BLOG SPOTLIGHT
ALTR’s Format-Preserving Encryption, powered by FF3-1 algorithm and ALTR’s trusted policies, offers a comprehensive solution for securing sensitive data.
Read more
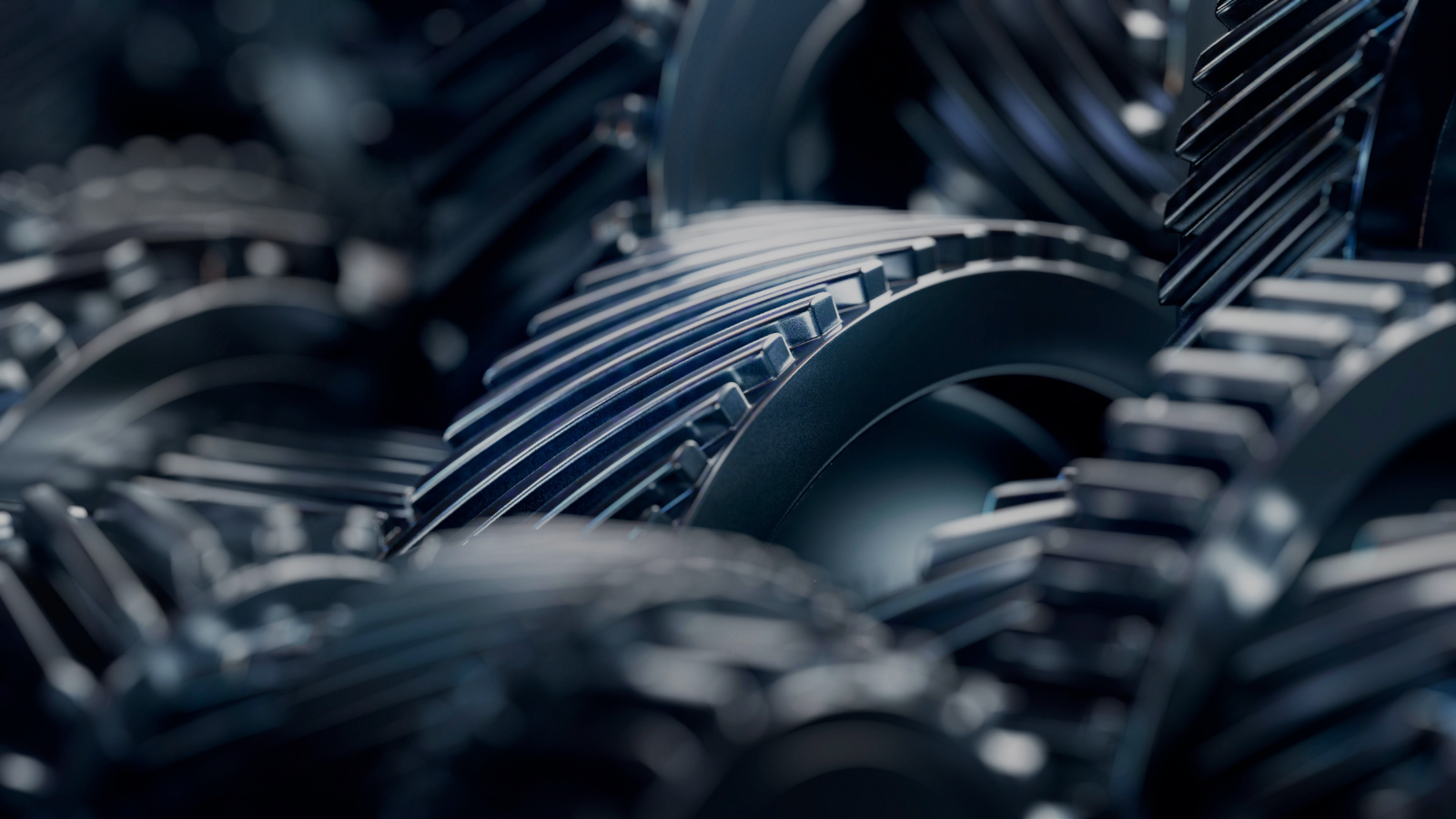
Popular
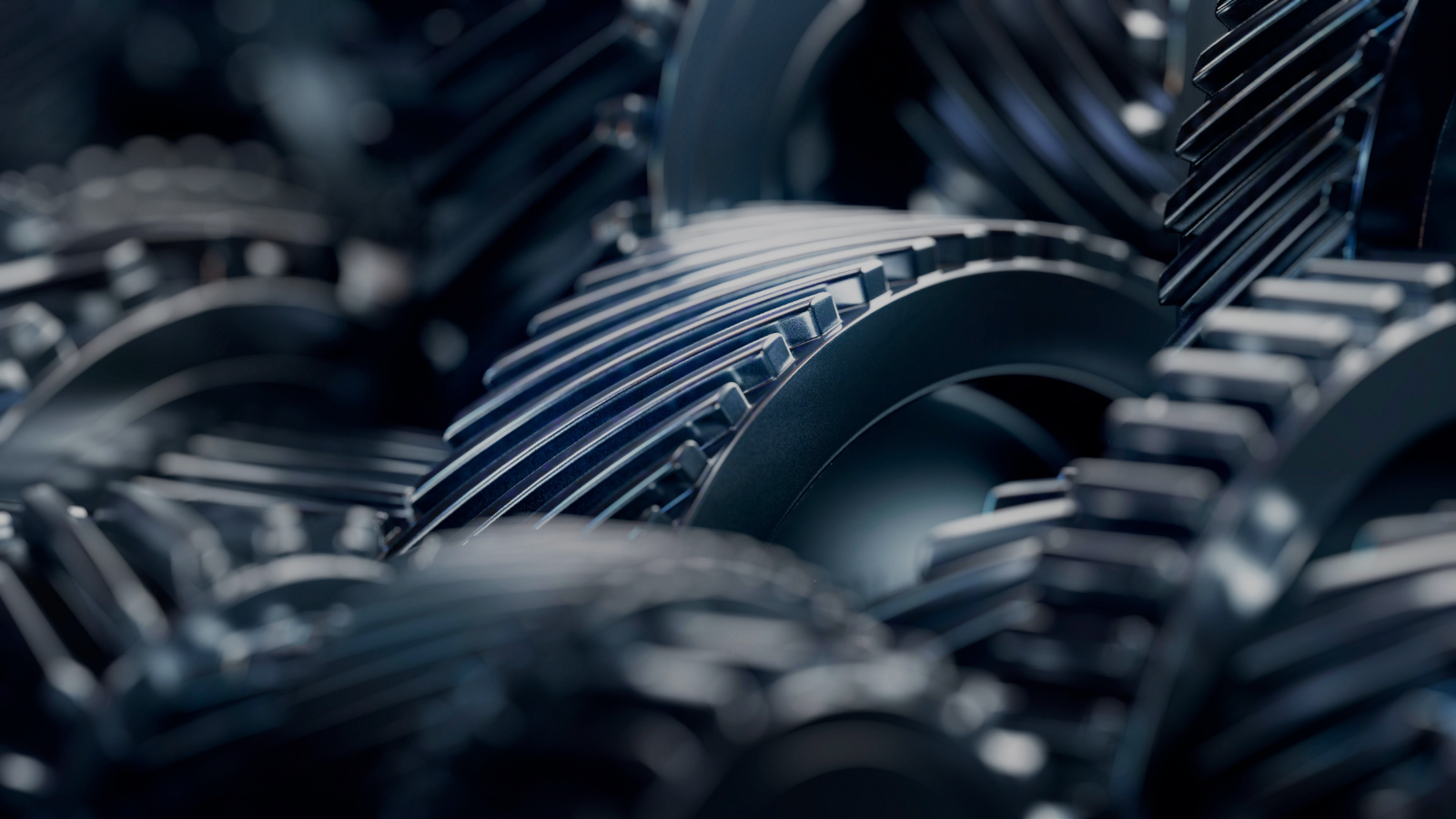
Oct 30
0
min
Format-Preserving Encryption: A Deep Dive into FF3-1 Encryption Algorithm
In the ever-evolving landscape of data security, protecting sensitive information while maintaining its usability is crucial. ALTR’s Format Preserving Encryption (FPE) is an industry disrupting solution designed to address this need. FPE ensures that encrypted data retains the same format as the original plaintext, which is vital for maintaining compatibility with existing systems and applications. This post explores ALTR's FPE, the technical details of the FF3-1 encryption algorithm, and the benefits and challenges associated with using padding in FPE.
What is Format Preserving Encryption?
Format Preserving Encryption is a cryptographic technique that encrypts data while preserving its original format. This means that if the plaintext data is a 16-digit credit card number, the ciphertext will also be a 16-digit number. This property is essential for systems where data format consistency is critical, such as databases, legacy applications, and regulatory compliance scenarios.
Technical Overview of the FF3-1 Encryption Algorithm
The FF3-1 encryption algorithm is a format-preserving encryption method that follows the guidelines established by the National Institute of Standards and Technology (NIST). It is part of the NIST Special Publication 800-38G and is a variant of the Feistel network, which is widely used in various cryptographic applications. Here’s a technical breakdown of how FF3-1 works:
Structure of FF3-1
1. Feistel Network: FF3-1 is based on a Feistel network, a symmetric structure used in many block cipher designs. A Feistel network divides the plaintext into two halves and processes them through multiple rounds of encryption, using a subkey derived from the main key in each round.
2. Rounds: FF3-1 typically uses 8 rounds of encryption, where each round applies a round function to one half of the data and then combines it with the other half using an XOR operation. This process is repeated, alternating between the halves.
3. Key Scheduling: FF3-1 uses a key scheduling algorithm to generate a series of subkeys from the main encryption key. These subkeys are used in each round of the Feistel network to ensure security.
4. Tweakable Block Cipher: FF3-1 includes a tweakable block cipher mechanism, where a tweak (an additional input parameter) is used along with the key to add an extra layer of security. This makes it resistant to certain types of cryptographic attacks.
5. Format Preservation: The algorithm ensures that the ciphertext retains the same format as the plaintext. For example, if the input is a numeric string like a phone number, the output will also be a numeric string of the same length, also appearing like a phone number.
How FF3-1 Works
1. Initialization: The plaintext is divided into two halves, and an initial tweak is applied. The tweak is often derived from additional data, such as the position of the data within a larger dataset, to ensure uniqueness.
