring data privacy and security, especially when dealing with PHI and PII data. Detokenization combined with the automation of active policy allows for data to land in Snowflake with policy attached, meaning that the sensitive data is protected at rest, in motion, and as soon as it lands in Snowflake.
Why Detokenization?
Data Teams and InfoSec Teams alike often struggle with safeguarding the data entrusted to them in a way that is scalable and flexible to their business needs. Security teams need to restrict improper access to data as much as possible, while data teams need immediate analysis of data upon inception. Both teams need to ensure sensitive data remains safe while still being able to derive analytical and operational value from the data.
Value Add: Operational and Automated Data
ALTR’s detokenization offering operationalizes sensitive data, ensuring active policy remains attached, and automating the detokenization of that sensitive data only when necessary. Detokenization can be automated through policy, so that unmasked data is only available to the correct users at the correct time – freeing up the time of hands-on-keyboard team members and guaranteeing policy compliance. This also ensures that service accounts entering Snowflake can operate on policy-based data without gaining access to sensitive data that should remain obfuscated.
Wrapping Up
ALTR is thrilled to be the first data governance and data security solution to offer detokenization on Snowflake, and we are excited about the potential of this product offering to help Data Teams and InfoSec Teams alike derive the most value from their sensitive data at scale.
See It In Action: Automated Data Governance, Real Time Security
Let us show you:
- How we integrate with industry-leading data platforms and databases like Snowflake, Matillion and Tableau to protect your sensitive data “to the left” of your cloud data warehouse
- How you can protect data with your ETL throughout your cloud data migration
- How easy it is to automate data governance and security at scale across your enterprise

Nov 28
0
min
Why DIY Data Governance Fails Miserably
ALTR Blog
In the world of data governance, companies often feel trapped between two seemingly stark choices: the expensive, high-end solutions that can start at a staggering $100,000 or more or the DIY approach that promises cost savings but often ends in frustration and failure. The allure of going the do-it-yourself route is undeniable, but what many fail to realize is that it comes with its own set of hidden costs and challenges that can cripple even the most well-intentioned efforts. The good news is that DIY is not the only cost-effective option available. Hidden beneath this dilemma lies a powerful alternative - self-service data governance. This blog delves into the reasons why DIY data governance frequently falls short of expectations, leaving organizations in a state of confusion and vulnerability. Moreover, it highlights the transformative potential of low-code/no-code self-service data governance solutions.
The Temptation of DIY
The decision to pursue DIY data governance often stems from a desire to avoid the hefty price tags and long implementation times associated with enterprise-level solutions. Companies believe they can cut costs by employing in-house programmers to write custom code and allocating existing resources to manage data governance. At first glance, it seems like a cost-effective solution, but this path is fraught with pitfalls.
1. The Cost of Expertise
One of the most significant hidden costs of DIY data governance is the expense of hiring and retaining experienced data engineers. Customizing data governance solutions requires skilled professionals who understand the intricacies of data management, security, and compliance. These experts demand competitive salaries and can be challenging to find and retain.
2. Time-Consuming Commitments
DIY data governance projects often monopolize valuable time and resources that could be better allocated to core data-related tasks. Teams become bogged down with the intricacies of developing and maintaining custom solutions, diverting attention from strategic data initiatives.
3. Scaling Challenges
As organizations grow and accumulate more data and users, the limitations of DIY solutions become painfully apparent. Custom-built systems struggle to scale efficiently, leading to bottlenecks, performance issues, and a growing sense of frustration.
4. Governance Changes and Enforcement
Enforcing and changing data governance policies becomes increasingly complex when dealing with a DIY approach. Who is responsible for setting and editing policies? How can changes be made without disrupting the entire system? What initially appears as a straightforward change to a data governance policy can spiral into a protracted development, testing, and promotion process. These issues lead to policy enforcement gaps and an added layer of complexity that can grind data governance to a halt.
5. Forgoing Critical Decision Making
Another common pitfall is the rush to migrate data to the cloud without considering critical decisions such as security standards. Neglecting issues like adhering to NIST standards or establishing a clear permissions hierarchy can lead to security breaches and compliance violations down the line.
6. The Spectrum of Gray in Data Governance
Data governance isn't a one-size-fits-all concept, and this lack of clarity contributes to the failure of many DIY initiatives. The absence of a unified strategy can lead to confusion within organizations, with individuals not understanding the boundaries of their roles or how different teams will consume data. The result is a fragmented approach that hinders effective data governance.