2. Round Function: In each round, the round function takes one half of the data and a subkey as inputs. The round function typically includes modular addition, bitwise operations, and table lookups to produce a pseudorandom output.
3. Combining Halves: The output of the round function is XORed with the other half of the data. The halves are then swapped, and the process repeats for the specified number of rounds.
4. Finalization: After the final round, the halves are recombined to form the final ciphertext, which maintains the same format as the original plaintext.
Benefits of Format Preserving Encryption
Implementing FPE provides numerous benefits to organizations:
1. Compatibility with Existing Systems: Since FPE maintains the original data format, it can be integrated into existing systems without requiring significant changes. This reduces the risk of errors and system disruptions.
2. Improved Performance: FPE algorithms like FF3-1 are designed to be efficient, ensuring minimal impact on system performance. This is crucial for applications where speed and responsiveness are critical.
3. Simplified Data Migration: FPE allows for the secure migration of data between systems while preserving its format, simplifying the process and ensuring compatibility and functionality.
4. Enhanced Data Security: By encrypting sensitive data, FPE protects it from unauthorized access, reducing the risk of data breaches and ensuring compliance with data protection regulations.
5. Creation of production-like data for lower trust environments: Using a product like ALTR’s FPE, data engineers can use the cipher-text of production data to create useful mock datasets for consumption by developers in lower-trust development and test environments.
Security Challenges and Benefits of Using Padding in FPE
Padding is a technique used in encryption to ensure that the plaintext data meets the required minimum length for the encryption algorithm. While padding is beneficial in maintaining data structure, it presents both advantages and challenges in the context of FPE:
Benefits of Padding
1. Consistency in Data Length: Padding ensures that the data conforms to the required minimum length, which is necessary for the encryption algorithm to function correctly.
2. Preservation of Data Format: Padding helps maintain the original data format, which is crucial for systems that rely on specific data structures.
3. Enhanced Security: By adding extra data, padding can make it more difficult for attackers to infer information about the original data from the ciphertext.
Security Challenges of Padding
1. Increased Complexity: The use of padding adds complexity to the encryption and decryption processes, which can increase the risk of implementation errors.
2. Potential Information Leakage: If not implemented correctly, padding schemes can potentially leak information about the original data, compromising security.
3. Handling of Padding in Decryption: Ensuring that the padding is correctly handled during decryption is crucial to avoid errors and data corruption.
Wrapping Up
ALTR's Format Preserving Encryption, powered by the technically robust FF3-1 algorithm and married with legendary ALTR policy, offers a comprehensive solution for encrypting sensitive data while maintaining its usability and format. This approach ensures compatibility with existing systems, enhances data security, and supports regulatory compliance. However, the use of padding in FPE, while beneficial in preserving data structure, introduces additional complexity and potential security challenges that must be carefully managed. By leveraging ALTR’s FPE, organizations can effectively protect their sensitive data without sacrificing functionality or performance.
For more information about ALTR’s Format Preserving Encryption and other data security solutions, visit the ALTR documentation
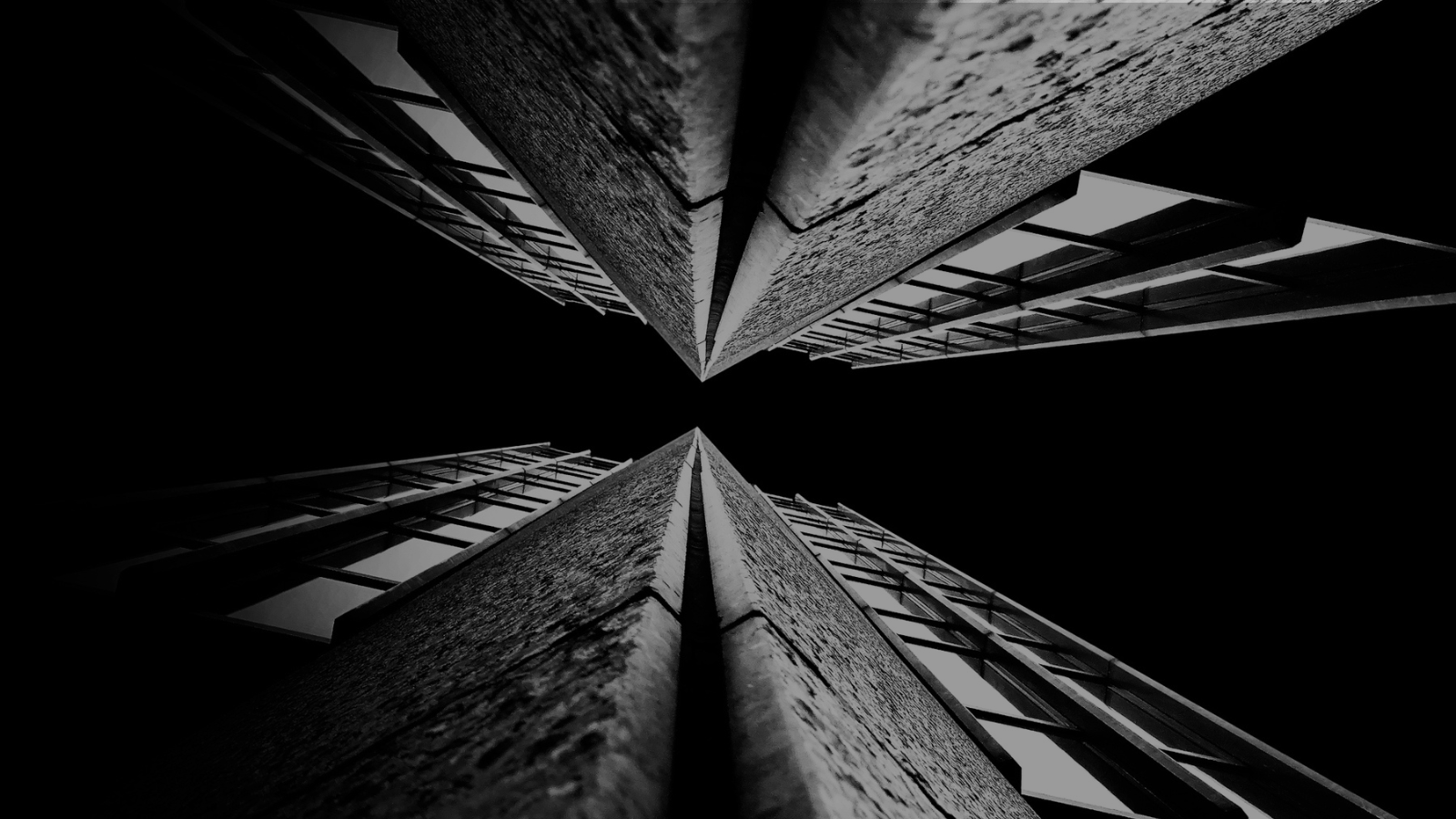
Oct 22
0
min
The CISO's Dilemma: Securing Snowflake's ACCOUNTADMIN Role
For years (even decades) sensitive information has lived in transactional and analytical databases in the data center. Firewalls, VPNs, Database Activity Monitors, Encryption solutions, Access Control solutions, Privileged Access Management and Data Loss Prevention tools were all purchased and assembled to sit in front of, and around, the databases housing this sensitive information.
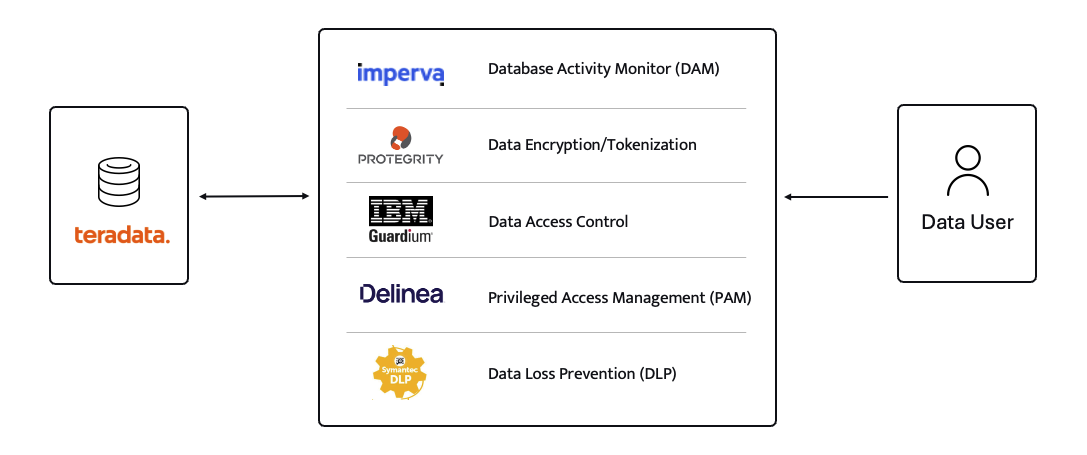
Even with all of the above solutions in place, CISO’s and security teams were still a nervous wreck. The goal of delivering data to the business was met, but that does not mean the teams were happy with their solutions. But we got by.
The advent of Big Data and now Generative AI are causing businesses to come to terms with the limitations of these on-prem analytical data stores. It’s hard to scale these systems when the compute and storage are tightly coupled. Sharing data with trusted parties outside the walls of the data center securely is clunky at best, downright dangerous in most cases. And forget running your own GenAI models in your datacenter unless you can outbid Larry, Sam, Satya, and Elon at the Nvidia store. These limits have brought on the era of cloud data platforms. These cloud platforms address the business needs and operational challenges, but they also present whole new security and compliance challenges.
ALTR’s platform has been purpose-built to recreate and enhance these protections required to use Teradata for Snowflake. Our cutting-edge SaaS architecture is revolutionizing data migrations from Teradata to Snowflake, making it seamless for organizations of all sizes, across industries, to unlock the full potential of their data.