Unlock the Power of Self-Service Data Governance
At the crossroads where DIY data governance meets the realm of costly enterprise solutions, a game-changing alternative emerges: self-service data governance. Within this paradigm, two formidable allies take center stage to address the challenges that DIY data governance presents—automation and low-code/no-code software. Together, these dynamic features provide transformative benefits, fundamentally reshaping how organizations approach data access and data security and alleviate the intricacies that frequently hinder DIY initiatives.
Automation takes center stage, revolutionizing the scalability of data governance. It empowers organizations to effortlessly navigate the intricate landscape of data access management, regardless of their size. As data pools expand and user demands grow, automation becomes a resource-efficient solution, preventing accidental data breaches and ensuring regulatory compliance. It supplants the labour-intensive processes associated with DIY, providing a systematic, agile approach that adapts seamlessly as an organization's data requirements evolve.
Low-code/no-code solutions further augment the arsenal against DIY challenges. These interfaces simplify the implementation of data governance by eliminating the need for extensive custom coding. No one needs to know SQL, Apache Ranger or YAML.
Low-code/no-code software expedites the automation of data security, enabling organizations to effortlessly apply granular access policies to multiple users simultaneously. This approach accelerates the deployment of governance measures and makes them accessible to a broader range of users, reducing the reliance on specialized technical expertise.
Wrapping Up
While DIY data governance may seem like an appealing alternative to costly enterprise solutions, it often leads organizations down a treacherous path filled with hidden expenses, scalability issues, and governance challenges. Luckily, self-service data governance solutions like ALTR offer a transformative path for organizations seeking to transcend the limitations and pitfalls of the DIY approach. With the ability to reduce time-value from 6 months to 60 minutes, ALTR provides an efficient and cost-effective means to streamline data governance processes, enhance data security, and maximize the value of data assets. And the best part, organizations can get started for free. Embracing tools like ALTR heralds a future where data governance becomes agile, accessible, and highly effective, ensuring that organizations thrive in an increasingly data-driven landscape.

Nov 20
0
min
Why Tomorrow is Too Late for Data Governance and Security
ALTR Blog
Data is the new oil fueling industry transformations, innovations, and revolutions. However, just as oil can cause environmental hazards if mishandled, poorly managed data can lead to catastrophic consequences for organizations. The imperative question is not if but when organizations should start implementing data governance and security. The straightforward answer is now, and this article delves into the compelling reasons behind this urgency.
The Increasing Value and Vulnerability of Data
In the past decade, data has transcended its role from being a mere byproduct of business activities to a core asset. The rise of big data analytics, artificial intelligence, and machine learning has exponentially increased the value derived from data. However, with great value comes great vulnerability. The more integral data becomes to business operations, the more attractive it becomes to malicious entities.
The Ever-Evolving Regulatory Landscape
The regulatory landscape regarding data privacy and security is constantly evolving. Regulations like the General Data Protection Regulation (GDPR) in Europe and the California Consumer Privacy Act (CCPA) in the United States have set new precedents in data protection. These regulations don't just demand compliance; they necessitate a proactive approach to data governance. Non-compliance can result in hefty fines, but beyond that, it can severely damage an organization's reputation.
The High Cost of Data Breaches
Data breaches are no longer rare occurrences but a looming threat. The cost of a data breach is not just financial; it encompasses legal repercussions, loss of customer trust, and long-term brand damage. IBM's Cost of a Data Breach Report highlights the escalating costs and impacts of data breaches. This reality underscores the need for robust data governance and security measures as foundational, not just add-on, business strategies.
Early Implementation: A Strategic Advantage
Implementing data governance and security early offers a strategic advantage. It's easier and more cost-effective to embed these practices into the organizational fabric from the start rather than retrofitting them later. Early implementation allows organizations to build a culture of data responsibility, where data is handled with the care and strategic insight it deserves.
The Role of Data in Decision-Making
Data-driven decision-making is the cornerstone of modern businesses. The integrity and reliability of data are paramount in this process. Without strong governance and security, data can be corrupted, leading to misguided decisions that could have far-reaching negative consequences.
Data Security as Customer Trust Builder
In an era where customers are increasingly aware and concerned about their data privacy, robust data governance and security can become a unique selling proposition. Demonstrating a commitment to data security can build customer trust and loyalty, which are invaluable assets in the competitive business landscape.
The Technological Imperative
Technology evolves at a breakneck pace, as do the methods to compromise it. Organizations that delay implementing data governance and security are in a perpetual game of catch-up, vulnerable to the latest threats. An early start in adopting these practices means staying ahead in this technological race.
The Human Factor
Data breaches are not always a result of sophisticated cyber-attacks; often, they stem from human error. Early implementation of data governance and security involves training and creating employee awareness, which is crucial in mitigating these risks.
The Competitive Edge
How an organization manages its data can become a competitive advantage in the data economy. Companies that excel in data governance and security are more agile, make better decisions, and are trusted more by customers and partners. This trust translates into tangible business outcomes.
Future-proofing the Business
The future is data-centric, and the businesses that thrive will be those that have mastered the art of managing and protecting their data. Implementing data governance and security is not just about addressing current needs; it's about future-proofing the business in a world where data will only grow in importance.
How to Get Started with Data Governance & Security
Embarking on the data governance and security journey can seem daunting, but it is essential and manageable with the right approach. Here are practical tips to help organizations get started:
Assess Your Organization’s Readiness
Evaluating your organization's current state is crucial before embarking on a data governance and security journey. This assessment involves reviewing existing data management practices, understanding the data lifecycle within your organization, and identifying the key stakeholders. Evaluate the technology infrastructure and its capacity to support data governance initiatives. Understand the level of data literacy among employees and identify the gaps in skills and knowledge that need to be addressed. This readiness assessment lays the groundwork for a successful data governance program by highlighting areas that require immediate attention and improvement.
Define Data Governance Objectives and Scope
The next step is clearly articulating what you aim to achieve through data governance. Objectives can range from ensuring regulatory compliance and improving data quality to enabling better decision-making. Once objectives are set, define the scope of your data governance program. Decide which data assets will be governed, who will be involved, and the boundaries of the initiative. This step ensures that the data governance and security program is aligned with the organization's strategic goals and has a clear direction.
Establish a Data Governance Framework
Creating a robust data governance framework is vital. This framework should encompass policies, standards, procedures, and data management guidelines. It also involves defining roles and responsibilities around data, such as data owners, stewards, and custodians. The framework should be flexible yet comprehensive, accommodating changes in business strategies, technologies, and regulations. Effective governance frameworks are often iterative, evolving as the organization grows and learns.
Implement Data Quality Management
High data quality is essential for reliable analytics and decision-making. Start by defining data quality for your organization, including accuracy, completeness, consistency, and timeliness. Develop processes for ongoing data quality assessment, including data cleansing, validation, and remediation methods. Establishing a mechanism for continuous data quality monitoring and improvement is also essential. This ongoing commitment to maintaining high data quality is a cornerstone of effective data governance.
Establish Data Security and Privacy Controls
Establishing robust data security and privacy controls is non-negotiable in the era of increasing cyber threats and stringent data protection laws. This involves deploying technological solutions like access controls and tokenization and formulating policies and procedures that ensure data is handled securely and ethically. Regular training and employee awareness programs about data security best practices are also crucial. Ensuring compliance with relevant data protection laws like GDPR or CCPA should be integral to your data governance program.
Define Data Governance Metrics
To measure the effectiveness of your data governance program, it's essential to define relevant metrics and KPIs. These could include data quality measures, compliance levels, the efficiency of data management processes, or the impact of data governance on decision-making. Regularly tracking these metrics will help assess the program's performance and identify areas for improvement. It also helps demonstrate the value of data governance to stakeholders and secure ongoing support.
Continuously Evolve and Improve
Data governance is not a one-time project but a continuous journey. As your organization evolves, so should your data governance program. This means regularly revisiting and revising the governance framework, staying updated with emerging technologies, cybersecurity threats and regulatory changes, and continually seeking stakeholder feedback. Foster a culture of continuous improvement where learning and adapting are part of the organization's approach to data governance.
Wrapping Up
The question of when to implement data governance and data security has a simple yet profound answer: the time is now. In an increasingly data-driven world, these practices are not just safeguards but fundamental to sustainable business growth and success. Organizations that recognize and act on this imperative will navigate the future with confidence and resilience, turning their data into a wellspring of opportunities rather than a source of constant threats. Therefore, the call to action is clear and immediate: prioritize data governance and security today, for it is the cornerstone upon which the successful organizations of tomorrow will be built.
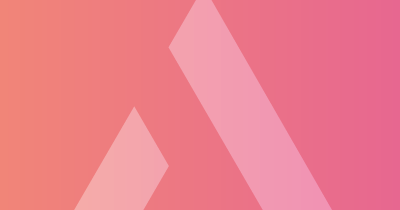
Nov 15
0
min
What is Data De-identification & Why Is It Necessary?