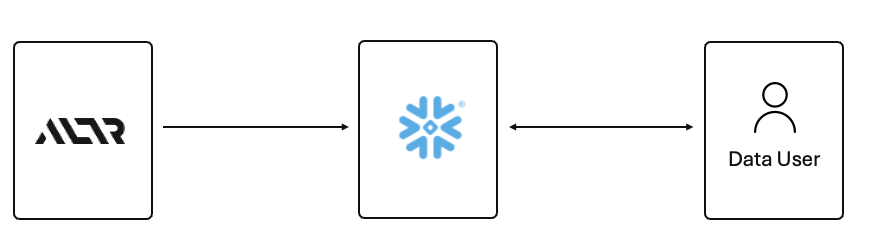
What spurred this blog is that a company reached out to ALTR to help them with data security on Snowflake. Cool! A member of the Data & Analytics team who tried our product and found love at first sight. The features were exactly what was needed to control access to sensitive data. Our Format-Preserving Encryption sets the standard for securing data at rest, offering unmatched protection with pricing that's accessible for businesses of any size. Win-win, which is the way it should be.
Our team collaborated closely with this person on use cases, identifying time and cost savings, and mapping out a plan to prove the solution’s value to their organization. Typically, we engage with the CISO at this stage, and those conversations are highly successful. However, this was not the case this time. The CISO did not want to meet with our team and practically stalled our progress.
The CISO’s point of view was that ALTR’s security solution could be completely disabled, removed, and would not be helpful in the case of a compromised ACCOUNTADMIN account in Snowflake. I agree with the CISO, all of those things are possible. Here is what I wanted to say to the CISO if they had given me the chance to meet with them!
The ACCOUNTADMIN role has a very simple definition, yet powerful and long-reaching implications of its use:

One of the main points I would have liked to make to the CISO is that as a user of Snowflake, their responsibility to secure that ACCOUNTADMIN role is squarely in their court. By now I’m sure you have all seen the news and responses to the Snowflake compromised accounts that happened earlier this year. It is proven that unsecured accounts by Snowflake customers caused the data theft. There have been dozens of articles and recommendations on how to secure your accounts with Snowflake and even a mandate of minimum authentication standards going forward for Snowflake accounts. You can read more information here, around securing the ACCOUNTADMIN role in Snowflake.
I felt the CISO was missing the point of the ALTR solution, and I wanted the chance to explain my perspective.
ALTR is not meant to secure the ACCOUNTADMIN account in Snowflake. That’s not where the real risk lies when using Snowflake (and yes, I know—“tell that to Ticketmaster.” Well, I did. Check out my write-up on how ALTR could have mitigated or even reduced the data theft, even with compromised accounts). The risk to data in Snowflake comes from all the OTHER accounts that are created and given access to data.
The ACCOUNTADMIN role is limited to one or two people in an organization. These are trusted folks who are smart and don’t want to get in trouble (99% of the time). On the other hand, you will have potentially thousands of non-ACCOUNTADMIN users accessing data, sharing data, screensharing dashboards, re-using passwords, etc. This is the purpose of ALTR’s Data Security Platform, to help you get a handle on part of the problem which is so large it can cause companies to abandon the benefits of Snowflake entirely.
There are three major issues outside of the ACCOUNTADMIN role that companies have to address when using Snowflake:
1. You must understand where your sensitive is inside of Snowflake. Data changes rapidly. You must keep up.
2. You must be able to prove to the business that you have a least privileged access mechanism. Data is accessed only when there is a valid business purpose.
3. You must be able to protect data at rest and in motion within Snowflake. This means cell level encryption using a BYOK approach, near-real-time data activity monitoring, and data theft prevention in the form of DLP.
The three issues mentioned above are incredibly difficult for 95% of businesses to solve, largely due to the sheer scale and complexity of these challenges. Terabytes of data and growing daily, more users with more applications, trusted third parties who want to collaborate with your data. All of this leads to an unmanageable set of internal processes that slow down the business and provide risk.
ALTR’s easy-to-use solution allows Virgin Pulse Data, Reporting, and Analytics teams to automatically apply data masking to thousands of tagged columns across multiple Snowflake databases. We’re able to store PII/PHI data securely and privately with a complete audit trail. Our internal users gain insight from this masked data and change lives for good.
- Andrew Bartley, Director of Data Governance
I believed the CISO at this company was either too focused on the ACCOUNTADMIN problem to understand their other risks, or felt he had control over the other non-admin accounts. In either case I would have liked to learn more!
There was a reason someone from the Data & Analytics team sought out a product like ALTR. Data teams are afraid of screwing up. People are scared to store and use sensitive data in Snowflake. That is what ALTR solves for, not the task of ACCOUNTADMIN security. I wanted to be able to walk the CISO through the risks and how others have solved for them using ALTR.
The tools that Snowflake provides to secure and lock down the ACCOUNTADMIN role are robust and simple to use. Ensure network policies are in place. Ensure MFA is enabled. Ensure you have logging of ACCOUNTADMIN activity to watch all access.
I wish I could have been on the conversation with the CISO to ask a simple question, “If I show you how to control the ACCOUNTADMIN role on your own, would that change your tone on your teams use of ALTR?” I don’t know the answer they would have given, but I know the answer most CISO’s would give.
Nothing will ever be 100% secure and I am by no means saying ALTR can protect your Snowflake data 100% by using our platform. Data security is all about reducing risk. Control the things you can, monitor closely and respond to the things you cannot control. That is what ALTR provides day in and day out to our customers. You can control your ACCOUNTADMIN on your own. Let us control and monitor the things you cannot do on your own.

Oct 14
0
min
How Cloud Data Security Enables Business Outcomes without Sacrificing Compliance
Since 2015 the migration of corporate data to the cloud has rapidly accelerated. At the time it was estimated that 30% of the corporate data was in the cloud compared to 2022 where it doubled to 60% in a mere seven years. Here we are in 2024, and this trend has not slowed down.
Over time, as more and more data has moved to the cloud, new challenges have presented themselves to organizations. New vendor onboarding, spend analysis, and new units of measure for billing. This brought on different cloud computer-related cost structures and new skillsets with new job titles. Vendor lock-in, skill gaps, performance and latency and data governance all became more intricate paired with the move to the cloud. Both operational and transactional data were in scope to reap the benefits promised by cloud computing, organizational cost savings, data analytics and, of course, AI.
The most critical of these new challenges revolve around a focus on Data Security and Privacy. The migration of on-premises data workloads to the Cloud Data Warehouses included sensitive, confidential, and personal information. Corporations like Microsoft, Google, Meta, Apple, Amazon were capturing every movement, purchase, keystroke, conversation and what feels like thought we ever made. These same cloud service providers made this easier for their enterprise customers to do the same. Along came Big Data and the need for it to be cataloged, analyzed, and used with the promise of making our personal lives better for a cost. The world's population readily sacrificed privacy for convenience.
The moral and ethical conversation would then begin, and world governments responded with regulations such as GDPR, CCPA and now most recently the European Union’s AI Act. The risk and fines have been in the billions. This is a story we already know well. Thus, Data Security and Privacy have become a critical function primarily for the obvious use case, compliance, and regulation. Yet only 11% of organizations have encrypted over 80% of their sensitive data.
With new challenges also came new capabilities and business opportunities. Real time analytics across distributed data sources (IoT, social media, transactional systems) enabling real time supply chain visibility, dynamic changes to pricing strategies, and enabling organizations to launch products to market faster than ever. On premise applications could not handle the volume of data that exists in today’s economy.
Data sharing between partners and customers became a strategic capability. Without having to copy or move data, organizations were enabled to build data monetization strategies leading to new business models. Now building and training Machine Learning models on demand is faster and easier than ever before.
To reap the benefits of the new data world, while remaining compliant, effective organizations have been prioritizing Data Security as a business enabler. Format Preserving Encryption (FPE) has become an accepted encryption option to enforce security and privacy policies. It is increasingly popular as it can address many of the challenges of the cloud while enabling new business capabilities. Let’s look at a few examples now:
Real Time Analytics - Because FPE is an encryption method that returns data in the original format, the data remains useful in the same length, structure, so that more data engineers, scientists and analysts can work with the data without being exposed to sensitive information.
Data Sharing – FPE enables data sharing of sensitive information both personal and confidential, enabling secure information, collaboration, and innovation alike.
Proactive Data Security– FPE allows for the anonymization of sensitive information, proactively protecting against data breaches and bad actors. Good holding to ransom a company that takes a more proactive approach using FPE and other Data Security Platform features in combination.
Empowered Data Engineering – with FPE data engineers can still build, test and deploy data transformations as user defined functions and logic in stored procedures or complied code will run without failure. Data validations and data quality checks for formats, lengths and more can be written and tested without exposing sensitive information. Federated, aggregation and range queries can still run without fail without the need for decryption. Dynamic ABAC and RBAC controls can be combined to decrypt at runtime for users with proper rights to see the original values of data.
Cost Management – While FPE does not come close to solving Cost Management in its entirety, it can definitely contribute. We are seeing a need for FPE as an option instead of replicating data in the cloud to development, test, and production support environments. With data transfer, storage and compute costs, moving data across regions and environments can be really expensive. With FPE, data can be encrypted and decrypted with compute that is a less expensive option than organizations' current antiquated data replication jobs. Thus, making FPE a viable cost savings option for producing production ready data in non-production environments. Look for a future blog on this topic and all the benefits that come along.
FPE is not a silver bullet for protecting sensitive information or enabled these business use cases. There are well documented challenges in the FF1 and FF3-1 algorithms (another blog on that to come). A blend of features including data discovery, dynamic data masking, tokenization, role and attribute-based access controls and data activity monitoring will be needed to have a proactive approach towards security within your modern data stack. This is why Gartner considers a Data Security Platform, like ALTR, to be one of the most advanced and proactive solutions for Data security leaders in your industry.

Oct 2
0
min
What is Format-Preserving Encryption & Why It’s the Missing Piece in Your Security Strategy
Securing sensitive information is now more critical than ever for all types of organizations as there have been many high-profile data breaches recently. There are several ways to secure the data including restricting access, masking, encrypting or tokenization. These can pose some challenges when using the data downstream. This is where Format Preserving Encryption (FPE) helps.
This blog will cover what Format Preserving Encryption is, how it works and where it is useful.
What is Format Preserving Encryption?
Whereas traditional encryption methods generate ciphertext that doesn't look like the original data, Format Preserving Encryption (FPE) encrypts data whilst maintaining the original data format. Changing the format can be an issue for systems or humans that expect data in a specific format. Let's look at an example of encrypting a 16-digit credit card number:

As you can see with a Standard Encryption type the result is a completely different output. This may result in it being incompatible with systems which require or expect a 16-digit numerical format. Using FPE the encrypted data still looks like a valid 16-digit number. This is extremely useful for where data must stay in a specific format for compatibility, compliance, or usability reasons.
>>>You Might Also Like: FPE vs Tokenization vs TSS
How does Format Preserving Encryption work?
Format Preserving Encryption in ALTR works by first analyzing the column to understand the input format and length. Next the NIST algorithm is applied to encrypt the data with the given key and tweak. ALTR applies regular key rotation to maximize security. We also support customers bringing their own keys (BYOK). Data can then selectively be decrypted using ALTR’s access policies.
Why use Format Preserving Encryption
FPE offers several benefits for organizations that deal with structured data:
1. Adds extra layer of protection: Even if a system or database is breached the encryption makes sensitive data harder to access.
2. Original Data Format Maintained: FPE preserves the original data structure. This is critical when the data format cannot be changed due to system limitations or compliance regulations.
3. Improves Usability: Encrypted data in an expected format is easier to use, display and transform.
4. Simplifies Compliance: Many regulations like PCI-DSS, HIPAA, and GDPR will mandate safeguarding, such as encryption, of sensitive data. FPE allows you to apply encryption without disrupting data flows or reporting, all while still meeting regulatory requirements.
When to use Format Preserving Encryption?
FPE is widely adopted in industries that regularly handle sensitive data. Here are a few common use cases:
- Healthcare: Hospitals and healthcare providers could use FPE to protect Social Security numbers, patient IDs, and medical records. It ensures sensitive information is encrypted while retaining the format needed for billing and reporting.
- Telecoms: Telecom companies can encrypt phone numbers and IMSI (International Mobile Subscriber Identity) numbers with FPE. This allows the data to be securely transmitted and processed in real-time without decryption.
- Government and Defense: Government agencies can use FPE to safeguard data like passport numbers and classified information. Preserving the format ensures seamless data exchange across systems without breaking functionality.
- Data Sharing: In this blog we talk about how FPE can help with Snowflake Data Sharing use cases.
Wrapping Up
ALTR offers various masking, tokenization and encryption options to keep all your Snowflake data secure. Our customers are seeing the benefit of Format Preserving Encryption to enhance their data protection efforts while maintaining operational efficiency and compliance. For more information, schedule a product tour or visit the Snowflake Marketplace.
Browse All
Thank you! Your submission has been received!
Oops! Something went wrong while submitting the form.

Oct 12
0
min
8 Signs It's Time to Leverage Data Tokenization
ALTR Blog
In a world swirling with data at every turn, protecting sensitive information has transcended from a mere consideration to an absolute imperative. Enter data tokenization — a cutting-edge method which morphs critical data into a cryptic string of characters or tokens. The beauty of tokenization lies in shielding the original data from prying eyes and ensuring its essence and functionality remain intact.
Whether you're a budding startup, an established enterprise, or an individual trying to navigate the vast seas of the digital realm, understanding the nuances of tokenization is paramount. So, when should you harness the power of this digital knight in shining armor? Let's unravel the telltale signs that herald the need for data tokenization in your arsenal:
- You Handle Sensitive Data
If your organization deals with sensitive data, tokenization should be on your radar. Think about a typical e-commerce site. When customers shop, they provide information from credit card numbers to shipping addresses. Similarly, banks, beyond just account details, manage various documents, from loan applications to transaction histories. Meanwhile, healthcare providers store patient records, medication histories, and appointment details. With the increasing volume of sensitive information at stake, security becomes paramount. One significant breach can lead to financial losses and damage trust and reputation. If you're handling this kind of sensitive data, integrating tokenization into your security protocol offers a vital layer of added data protection.
- You're Subject to Complex Compliance Requirements
Regulatory compliance isn't just a checkbox – it's a fundamental requirement that ensures businesses operate within the bounds of the law and prioritize customer data security. Different sectors come with their unique set of standards. For instance, if you're in the payment industry, you'd be familiar with PCI DSS (Payment Card Industry Data Security Standard), which lays out rigorous requirements for handling cardholder data. Similarly, healthcare providers must adhere to HIPAA (Health Insurance Portability and Accountability Act), ensuring patient information is treated with utmost confidentiality and security. If you're grappling with the intricacies of such compliance or seeking more efficient ways to ensure your organization stays compliant, tokenization could be your guiding star.
- Your Organization Supports Remote Work
The wave of remote work, which began as a necessity in many cases, has become a staple of modern business culture. While this shift has brought about flexibility and broader talent access, it has also introduced a set of challenges in data security. As employees log in from various locations, devices, and networks, the number of endpoints – or access points – in an organization's data system has grown exponentially. This proliferation creates multiple gateways for potential cyber threats and amplifies the risk of unintentional data exposures by employees working in less secure environments. Now, imagine if the sensitive data they access were tokenized. Instead of the actual data, what's being accessed remotely would be a set of randomized tokens, which, even if intercepted or accidentally leaked, would be meaningless and unusable. In essence, tokenization acts as a protective shield, ensuring that while your team enjoys the flexibility of remote work, your sensitive data remains uncompromised, no matter where it's accessed.
- You Utilize Third-Party Integrations
Third-party interactions are ubiquitous, whether a payment gateway integration on an e-commerce site, using cloud services for storage, or a CRM system managed by an external provider. However, each integration presents a doorway; unfortunately, not all doors are impenetrable. With every added connection, the surface area vulnerable to potential cyberattacks or mishandling expands. Here's where the magic of tokenization becomes invaluable. In the event of a security breach on the vendor's side or any inadvertent mishap, your data is rendered meaningless. Tokenization ensures that the data you entrust them with remains in a protective cocoon, minimizing potential risk.
- You're Embracing the Cloud or Multi-Cloud Environments
Beyond just adopting a single cloud solution, your organization may have decided to leverage multi-cloud strategies, harnessing the strengths of various cloud providers to optimize your operations. While this multi-cloud approach offers redundancy, agility, and tailored solutions, it also introduces a spiderweb of complexity in data security. Each cloud provider has its unique architecture, security protocols, and access controls. Managing sensitive data across these diverse platforms while ensuring consistent security becomes a Herculean task. Enter tokenization. Tokenization is a universal security protecting data, whether in transit or at rest.
- You're in an Era of Rapid Business Growth
Every new customer, transaction, or market entry translates into more data points to guard. Whether it's proprietary business intelligence, customer personal details, or transaction histories, the databank swells as the business thrives. But herein lies a potential pitfall: can your data security measures keep pace as your business scales?
This is where implementing tokenization early in the growth journey becomes invaluable. With tokenization, security isn't a reactive measure, constantly playing catch-up with growth. Instead, it's proactive, creating a scalable and consistent protective layer, providing peace of mind in the fast-paced world of business expansion.
- You Rely on Data Analytics
Advanced analytics can unveil patterns, predict trends, and guide strategic decisions, fueling an organization's growth and innovation. However, diving deep into this sea of data often means accessing sensitive information, whether individual customer behaviors, purchasing histories, or proprietary business metrics. This poses a problem: How can your business glean actionable insights without risking the exposure of delicate information?
Tokenization transforms sensitive data into a series of randomized tokens that retain the structure and utility of the original data but mask its actual content. As a result, analysts can run their algorithms, build models, and generate reports using this tokenized data, reaping the benefits of data-driven insights without contacting sensitive information. In doing so, tokenization makes a bridge, harmoniously linking the need for comprehensive data analytics with the imperative of data security.
- You Lack a Multilayered Data Security Approach
Relying on a single line of defense is both naive and risky. If your organization doesn't have a layered approach to data security, you're leaving the door open for potential breaches. Tokenization can be a critical component of a comprehensive, multilayered security strategy. When combined with other security measures like encryption, firewall protection, and intrusion detection systems, tokenization ensures that even if one layer is compromised, others remain intact to guard your sensitive data. If you recognize that your security measures are overly simplistic or singularly focused, it might be a clear sign that you need to integrate tokenization into your data protection strategy.
Wrapping Up
Data tokenization isn't just a buzzword; it's a practical solution for organizations of all sizes and industries to secure sensitive information. Recognizing the signs that you might need tokenization is the first step toward a comprehensive data protection strategy. With the increasing complexities of the digital age, taking proactive steps to ensure the security and privacy of data is paramount.
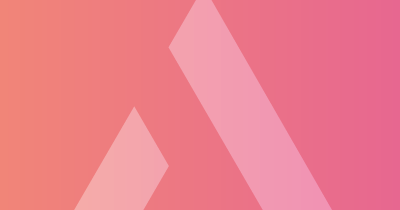
Sep 27
0
min
Navigating GDPR: A Comprehensive Guide to Achieving Regulatory Compliance
ALTR Blog
Personal data has become a precious asset, underscoring the imperative to safeguard individuals' privacy and data rights. The General Data Protection Regulation (GDPR) stands as a groundbreaking legal framework, purpose-built to protect individuals' personal data and impose rigorous regulations upon organizations engaged in its collection and processing.
In this blog, we'll navigate the essence of GDPR, its far-reaching ramifications across diverse industries, the indispensable measures for GDPR compliance, and the central significance of robust data governance and security in realizing and sustaining this compliance.
What is GDPR?
GDPR, or the General Data Protection Regulation, is a comprehensive data protection regulation implemented by the European Union (EU) in May 2018. GDPR applies not only to organizations based within the EU but also to those outside of it, provided they process the personal data of EU residents.
GDPR Principles
The General Data Protection Regulation (GDPR) is built upon six fundamental principles organizations must adhere to when processing personal data. These principles are designed to ensure that individuals' data rights are respected and that data processing is lawful and ethical.
Lawfulness, Fairness, and Transparency
This principle emphasizes that data processing must be conducted lawfully and have a legal basis. Organizations must be transparent about collecting and processing personal data, providing individuals with clear and easily understandable information about their data practices. The processing must also be fair, ensuring that individuals are not treated unfairly or deceived.
Purpose Limitation
Organizations are required to collect and process personal data for specified, explicit, and legitimate purposes. In other words, data should only be used for the initially collected purposes, and any additional use should be compatible with those original purposes.
Data Minimization
The principle of data minimization dictates that organizations should only collect and retain personal data necessary for the intended purpose. Excessive or irrelevant data should not be collected, and data should be kept current.