ALTR Blog
The sheer volume of data generated daily has unlocked a wealth of opportunities for research, innovation, and business growth. The insights and knowledge that businesses can glean from this data are invaluable. However, all of this information also brings a pressing concern—privacy. As data flows ceaselessly across digital channels, it becomes increasingly challenging to safeguard individuals' sensitive information. So, how can we harness the immense power of data analytics while ensuring that personal privacy remains inviolable? Enter data de-identification, a process that serves as the bridge between data-driven insights and privacy protection.
What is Data De-identification?
Data de-identification is a form of dynamic data masking where personally identifiable information (PII) is removed from datasets to safeguard privacy. PII includes any data that can be used to directly or indirectly identify individuals, such as names, addresses, social security numbers, etc.
Why is De-identification Necessary?
Privacy Protection
Foremost, data de-identification serves as an indispensable guardian of individuals' privacy. In an age where personal data is collected, processed, and shared unprecedentedly, the risk of privacy infringements looms large. De-identification acts as a shield, allowing organizations to unlock the potential of data while ensuring that sensitive, personally identifiable information (PII) remains concealed. It safeguards individuals from identity theft, unauthorized access, and misuse of their personal details.
Data Sharing and Collaboration
De-identification paves the way for seamless data sharing and collaboration within and between organizations. Researchers, businesses, and institutions often need to exchange data to drive innovation, conduct studies, and make informed decisions. By removing or altering PII, de-identification allows these entities to share data without violating privacy regulations or risking individuals' sensitive information, fostering a collaborative ecosystem where insights can be pooled, and collective knowledge can flourish.
Unlocking Data's Potential
Data harbors the immense potential for valuable insights and discoveries in its raw and unprocessed form. However, this potential often remains untapped due to privacy concerns. Data de-identification bridges this gap by allowing organizations to harness the power of data analytics and research without compromising individual privacy. It empowers businesses to improve products and services, researchers to advance scientific understanding, and policymakers to make informed decisions based on data-driven evidence.
Regulatory Compliance
The regulatory landscape surrounding data privacy is becoming increasingly stringent. Regulations like the General Data Protection Regulation (GDPR) in Europe and the Health Insurance Portability and Accountability Act (HIPAA) in the United States mandate the protection of individuals' personal data. Failure to comply with these regulations can result in severe penalties. De-identification is fundamental for organizations to ensure they align with these legal requirements while benefiting from data usage.
Ethical Data Handling
Beyond legal obligations, de-identification aligns with ethical data handling practices. It demonstrates an organization's commitment to responsible and ethical data stewardship, fostering trust among data subjects and stakeholders. By implementing de-identification measures, organizations are dedicated to striking a fair and honest balance between data's utility and personal privacy preservation.
How to De-Identify Data
Data de-identification typically involves a two-step approach:
Step 1: Data Classification
The first step in data de-identification is to classify and tag the data according to its sensitivity and regulatory requirements and to identify direct and indirect identifiers within the dataset. Here's a breakdown of these identifiers:
Direct Identifiers are unique data elements that can directly point to an individual. Examples include Social Security numbers, passport numbers, and taxpayer identification numbers. These identifiers pose a high risk to privacy and require careful handling.
Indirect Identifiers consist of personal attributes that, on their own, are not unique to any particular individual. Examples include height, ethnicity, hair color, and more. While they may not individually identify someone, combining multiple indirect identifiers can reveal an individual's identity. Managing and protecting indirect identifiers is essential to prevent re-identification.
Automated data classification is often used to assist in this process. These tools can recognize and label direct and indirect identifiers, making the de-identification process more efficient and reducing the risk of human error.
Step 2: Data Masking
Once the data has been classified and tagged, it must be masked. Data masking involves concealing or altering parts of data to protect sensitive information while maintaining data utility. Here are some critical data masking techniques:
Tokenization: Tokenization replaces sensitive data with unique tokens or identifiers that have no intrinsic meaning but can be used consistently throughout the dataset. Tokenized data can be reversed only by those with access to the tokenization key.
Partial Redaction: Sensitive information, such as specific names or identification numbers, can be partially redacted, replacing some characters with placeholders or generic labels. For example, "John Smith" might become "J**** S****."
Generalization: Generalization involves replacing precise values with broader categories or ranges. For instance, age groups could replace exact ages (e.g., "25-34").
Substitution: Sensitive data can be substituted with fictitious or generic data while preserving the data's overall structure and statistical properties. For example, actual names can be replaced with familiar names like "User 1" or "Customer A."
Applications of Data De-Identification
De-identification plays a critical role in various domains:
- Healthcare: De-identified medical records allow researchers to study disease patterns, treatment outcomes, and public health trends while safeguarding patient privacy.