Accuracy
GDPR requires that personal data be accurate and, if necessary, kept up to date. Organizations must take reasonable steps to ensure that inaccurate or outdated data is rectified or erased promptly.
Storage Limitation
Personal data should not be kept longer than is necessary for the purposes for which it was collected. Organizations must establish data retention policies that specify how long different data types will be retained and for what reasons. Data that is no longer needed should be securely deleted.
Integrity and Confidentiality
This principle emphasizes the need to protect personal data against unauthorized or unlawful processing, as well as against accidental loss, destruction, or damage. Organizations must implement appropriate technical and organizational measures to ensure data security, including tokenization, encryption, access controls, and regular security assessments.
Accountability
Organizations are responsible for and must be able to demonstrate compliance with the principles of GDPR. This includes maintaining records of processing activities, conducting Data Protection Impact Assessments (DPIAs) where necessary, and appointing Data Protection Officers (DPOs) where required.
The Role of Data Security and Data Governance
Data security and data governance play pivotal roles in achieving and maintaining compliance with the General Data Protection Regulation (GDPR).
Data Security
Data security is the foundation of GDPR compliance and involves safeguarding personal data against unauthorized access, breaches, or misuse. GDPR emphasizes the importance of protecting personal data through technical and organizational measures. Here's how data security is vital in GDPR compliance:
Data Protection Methods:
Implementing robust data protection measures, including data masking techniques such as tokenization and encryption, helps secure data in transit and at rest, rendering it unintelligible without the corresponding decryption or detokenization key, even in the event of unauthorized access.
Access Controls:
Strict access controls and authentication mechanisms ensure that only authorized personnel can access personal data. This includes role-based access control, strong password policies, and multi-factor authentication.
Regular Security Assessments:
Regular security assessments and audits identify vulnerabilities and weaknesses in your data processing systems. These assessments help in addressing security issues proactively.
Data Breach Prevention and Response:
Robust data breach prevention mechanisms and a well-defined incidence response plan are critical. GDPR mandates that organizations promptly report any data breaches within a strict 72-hour timeframe.
Data Governance
Data governance encompasses the policies, processes, and procedures that ensure the quality, integrity, and protection of data within an organization. In GDPR compliance, data governance plays a significant role in managing personal data throughout its lifecycle. Here's how data governance contributes to GDPR compliance:
Data Mapping and Classification:
Data governance involves identifying and classifying personal data, understanding where it resides, and documenting its flow within the organization. This is crucial for GDPR's data inventory and documentation requirements.
Data Protection Impact Assessments (DPIAs):
Data governance helps conduct DPIAs, which are mandatory for high-risk processing activities. DPIAs assess the impact of data processing on individuals' privacy and identify measures to mitigate risks.
Data Retention and Erasure:
Data governance policies dictate data retention and erasure practices, ensuring that personal data is not kept longer than necessary. GDPR mandates the right to erasure, often referred to as the "right to be forgotten."
Data Subject Rights:
Data governance processes should support data subject rights, such as access, rectification, and objection. Data governance ensures that organizations can respond promptly to these requests.
Data Portability:
Data governance should enable the data portability requirement of GDPR, allowing individuals to obtain and reuse their personal data for their own purposes across different services.
Documentation and Records:
Data governance assists in maintaining the required records of processing activities, privacy policies, and documentation necessary to demonstrate GDPR compliance.
Accountability:
Effective data governance demonstrates accountability, a fundamental GDPR principle. It ensures that organizations take responsibility for their data processing activities and can provide evidence of compliance.
Impact of GDPR on Key Industries
GDPR's impact reverberates across various industries, compelling organizations to prioritize data protection, transparency, and individual rights. Compliance is not just a legal requirement but also a testament to an organization's commitment to preserving the integrity of personal data in an increasingly data-driven world.
Healthcare
In the healthcare sector, GDPR places a paramount emphasis on safeguarding patient data. Healthcare organizations must adhere to stringent data protection measures, ensuring patient records remain confidential and secure. Access control mechanisms are implemented to limit data access to authorized personnel only, and there is a heightened focus on timely breach notification, underscoring the significance of transparency and swift action in case of a data breach.
Finance
Financial institutions handle a treasure trove of sensitive financial and personal information, making GDPR compliance imperative. GDPR entails establishing robust data security measures for these organizations, including secure data storage and transaction tracking. Comprehensive data protection measures becomes a standard practice to protect financial data, bolstering trust and ensuring regulatory compliance.
E-commerce
Online retailers rely heavily on customer data for personalized shopping experiences. Under GDPR, they are tasked with ensuring that customer data is processed securely. Obtaining explicit consent for marketing communications becomes crucial, and mechanisms for easy opt-out must be readily available to customers. GDPR obligates e-commerce companies to strike a delicate balance between data-driven personalization and individual privacy rights.
Technology
Often at the forefront of data innovation, tech companies must adopt a "privacy by design and default" approach. This means that data protection must be integrated into the very fabric of their products and services. From social media platforms to software developers, tech companies must prioritize user privacy, emphasizing data protection as an integral part of their offerings.
Marketing
Marketers relying heavily on customer data for targeted campaigns must navigate GDPR's stringent requirements for data processing. Explicit consent for data processing is mandated, and users must have the option to opt-out seamlessly from data collection and marketing initiatives. GDPR challenges marketers to build trust through transparent practices while delivering effective campaigns.
Education
Educational institutions handling vast amounts of student and staff data must adhere to GDPR's strict consent and data retention rules. This means obtaining explicit and informed consent for data processing activities and ensuring that data is retained only for as long as necessary. GDPR underscores the importance of protecting the sensitive information of students and staff within the educational sector.
GDPR Penalties and Enforcement
GDPR places a significant emphasis on compliance, and to incentivize organizations to adhere to its principles and requirements, it introduces a comprehensive framework of fines and enforcement measures for non-compliance. Understanding these penalties is essential for organizations striving to meet GDPR standards. Below, we clarify the potential fines and penalties for non-compliance and provide examples of GDPR enforcement actions:
Administrative Fines
GDPR empowers supervisory authorities, such as Data Protection Authorities (DPAs), to impose administrative fines on organizations that fail to comply with the regulation. These fines can be substantial and vary depending on the severity of the violation. There are two tiers of penalties:
- Lower Tier: For less severe infringements, organizations can be fined up to €10 million or 2% of their global annual turnover, whichever is higher.
- Upper Tier: More severe violations can lead to fines of up to €20 million or 4% of the organization's global annual turnover, whichever is higher.
Warnings and Reprimands
In addition to fines, supervisory authorities can issue warnings and reprimands to organizations for non-compliance. These serve as initial steps to encourage corrective actions and compliance with GDPR.
Suspension of Data Processing
Supervisory authorities can temporarily or permanently suspend data processing activities if they find that an organization's processing activities infringe on individuals' rights and freedoms.
Withdrawal of Certifications
If an organization holds GDPR certifications or seals, such as Privacy Shield certifications, the supervisory authority can withdraw these certifications if the organization fails to meet GDPR standards.
Examples of GDPR Enforcement Actions
TikTok (2023): Irish Data Protection Commissioner (DPC) fined TikTok €345m for breaching a number of GDPR rules, including putting 13-17-year-old users' accounts on default public setting.
Meta Platforms (2022): Meta Platforms Ireland Limited (MPIL), the data controller of 'Facebook' social media network, was issued a fine of €265m along with corrective measures.
Google (2019): The French data regulator (CNIL) fined Google €50 million for a "lack of transparency, inadequate information and lack of valid consent regarding ads personalisation"
Wrapping Up
GDPR has ushered in a new era of data protection and privacy rights. Complying with this regulation is essential to avoid hefty fines and build trust with customers and stakeholders. By conducting thorough data audits, implementing robust security measures, and prioritizing data governance, organizations can comply with GDPR and elevate their data protection standards, benefiting both their business and the individuals they serve.

Sep 20
0
min
11 Data Security Mistakes You Should Never Make
ALTR Blog
Data breaches often likened to digital earthquakes, have the potential to rattle organizations to their core. They can bring about a tsunami of consequences, from crippling financial losses and tattered reputations to mounting legal liabilities. In this turbulent digital landscape, the unsung heroes, data security teams, are vigilant guardians of an organization's most valuable asset: sensitive information. Yet, even the steadiest hands can falter, and even the sharpest minds can slip. In this blog, we'll explore 11 data security mistakes that data security teams must avoid.
1. Weak Password Policies
Passwords serve as the first line of defense against unauthorized access, and their strength directly correlates with an organization's vulnerability to cyberattacks. Without robust password policies, attackers can exploit the weakest link in the security chain - user passwords.
Data security teams must emphasize the importance of strong password policies to mitigate this risk. Password complexity requirements, including uppercase and lowercase letters, numbers, and special characters, create formidable barriers against brute-force attacks. Regular password changes further fortify this defense, reducing the window of opportunity for malicious actors. Multi-factor authentication (MFA) is the crown jewel of password security, as it adds an additional layer of protection by requiring users to provide two or more forms of verification before gaining access.
2. Inadequate Access Control
Inadequate access control is a recipe for disaster in data security. Allowing users or systems to have more access privileges than necessary is akin to leaving the vault door ajar in a bank; it invites trouble. Once inside the strategy, hackers can exploit these overly permissive access rights to move laterally, access sensitive data, and wreak havoc with impunity.
Data security teams must embrace the "least privilege" principle as their guiding philosophy to avert this threat. This principle revolves around granting users and systems the absolute minimum access required to fulfill their designated tasks. By adhering to this principle, teams ensure that only authorized personnel can access specific data or resources, mitigating the risk of unauthorized access.
Moreover, access control should be dynamic and evolving in response to changing organizational roles and responsibilities. When an employee's role changes or leaves the company, their access rights should promptly reflect these adjustments. Access control is not a one-time task but a continuous process that demands vigilance and adaptability.
3. Failure to Address Known Vulnerabilities
Despite the constant evolution of threats and the release of security patches and updates, some organizations neglect to apply these fixes to their systems and software promptly. This oversight can be catastrophic, as cybercriminals often target well-documented vulnerabilities to exploit weaknesses in an organization's defenses. Data security teams must prioritize vulnerability management by establishing a robust patch management process, conducting regular vulnerability assessments, and promptly addressing identified vulnerabilities. Failing to do so not only leaves an organization exposed to known risks but also undermines the integrity and credibility of its data security efforts.