- Finance: Financial institutions use de-identification to protect customer data when conducting fraud detection and risk assessment analyses.
- Research: Scientists can share datasets for collaborative research while complying with ethical guidelines and privacy regulations.
- Marketing and Analytics: Companies can analyze customer data without compromising individual privacy, helping to improve products and services.
- Government: De-identification enables agencies to share sensitive data for policy analysis while complying with data protection laws.
Wrapping Up
Data de-identification is a vital tool in the age of data privacy concerns. It allows organizations to harness the power of data for research, analytics, and business growth while protecting individuals' privacy. However, it's crucial to approach de-identification cautiously, understanding its limitations and staying compliant with relevant laws and regulations. As technology advances and privacy concerns persist, the role of de-identification will only become more significant in our data-driven society.
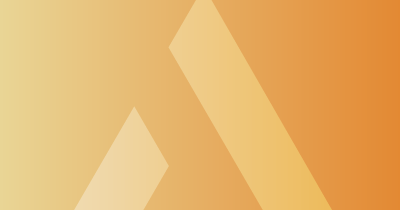
Nov 1
0
min
AI and LLM Data Security: Strategies for Balancing Innovation and Data Protection
ALTR Blog
The rapid ascent of artificial intelligence (AI) and large learning models (LLMs) has transformed the business landscape, ushering in a new era of innovation and efficiency. However, this meteoric rise has also brought about significant concerns surrounding the use and protection of sensitive data. This blog will explore how companies can strike a delicate balance between harnessing the power of AI and LLMs while safeguarding the sensitive data entrusted to them.
Understanding the Challenges
1. 'The Intern Problem'
Imagine Sarah, an eager HR intern tasked with analyzing trends in employee engagement and providing recommendations for improving HR processes. She uses the company's internal LLM-powered chatbot as part of her research. While she only needs access to employee surveys and general HR reports, Sarah experiences unrestricted access to the extensive HR database, which includes employee records, payroll information, performance reviews, and confidential HR communications.
This scenario represents a genuine concern faced by organizations utilizing AI and LLMs. Without stringent data protection, there's a heightened risk of data breaches that can result in financial losses, regulatory fines, and severe damage to the organization's reputation.
2. 'The Samsung Problem'
Samsung banned ChatGPT in April after engineers passed sensitive data to the LLM, including source code from a semiconductor database and minutes from an internal meeting. Studies have even suggested that as much as 4% of employees may inadvertently input sensitive data into LLMs.
This highlights the dangerous risk of insider threats within organizations, where trusted personnel can exploit AI and LLM tools for unauthorized data sharing (maliciously or not), potentially resulting in intellectual property theft, corporate espionage, and significant damage to an organization's reputation.
The Growing Concern and Immediate Responses
Companies are not taking these challenges lightly. They've initiated significant measures to thwart data leaks. These actions include outright bans on using LLMs by employees, adopting basic controls provided by generative AI providers, and leveraging various data security services, such as content scanning and LLM firewalls.
Unfortunately, the immediate future indicates that the data security problem will only intensify. When prompted effectively, LLMs are adept at extracting valuable data from training data. This poses a unique set of challenges that require modern technical solutions.
The Road to Equilibrium: Strategies for Balancing Innovation and Data Protection
Data Governance Frameworks
Establishing comprehensive data governance frameworks is paramount in balancing the potential of LLMs and AI technologies with robust data protection. These frameworks serve as the foundational blueprint, delineating policies, procedures, and roles for meticulous management, access control, and data protection. With these straightforward guidelines, organizations ensure that data is consistently and securely handled throughout the entire data lifecycle, from collection to disposal.
Data Classification
Not all data is created equal, and a one-size-fits-all approach to protection falls short of the mark, particularly in the context of LLMs and AI. Implementing robust data classification systems becomes pivotal, categorizing data based on its inherent sensitivity. For example, susceptible data, such as personally identifiable information (PII) or proprietary research findings, demands the highest level of protection. In contrast, less sensitive data, like publicly available information, requires less stringent safeguards. In the world of LLMs, this tailored approach ensures that sensitive data is always shielded.
Access Controls
As the conversation revolves around LLMs, implementing precise access controls becomes a linchpin in maintaining equilibrium. Organizations must strategically determine who can access what data and under what conditions. Here, the principle of least privilege takes center stage, advocating for granting individuals the minimum level of access required to fulfill their tasks. With this strategy, organizations can ensure that only authorized personnel can engage with sensitive data when utilizing LLMs, significantly reducing the risk of unauthorized access, data breaches, or privacy violations.