4. Neglecting Data Classification
Data classification is a critical aspect of data security that often goes overlooked. Incorrectly classifying data based on its sensitivity and importance can lead to mishandling and inadequate protection. Data security engineers should implement a robust data classification system that categorizes data into different levels, enabling organizations to apply appropriate security controls, access restrictions, and encryption based on the data's classification.
5. Disregarding Data Masking
Failure to implement data masking exposes sensitive data in non-production environments, making them attractive targets for data breaches or unauthorized access. This can occur when developers, testers, or other personnel inadvertently expose sensitive information while working with datasets that mirror real production data.
Data security engineers must recognize that not all employees or stakeholders require access to actual sensitive data in non-production settings. Neglecting data masking in these environments is a mistake that can lead to privacy violations, regulatory non-compliance, and significant reputational damage. By adopting data masking as a standard practice, organizations can balance data utility and protection, ensuring that sensitive information remains secure while enabling essential business processes to continue uninterrupted.
6. Not Regularly Backing Up Data
Data loss is a specter that haunts organizations across the digital landscape, often lurking in the shadows, waiting for the opportune moment to strike. It doesn't discriminate; it can manifest through malicious cyberattacks, unrelenting hardware failures, or the simple slip of a keystroke in the hands of well-intentioned employees. Not regularly backing up data in this precarious environment is akin to walking a tightrope without a safety net.
Data security teams must establish robust and automated backup processes that operate as the organization's safety net. These processes ensure that critical data is captured, encrypted, and stored regularly. The importance of regularity cannot be overstated; it's the difference between recovery and irreversible loss when disaster strikes.
7. Inadequate Incident Response and Disaster Recovery Plans
One of the most pivotal data security mistakes an organization can make is neglecting to establish a comprehensive Incident Response Plan (IRP) and Disaster Recovery Plan (DRP).
An Incident Response Plan is a roadmap that outlines how an organization will react when a data security incident occurs. It defines roles, responsibilities, and procedures for promptly detecting, reporting, and mitigating security breaches. Without an IRP, chaos may ensue, response times may lag, and critical evidence could be lost, exacerbating the impact of the incident.
Similarly, a Disaster Recovery Plan focuses on the organization's ability to recover and restore data and operations in the aftermath of a disaster, whether a cyberattack, natural disaster, or system failure. Neglecting a DRP can result in extended downtime, loss of vital data, and significant financial setbacks.
8. Overlooking Data Migration Security
Data migration is a complex process that involves transferring data from one system or location to another. It's a prime opportunity for data security mistakes if not handled carefully. Data security teams must ensure that the migrated data is adequately protected. This includes encrypting data in transit, validating data integrity before and after migration, and conducting thorough testing to avoid potential data leakage or corruption during migration. Moreover, teams should plan for the decommissioning or secure disposal of old systems or storage media after migration to prevent data exposure. Additionally, considering compliance requirements and regulations during data migration is crucial to avoid legal and regulatory pitfalls.
9. Failure to Recognize the Need for Centralized Data Security
Data is often dispersed across various systems, departments, and even cloud services in a modern organization. Failing to establish a centralized approach to data security can result in fragmented security measures, making it challenging to enforce consistent policies, monitor threats comprehensively, and respond effectively to security incidents. Data security teams must understand that a centralized approach streamlines security management and ensures that data protection strategies are cohesive and aligned with the organization's overall security objectives. Ignoring the necessity of centralized data security is a mistake that can leave an organization vulnerable to breaches and data leaks.
10. Forgetting to Assign Responsibility for the Data
A critical data security mistake is the failure to assign responsibility for the data. When no one is accountable for data security, it often leads to a lack of ownership and oversight. This can confuse who should implement security measures, enforce policies, and respond to data breaches. Assigning responsibility for data security ensures that individuals or teams are dedicated to safeguarding sensitive information, regularly assessing risks, and staying updated with evolving threats and compliance requirements. Without clear ownership, an organization is more susceptible to data security lapses and may struggle to establish a cohesive and effective security posture.
11. Insufficient Employee Training
Even the most robust technological defenses can be compromised if employees are not adequately educated and aware of security best practices. In the digital age, where phishing attacks, social engineering tactics, and other forms of cyber manipulation are prevalent, employees serve as the frontline defense.
Without proper training, employees may inadvertently click on malicious links, share sensitive information with unauthorized individuals, or fall victim to phishing scams. These actions can lead to data breaches with significant consequences, including financial losses and damage to the organization's reputation.
Data security teams must recognize that technology alone cannot thwart all threats. Ongoing, comprehensive training programs are essential to ensure that employees are not the weakest link in the security chain.
Wrapping Up
Data security is an ongoing process that requires vigilance and a proactive approach. By avoiding these eleven common data security mistakes and implementing robust security measures, data security teams can help protect their organizations from the ever-evolving threat landscape. Remember, in data security, it's not a matter of if a breach will occur but when, so being prepared is essential to minimize damage and maintain trust.

Sep 13
0
min
Security in a Modern Business Intelligence Architecture
ALTR Blog
In the data-rich landscape of today's business world, companies are perpetually on the hunt for innovative methods to tap into the potential of their data. They yearn to transform the vast sea of information at their fingertips into a strategic advantage, to make decisions that are not just educated but visionary. And in this ambitious quest for data-driven excellence, Business Intelligence (BI) emerges as the unsung hero. Yet, as the digital realm becomes increasingly treacherous, where data is king and vulnerability is the enemy, safeguarding your BI architecture has catapulted priorities to the forefront.
In this blog, we'll delve into crucial security parameters of a business intelligence architecture, including thoughtful insights from experts in the modern data ecosystem.
- Access Control
Controlling who has access to your BI platform and what they can do with that access is fundamental to security. Fine-grained access control mechanisms should be in place to restrict users to only the data and functionalities they need for their roles.
Chris Struttman, CTO, ALTR
Security is being able to protect the data from unauthorized access but keeping the data functional so that it can still be operated on. This allows data and security teams to achieve their goals harmoniously.
- Encryption
Data is the lifeblood of any BI system, and securing it from unauthorized access or theft is a top priority. Modern BI architectures employ robust encryption techniques to protect data at rest and in transit. This includes encrypting data stored in databases, data warehouses, and during data transfer between various components of the BI system.
John Bagnall, Senior Product Manager, Matillion
It's about applying strong access controls, data encryption, routine audits and adherence to regulations. Keeping on top of protecting sensitive information prevents unauthorized access and maintains data confidentiality. Using this for secure data transmission, robust authentication methods, and ongoing monitoring for potential threats is and should be paramount. A clear and comprehensive strategy is essential to address internal and external risks.
- Data Masking
Sensitive data should be masked to protect privacy and comply with regulations like GDPR. Data masking ensures that only authorized individuals can see complete data while others view masked or scrambled versions. This is especially important when sharing reports or dashboards externally or with third-party vendors.
- Data Classification
Data classification is a pivotal aspect of modern business intelligence security. It involves categorizing data based on sensitivity, enabling organizations to apply appropriate security measures. By classifying data like "public," "internal," and "confidential," businesses can determine who should have access and what level of protection is necessary for each type of data.
Pat Dionne, President & CEO, Passerelle
Modern BI architecture has evolved with new tools that focus solely on data controls and take the burden of protecting data from the Business Intelligence tool layer. Concepts such as Tags and Policies provide the ability to secure data at scale more effectively. Coupled with traditional concepts such as data classification, rows, columns and object-level access, you can construct very granular data access policies to enable/support the modern data-driven organization.
- Auditing and Logging
Visibility into what happens within your BI system is crucial for detecting and responding to security incidents. Modern BI architectures incorporate robust auditing and logging capabilities, allowing administrators to monitor user activities, access to data, and system events. These logs can provide valuable insights into potential threats or suspicious behavior.
- Secure Data Integration
BI systems often require data from various sources, including on-premises databases, cloud services, and external APIs. Integrating these data sources securely is essential. Secure data integration practices involve using secure APIs, OAuth authentication, and data transformation processes that do not expose sensitive information.
- Regular Security Updates and Patch Management
Security vulnerabilities are discovered regularly in BI tools and underlying infrastructure components. To mitigate risks, it's crucial to stay updated with security patches and updates for all components of your BI architecture, including databases, BI servers, and any third-party tools or plugins.
- Employee Training and Awareness
No matter how robust your technical security measures are, employees are often the weakest link in the security chain. Comprehensive training and awareness programs can help employees effectively recognize and respond to security threats. This includes phishing awareness, password management best practices, and general cybersecurity training.
- Disaster Recovery and Business Continuity
Businesses need to be prepared for the unexpected. A well-designed disaster recovery plan ensures your BI system can quickly recover from data breaches, hardware failures, or natural disasters. Regularly testing and updating this plan is essential to minimize downtime and data loss.
- Compliance with Regulations
Depending on your industry and geographical location, you may need to comply with various data protection regulations like GDPR, HIPAA, or CCPA. Your BI architecture should be designed with these regulations, and data handling practices should align with their requirements.
Mohideen Risvi.Y, Lead Frontend Developer, Decision Minds
Security involves a multi-layered approach. It includes user authentication, role-based access control, encryption, and secure data transmission. Regular audits, monitoring, and updates are vital in maintaining a secure environment. Additionally, privacy regulations like GDPR must be considered when handling sensitive data.
- Continuous Monitoring and Threat Detection
Proactive security measures are crucial, as are continuous monitoring and threat detection. Employing security information and event management (SIEM) systems or other monitoring tools can help identify and respond to security incidents in real time.
Wrapping Up
Security in a modern business intelligence architecture is multifaceted and requires a combination of technical, organizational, and human-centric measures. As data grows, investing in a robust security strategy is not just a best practice but a necessity to protect your organization's sensitive information and maintain the trust of your customers and stakeholders. Remember that security is an ongoing process that evolves with the threat landscape, so staying informed and proactive is critical to maintaining a secure BI environment.

Sep 6
0
min
Mastering Data Classification for Security and Efficiency: A Complete Guide
ALTR Blog
Data classification is crucial in modern organizations, enabling them to effectively organize, secure, and derive value from their data assets. By categorizing data based on its sensitivity, business impact, and compliance requirements, data classification provides a foundation for effective data governance and security.