Encryption and Anonymization
In the age of LLMs and AI, data security extends to rendering sensitive information impervious to unauthorized access. Encryption and anonymization techniques become indispensable tools in this endeavor. By encrypting data, organizations transform it into indecipherable code, even if it falls into the wrong hands. Anonymization further safeguards data by removing personally identifiable information, making it impossible to trace back to individuals. In the context of LLMs, these techniques ensure that sensitive data remains confidential, even during accidental exposure.
Regular Audits and Monitoring
Maintaining equilibrium necessitates continuous vigilance, mainly when LLMs are in play. Organizations must consistently monitor data access and usage patterns. Real-time alerts and regular audits serve as sentinel mechanisms, promptly identifying any anomalies or suspicious activities that may signal unauthorized access or misuse of data. This proactive approach allows organizations to respond swiftly to potential security threats and reinforces the integrity of data protection measures.
Employee Training
Instead of completely blocking LLM chatbots, incorporating employees with proper education and training is paramount. Ensuring that employees understand the importance of data security and their role in safeguarding sensitive information fosters a culture of security awareness and responsibility within the organization. Specifically tailored to LLMs, training programs can highlight the unique challenges and best practices associated with utilizing these advanced language models, empowering employees to make informed decisions that uphold data protection standards.
Vendor and Partner Due Diligence
Data protection extends beyond an organization's internal operations in an interconnected world. It encompasses third-party vendors and partners who handle organizational data. Extensive due diligence becomes imperative in assessing these external entities' security and compliance standards. Ensuring that they adhere to stringent data protection measures reinforces the organization's commitment to safeguarding data, even when it leaves its direct control.
Regulatory Compliance
Finally, organizations must remain well-informed and adaptive as regulations evolve in response to the digital age. Staying attuned to the ever-changing data protection landscape, especially concerning LLMs and AI, is essential. Strict adherence to emerging data protection regulations mitigates legal risks and strengthens customer trust. It demonstrates an organization's commitment to responsible data handling, bolstering its reputation in an era where data privacy is paramount.
Wrapping Up
As we move further into the era of data-driven decision-making, the ability to balance innovation with data security will be a defining factor in the success of organizations. Those who master this delicate equilibrium will safeguard their reputation and confidently drive business growth and innovation in an increasingly data-centric world.

Oct 25
0
min
10 Terrifying Consequences of Uncontrolled Data Access
ALTR Blog
As the year's spookiest season approaches, it's time to delve into a topic that can send chills down the spines of data security and governance professionals—uncontrolled data access. This blog will explore the hair-raising reasons why lack of data access control is a real "boo" for businesses.
The Haunting Specter of Data Breaches
Data breaches are the stuff of nightmares for organizations. When sensitive data access is left unrestricted, it's like leaving the front door wide open for malicious entities to sneak in. These breaches can expose a treasure trove of sensitive information, including customer data, financial records, and intellectual property, to unauthorized parties.
The consequences of a data breach can be devastating. Financial losses mount quickly due to potential fines and the expenses associated with investigating the breach, notifying affected parties, and implementing security measures to prevent future breaches. Moreover, the reputational damage can linger like a ghost, scaring away customers and partners for years to come.
The Curse of Non-Compliance
The specter of compliance regulations like GDPR haunts every organization dealing with data. Their primary aim is to protect the personal data of individuals and give them more control over how their information is collected, processed, and stored. However, unrestricted data access can lead organizations down the frightful alley to non-compliance, inviting the wrath of regulatory bodies. GDPR fines can be substantial, reaching up to 4% of global annual turnover, a chilling prospect for any organization.
Compliance requires careful data access control and robust data governance practices. This includes defining who can access what data, monitoring data access and usage, and implementing mechanisms to prevent unauthorized access or data breaches. GDPR also mandates data protection impact assessments (DPIAs), which involve identifying and mitigating risks associated with data processing activities. Unrestricted data access makes it nearly impossible to conduct DPIAs effectively, as data is not adequately controlled or monitored.
The Ghostly Stain on Reputation
Data breaches or unauthorized access can seriously damage an organization's reputation. When news of a breach hits the headlines, customers and partners may lose trust and confidence in the organization's ability to protect sensitive information. Negative publicity can linger, haunting an organization long after the breach. Rebuilding a damaged reputation is challenging and lengthy, often requiring substantial investments in public relations and customer outreach.