In this comprehensive guide, we will explore the concept of data classification, its importance, challenges, and the steps involved in implementing a data classification system. So, let's dive in and discover how data classification can revolutionize how you manage your data.
Understanding Data Classification
Data classification categorizes and labels data based on attributes, properties, or characteristics. The primary goal of data classification is to organize and manage data in a structured manner, making it easier to handle, protect, and utilize. This process involves assigning metadata tags, labels, or categories to data based on specific criteria, such as sensitivity, importance, content, or regulatory requirements.
The types of data classification can vary depending on the organization's needs and objectives. Common characteristics for classification include:
Content
This type of classification involves analyzing the actual content of data to categorize it. It may include keywords, file types, patterns, or specific data elements. Content-based classification is particularly useful for unstructured data like documents, emails, and multimedia files.
Context
Context-based classification considers metadata and contextual information associated with data. This includes details like data source, author, creation date, location, and how data relates to other information pieces. Context-based classification provides insights into data origin and usage, aiding decision-making.
Sensitivity
This classification type categorizes data based on its level of sensitivity. It involves assessing how confidential or private the information is, often applying labels like "public," "confidential," or "restricted." Sensitivity-based classification is crucial for implementing appropriate security measures.
Regulatory
Regulatory-based classification aligns data categories with specific regulatory requirements. Different industries are subject to various regulations (GDPR, HIPAA, etc.), and this classification ensures data is handled in accordance with these rules.
Lifecycle
Lifecycle-based classification considers the stage of the data's lifecycle. Data can be categorized as "active," "archived," or "deleted." This type helps organizations manage data storage, retention, and disposal effectively.
User
User-based classification allows individual users to assign classification labels based on their understanding of data. This type promotes user engagement and accountability in protecting and managing data.
Business Impact
This classification focuses on the significance of data to business operations. It helps prioritize data protection efforts by categorizing data as "critical," "important," or "non-essential."
Access
Access-based classification categorizes data based on the level of access required. Data can be labelled as "public," "internal," or "confidential," indicating who is authorized to view and modify it.
Time
Time-based classification categorizes data based on time-related criteria. Data might be classified as "current," "historical," or "upcoming," aiding in data retrieval and management.
Data Source
This type of classification is based on the origin of data. It could include labels like "customer data," "vendor data," or "employee data," helping manage and protect data from different sources.
When more data, columns and databases are added to your data warehouse, you need to ensure all data is governed accurately and quickly. Column headers can be deceptive, especially when you are managing tons of data, and the wrong data can exist in the wrong columns. It’s impossible, at scale, to manually check column by column and row by row for data accuracy, yet, knowing what data is sensitive you hold is the foundation for data access governance and security.
The Importance of Data Classification
Data classification is the foundation for various critical information management and security aspects. Here are some key reasons why data classification is essential for organizations:
Risk Management and Data Protection
Data classification enables organizations to identify and assess the risks associated with their data assets. By categorizing data based on its sensitivity and importance, organizations can prioritize their security efforts and implement appropriate controls to protect valuable or sensitive data from unauthorized access, loss, or theft. This proactive approach to risk management helps organizations mitigate potential threats and prevent data breaches.
Compliance and Regulatory Requirements
Many industries are subject to strict regulatory requirements that govern the handling, storage, and protection of specific data types. Data classification helps organizations comply with these regulations by ensuring that data is appropriately categorized and handled according to the relevant compliance standards. Organizations can avoid penalties, legal issues, and reputational damage by aligning data classification with regulatory requirements.
Efficient Data Storage and Retrieval
Organizations generate and accumulate vast amounts of data, making it challenging to store, manage, and retrieve information when needed efficiently. Data classification provides a structured framework for organizing data, making it easier to locate and retrieve specific information quickly. By categorizing data based on attributes, organizations can optimize storage resources, reduce data duplication, and improve overall data accessibility and usability.
Enhanced Data Governance and Decision-Making
Data classification lays the foundation for effective data governance practices. Organizations can establish clear guidelines and responsibilities for data management by categorizing data and assigning ownership and accountability. This promotes data integrity, accuracy, and consistency, enabling better decision-making based on reliable and trustworthy information.
Challenges in Data Classification
While data classification holds immense promise, it's not without its challenges. Implementing a data classification system requires addressing these hurdles to ensure its effectiveness and sustainability. Here are some of the key challenges organizations might encounter:
Data Accuracy and Consistency
Accurate data classification hinges on the quality and consistency of metadata and attributes used for classification. Inaccurate or inconsistent labeling can lead to misclassification, impacting security measures and decision-making. Ensuring data accuracy and maintaining consistent labeling standards are ongoing challenges that demand attention.
Evolving Data Landscape
Data is dynamic and constantly changing in form and context. Staying agile and updating classification criteria to reflect new data realities is essential to ensure the relevance and accuracy of the classification system. ALTR’s Classification processes remain up to date and ensure that as you continue to run classification on your data, all data remains healthy and accurate.
User Adoption and Compliance
For a data classification system to succeed, it needs to be embraced by users across the organization. Employees might resist the additional steps required for data classification, viewing it as cumbersome. Achieving widespread user adoption requires effective training, clear communication, and an understanding of how classification benefits them and the organization.
Balancing Automation and Human Judgment
While automation streamlines classification, there are instances where human judgment is critical. Striking the right balance between automated classification processes and involving human expertise is challenging. Overreliance on automation could lead to misclassifications, while too much manual intervention can slow down the process. ALTR’s easy to use point-and-click UI ensures that you are applying the correct tags to the correct data in real-time.
Privacy and Ethical Concerns
Classifying data based on sensitivity might inadvertently expose personal or sensitive information. Striking a balance between data classification for security purposes and respecting individual privacy rights can be complex. Organizations must ensure that sensitive data is appropriately protected, and data classification aligns with ethical guidelines.
Fortunately, ALTR sits at the intersection of Data Access Governance and Data Security, meeting the needs of both protection of sensitive data and proper data classification.
Critical Steps in Data Classification
Implementing a comprehensive data classification system involves several key steps. While the specific approach may vary depending on organizational requirements, here are some critical steps to consider:
- Define Data Classification Policies and Criteria - Establish written procedures and guidelines that define the categories and criteria for data classification within your organization. These policies should outline the attributes and characteristics used to classify data, such as sensitivity levels, business impact, regulatory requirements, and data ownership.
- Conduct Data Inventory and Assessment - Conduct a thorough inventory of your organization's data assets to identify the data types you handle, their locations, and their associated risks. Assess the sensitivity and importance of each data asset to determine the appropriate classification category.
- Develop a Classification Framework - Collaborate with relevant stakeholders, such as data scientists and business units, to develop a classification framework that aligns with your organization's needs and objectives. This framework should define the categories, labels, and metadata tags used to classify data consistently.
- Establish Security and Storage Standards - Identify security standards and best practices that align with each data classification category. Define appropriate handling practices, access controls, encryption requirements, and storage lifespan for each category. Implement storage standards that address data retention, archiving, and disposal.
- Implement Data Classification Tools and Technologies - Utilize data classification tools and technologies to automate and streamline the classification process. These tools can analyze data attributes, apply classification labels, and enforce security policies consistently across your data ecosystem.
- Train Employees and Foster Data Stewardship - Educate and train employees on data classification policies, procedures, and their roles and responsibilities in data stewardship. Foster a culture of data awareness and accountability to ensure consistent and accurate data classification throughout the organization.
- Regularly Review and Update Data Classification - Data classification is not a one-time effort. Regularly review and update your data classification system to adapt to evolving business needs, regulatory changes, and emerging data risks. Periodically assess the effectiveness and efficiency of your data classification practices and make necessary adjustments.
If sensitive data isn’t identified, it’s impossible to protect, leaving gaps in both privacy and security. ALTR integrates data classification into our policy enforcement engine, allowing users to automatically find, tag and enforce governance policy on data easily, all from the ALTR interface, as frequently as you need.
Tools and Technologies for Data Classification
Several tools and technologies can aid in the data classification process. Here are some commonly used tools:
Data Classification Software
Data classification software automates analyzing data attributes, assigning classification labels, and enforcing security policies. These tools utilize machine learning algorithms and pattern recognition techniques to classify data based on predefined criteria accurately.
ALTR’s data classification solution directly on Snowflake lets companies quickly identify and classify PII, PCI and PHI data so that it can be automatically controlled and secured. ALTR integrates with Snowflake’s Object Tagging functionality to import any Object Tags available in Snowflake. Two options are available for importing
Snowflake Object Tag data into ALTR:
- Importing any existing Object Tags available in Snowflake.
- Executing Snowflake Data Classification first and then importing all available object tags.
Data Loss Prevention (DLP) Solutions
Data loss prevention solutions help organizations identify and protect sensitive data from unauthorized access, loss, or leakage. These solutions can analyze data in real time, monitor data movement and access, and enforce policies to prevent data breaches. DLP solutions often incorporate data classification capabilities to identify sensitive data and apply appropriate protection measures.
ALTR’s Data Classification option via Google DLP Classification enables users to send a random sampling of their to Google’s DLP service for classification. In a Google DLP Classification, ALTR will select a random sample from each column in your Snowflake database and send that sample to Google DLP for analysis. Each column is sampled separately to protect the anonymity of data. Google’s DLP service returns possible classification results to ALTR, which associates those results to the affected columns as Data Tags.
With ALTR, you can automatically classify data directly in Snowflake, or via Google DLP – both options returning your classification results in minutes and into a robust Classification Report. Now you are able to apply policy based on categories and tags so your sensitive data remains secure, organized, and in compliance.
Wrapping Up
Data classification is a fundamental process that empowers organizations to manage, secure, and derive value from their data assets. By categorizing data based on attributes, organizations can implement appropriate security measures, ensure compliance with regulations, and optimize data storage and retrieval. With the right tools, technologies, and a robust data classification framework, organizations can unlock the full potential of their data and gain a competitive advantage in the digital landscape.
Classify Your Data for FREE on Snowflake
Are you ready to better understand what sensitive data you have? Start today for free with ALTR and:
- Automatically discover, classify and tag your data
- Control access to columns and rows of sensitive data with a click
Start Classifying: https://get.altr.com/free/

Aug 23
0
min
Data Security Essentials for Cloud Migration
ALTR Blog
As businesses continue to embrace the benefits of the cloud, the migration process presents a pivotal moment for organizations. While the cloud offers scalability, flexibility, and cost-efficiency, it also introduces several security risks.