The Operational Nightmare Unleashed
Data breaches can disrupt normal business operations. The immediate aftermath of a breach requires organizations to allocate resources and attention to investigate the incident, mitigate damage, and implement security measures. This diversion of resources can disrupt core business activities, causing operational nightmares. Moreover, the long-term impact on operational efficiency can be significant, as organizations may need to implement more stringent security measures and protocols.
The Rival's Unholy Grail
Competitors or malicious actors gaining access to sensitive information can exploit it for their benefit, potentially undermining an organization's competitive advantage. Losing valuable data to adversaries can be a business horror story, as competitors can use the stolen information to gain insights, develop similar products or services, or undercut the organization in the market. Protecting sensitive data is essential to maintaining a competitive edge in today's business landscape.
The Employee Morale Phantom
Employees are crucial assets to any organization, and trust is essential for a harmonious workplace. Trust can erode when employees perceive a lack of commitment to protecting their personal data or company secrets. A breach or unauthorized access incident can leave employees feeling vulnerable and uncertain about their organization's ability to safeguard their information. Decreased morale and potential talent attrition can haunt the workplace and impact an organization's productivity and culture.
The Silver Dagger Against Data Security Demons
While the challenges of uncontrolled data access may send shivers down your spine, automated data access control is a potent weapon that can help ward off these data security demons.
Fortifying Digital Fortresses:
Automated data access control tools act as vigilant sentinels guarding your digital fortresses. They monitor data access in real-time and trigger immediate alerts at the first sign of suspicious activity and shutting down access or initiating security protocols to neutralize the danger.
Role-Based Access:
With automated controls, you can establish role-based access policies. This means that employees, contractors, and other stakeholders are granted precisely the level of access necessary for their roles.
Time-Limited Permissions:
Automated systems allow you to set time-limited or rate-limited permissions. This is like giving a ghostly visitor a temporary pass to access your house. Once the time is up, or once they exceed a set level of access, their access is revoked automatically. This feature is invaluable for contractors, temporary employees, or projects that require specific access for a limited period.
Audit Trails:
Like leaving a trail of breadcrumbs through a haunted forest, automated access control systems maintain detailed audit trails—these logs record who accessed what data, when, and what actions they performed. In the event of a breach or incident, these logs serve as invaluable clues to investigate and contain the threat.
Adaptive Authentication:
Just as you'd demand proof of identity from a suspicious character at your doorstep, automated systems employ adaptive authentication. They assess the risk associated with each access attempt, requiring additional verification steps for high-risk activities. This adds an extra layer of security to your data.
Compliance Assurance:
Automated controls can be configured to ensure compliance with data protection regulations like GDPR or HIPAA. They enforce access policies consistently, reducing the risk of privacy violations that can result in hefty fines.
Scalability and Efficiency:
Automated systems scale with your organization, accommodating growth seamlessly. Whether you're a fledgling start-up or an enterprise, these controls efficiently manage access without overburdening data and security teams.
Wrapping Up
While Halloween may be a time for embracing the spooky, the horrors of uncontrolled data access are real for organizations. Data breaches, compliance nightmares, and security threats are chilling realities that can cripple businesses. As you prepare for Halloween, remember to exorcise the demons of unauthorized data access and embrace data security and governance. Stay safe, and have a spooktacular Halloween!
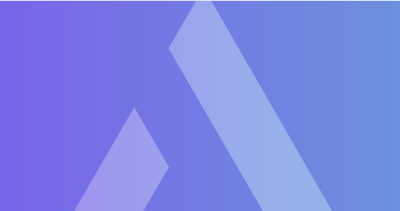
Oct 20
0
min
The Crucial Collaboration Between CISOs and CDOs in Data Governance and Security
ALTR Blog
In the ever-evolving data management landscape, two key roles are emerging as linchpins in the quest for data-driven success: Chief Information Security Officers (CISOs) and Chief Data Officers (CDOs). These two professionals find themselves at the intersection of data governance and security, holding distinct responsibilities that are pivotal to an organization's prosperity. CISOs are the guardians of data security, tirelessly working to identify and mitigate risks associated with data breaches and cyber threats. On the other hand, CDOs are the custodians of data quality, ensuring accuracy and consistency for meaningful insights. Though their missions may seem parallel, their approaches often diverge, leading to friction and missed opportunities. This blog explores the compelling case for the collaboration between CISOs and CDOs, highlighting how their joint efforts can fortify data governance and security practices and propel organizations toward data-driven excellence.
The CISO's Battle for Data Security
CISOs are the unsung guardians of an organization's digital fortress. Their primary duty revolves around identifying, mitigating, and managing risks associated with data security. In an era where cyber threats loom like dark clouds, the CISO is the stalwart defender, shielding sensitive information from the relentless onslaught of hackers and data breaches.
The CISO's mission is clear: to ensure that an organization's data remains impervious to external threats, unauthorized access, and data misuse. This responsibility demands constant vigilance, a comprehensive understanding of evolving cyber threats, and a proactive approach to security measures. But herein lies the paradox: the more stringent the security, the more challenging it becomes to provide seamless access to data, often causing friction with the CDO.
The CDO's Quest for Data Excellence
On the flip side, the Chief Data Officer is the architect of data excellence. CDOs are charged with the responsibility of ensuring that data is not only secure but also accurate, reliable, and accessible. In their world, data is not just a fortress to be protected but a valuable resource to be harnessed for strategic advantage.
CDOs seek real-time data access, for they understand that the competitive edge often hinges on swiftly responding to market dynamics and consumer preferences. The CDO's realm is data quality, consistency, and usability. However, this zeal for accessibility can sometimes clash with the CISO's imperative for security, setting the stage for an interdepartmental tug-of-war.
The Clash of Objectives
At first glance, CISOs and CDOs may share common ground, aiming to extract business value from data. However, their divergent strategies and priorities can create a disconnect that hinders progress and exposes organizations to vulnerabilities.
Consider a scenario where a CISO, committed to safeguarding data, enforces strict access controls and encryption protocols. While this is undoubtedly essential for security, it can inadvertently impede the CDO's quest for real-time data access. This misalignment of objectives can manifest as delays in data availability, negatively impacting decision-making and the organization's ability to adapt swiftly.
Conversely, when the CDO prioritizes rapid data access without adequate security measures, it risks exposing the organization to cyber threats and regulatory non-compliance. This approach can have dire consequences in an era of stringent data protection regulations, including hefty fines and reputational damage.
Speaking the Same Language
The solution to this conundrum lies in the collaboration between CISOs and CDOs. These roles must transcend their departmental silos and speak the same language. The key is establishing a framework where data governance and security practices complement each other rather than collide.
Shared Risk Awareness
CISOs and CDOs must develop a shared understanding of the risks associated with data. This involves open and ongoing communication to identify potential threats, vulnerabilities, and the consequences of data breaches. By aligning their risk assessments, they can make informed decisions that balance data security with accessibility.
Data Ownership and Accountability
Defining clear ownership and accountability for data is paramount. CISOs should lead in defining data classification and access controls, while CDOs can focus on setting data quality standards and usability guidelines. This cooperative approach guarantees that security measures do not impede data usability and that data quality remains upheld.
Unified Technology Platform
Organizations can streamline data governance and security processes by leveraging unified technology platforms. These platforms can provide a centralized view of data, allowing CISOs to enforce security measures seamlessly and giving CDOs the tools they need to maintain data quality and accessibility. Unified technology fosters efficiency and ensures that both roles have the necessary resources to achieve their goals, making the collaboration more effective.
Education and Awareness
Both CISOs and CDOs should invest in educating their teams and the broader organization about the importance of data security and governance. This fosters a culture of data responsibility and ensures all stakeholders align with the organization's objectives.
Continuous Evaluation and Adaptation
The landscape of data security and governance is ever-evolving. CISOs and CDOs must regularly reassess their strategies and adapt to emerging threats and opportunities. Collaboration allows for agility in responding to changing circumstances.
The Benefits of Collaboration
When CISOs and CDOs collaborate effectively, organizations reap numerous benefits. First and foremost, data becomes a trusted asset that is both secure and readily available. This fosters a culture of real-time data-driven decision-making, enhancing an organization's competitive edge.
Moreover, collaboration between these two critical roles minimizes the risk of data breaches and regulatory violations. By aligning their strategies, organizations can confidently navigate the complex landscape of data protection laws, avoiding costly penalties and preserving their reputation.
Innovation also thrives in an environment where data governance and security are harmonious. When data is both secure and accessible, it becomes a powerful catalyst for innovation, enabling organizations to explore new avenues and seize opportunities.
Wrapping Up
Data is the lifeblood of organizations. Its security and quality are non-negotiable, yet so is its accessibility for driving business value. Ultimately, it's not a matter of choosing between security and accessibility; it's about achieving both. When CISOs and CDOs speak the same language and work together, organizations can unlock the full potential of their data while fortifying their defenses against an ever-present array of threats. It's time to bridge the gap and unite forces for the sake of data and the future of business.
Get the latest from ALTR
Subscribe below to stay up to date with our team, upcoming events, new feature releases, and more.
Thank you! Your submission has been received!
Oops! Something went wrong while submitting the form.