In this blog, we'll delve into some common data security risks associated with cloud migration and share what experts think are critical security measures often overlooked and other essential considerations for a smooth, secure transition to the cloud.
Common Data Security Risks When Migrating to the Cloud
Lack of Visibility and Control: Cloud migration might result in reduced visibility and control over data, leading to challenges in monitoring, auditing, and enforcing security measures.
Misconfigured Access Controls: Poorly configured access controls could allow unauthorized users to access or modify sensitive data stored in the cloud, leading to data breaches.
Insider Threats: Employees with improper access or malicious intent could misuse their privileges to compromise or steal data during migration.
Shadow IT and Unauthorized Cloud Usage: Employees might use unauthorized cloud services, leading to data exposure and security risks that IT departments are unaware of.
Account Hijacking: Weak or compromised credentials could lead to unauthorized access to cloud accounts, enabling attackers to manipulate or steal sensitive data.
Data Interception during Transfer: Data transferred between on-premises systems, and the cloud can be intercepted if proper security mechanisms are not in place, leading to data leakage.
API Vulnerabilities: Cloud environments use APIs for communication between services. Attackers could exploit vulnerabilities in these APIs to gain unauthorized access to data.
Data Retention and Deletion: Inadequate data retention and deletion practices might result in sensitive data lingering in the cloud beyond its intended lifecycle, increasing the risk of exposure.
Expert Panel: What Security Measures Are Often Overlooked When Migrating to the Cloud?
Addressing the data security risks above requires a comprehensive approach that involves thorough risk assessment, proper planning, implementation of security controls, ongoing monitoring, and adherence to best practices in cloud security.
As part of our Expert Panel Series on LinkedIn, we asked experts in the modern data ecosystem what they think are the top security measures often overlooked when migrating to the cloud. Here's what we heard...
Pat Dionne, CEO & Cofounder, Passerelle
"Two aspects come to mind: data usage consent and monitoring for abnormal queries. Obtaining data usage consent for certain use cases is increasingly important and often overlooked in the rush to mine data for value. Monitoring for abnormal data queries based on a limits threshold will allow for detecting potential abnormal data usage and can prevent large data leaks."
James Beecham, Founder & CEO, ALTR
"Create a plan to prevent shadow IT! Listen to application and data users to ensure you meet their needs; otherwise, shadow IT will occur. Making a cloud migration plan in a closed room will only lead to problems."
Austin Ryan, Business Development Executive, ALTR
"Setting up an RBAC model and access policies is a great start, but the effort it takes to scale and maintain these policies is often overlooked. Every time you add new roles/users, migrate new data, create a change request, etc., there are manual tasks that typically fall in the laps of your already busy data engineers and slow down your entire organization. It loses much of its value if you can't manage these policies at scale and have secure real-time access to your data."
Damien Van Steenberge, Managing Partner, Codex Consulting
"Get your RBAC together! We often neglect it at the beginning of the project!"
Additional Security Must-Haves for Cloud Migration
Shift Left™ Abilities
Shifting Left means initiating robust data governance and security capabilities as the data leaves the source systems. Doing so ensures the policies are attached to, and remain with, the workload throughout the data journey to the cloud.
Data Classification
Categorize your data based on sensitivity levels, ensuring that highly sensitive information receives stricter security controls. This approach allows you to tailor security measures to the specific needs of each data type, minimizing the risk of data breaches and unauthorized access during migration and cloud operations.
Tokenization
By tokenizing sensitive data before transferring it to the cloud, you replace actual data with tokens, rendering the original information meaningless even if intercepted. This enhances data protection during migration, reducing the risk of exposure and unauthorized access to sensitive information.
API Security
Secure any APIs used for communication between applications and cloud services. Implement authentication, authorization, and rate limiting to prevent unauthorized access.
Data Residency and Compliance
Understand the regulatory requirements specific to your industry and ensure that your chosen cloud provider complies with them. Ensure data is stored in appropriate locations to meet data residency requirements.
Data Loss Prevention
Implement DLP solutions to monitor and prevent the unauthorized transfer or sharing of sensitive data. This helps prevent accidental data leakage or intentional data breaches.
Regular Data Backups
Implement a regular backup strategy to ensure that data can be restored in case of data loss, corruption, or a security incident—store backups in separate locations to mitigate risks.
Monitoring and Logging
Set up robust monitoring and logging mechanisms to detect unusual activities, unauthorized access attempts, and potential security breaches. Analyze logs to identify and respond to security incidents promptly.
Incident Response Plan
Develop a comprehensive incident response plan that outlines the steps to take during a security breach. This plan should include roles, responsibilities, communication procedures, and mitigation strategies.
Vendor Security Assessment
Assess the security practices of your chosen cloud provider. Understand how they handle data security, compliance, and incident response to ensure they meet your organization's requirements.
Data Deletion and Retention Policies
Establish clear policies for data retention and deletion. Ensure that data is deleted securely when no longer needed to prevent lingering data from being exposed.
Security Testing and Auditing
Regularly conduct security assessments, vulnerability scans, and penetration testing on your cloud infrastructure and applications. This helps identify and address potential security weaknesses.
Training and Awareness
Provide training to employees and stakeholders about cloud security best practices. Educate them on recognizing and responding to security threats, phishing attempts, and other risks.
Continuous Improvement
Cloud security is an ongoing process. Regularly review and update your security measures, staying informed about emerging threats and vulnerabilities.
Wrapping Up
Cloud migration can revolutionize an organization's operations, but without adequate security measures, the benefits can quickly become liabilities. Businesses can ensure a smooth, secure transition to the cloud by addressing these security measures. Remember that cloud security is an ongoing effort, requiring regular assessments, updates, and a proactive approach to stay ahead of evolving threats.
.png)
Aug 18
0
min
Shift Left: Why Governing and Securing Data from Source to Cloud Is Mission Critical
ALTR Blog
In today's fast-paced world, businesses are generating and accumulating data at an unprecedented rate. To maximize the value of data to the enterprise, any modern data architecture must contemplate how sensitive data is governed and protected across the entire data journey- from source, to the cloud, to users.
The focus for many companies is instilling effective data access governance and data security in their cloud destination, like Snowflake. However, risk, governance, compliance, and security stakeholders now recognize that those sensitive workloads should be subject to full data governance and protection before it lands in Snowflake. It’s no longer enough to rely on securing your data after it lands in a cloud data warehouse; data owners must protect data from the instant it migrates from a source system and throughout its entire journey to the cloud. This applies to data in ETL and ELT pipelines and transient storage mechanisms like GCS and Amazon S3 buckets.
ALTR’s unique architectural advantages allow any enterprise to easily extend robust data governance and security features on Snowflake upstream into data pipelines and data catalogs, guaranteeing the security of sensitive data throughout the entire data journey – something competitors cannot offer.
With data coming from many sources and the critical importance of securing that data upstream, the ability to shift your data governance and data security implementation left has become a necessary capability for the modern data enterprise.
What Does it Mean to “Shift Left”?
The modern data ecosystem faces a major issue with the complexity of moving highly sensitive data from on-premise systems, where it’s likely been held for years, to cloud data warehouses. Data teams are so hyper-focused on where the data lands in Snowflake that often they don’t realize that the data in motion, while traversing data pipelines, is visible in plain text. Failing to protect and secure data in motion before landing it in the cloud data warehouse represents a significant compliance risk in many highly regulated environments like Healthcare and Financial Services institutions.
“Shifting Left™” means initiating robust data governance and data security capabilities available in Snowflake and extending them back to data as it leaves source systems. Doing so ensures the policies are attached to, and remain with, the workload throughout the data journey to the cloud.
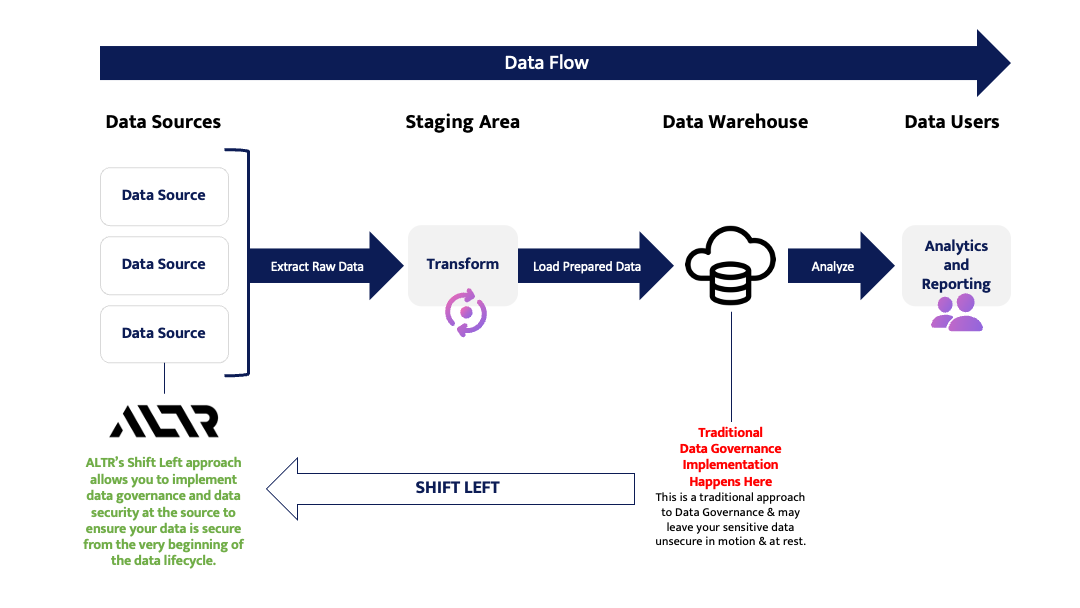
As soon as data leaves a source system and enters an ETL/ELT pipeline, that solution can call directly to ALTR through existing open-source connectors or via our Rest APIs to instrument data classification, data tagging, and data tokenization, directly in the ETL/ELT solution.
The same holds true for Data Catalogs. That means sensitive data is governed and protected from the instant it begins its journey from source to cloud. And, when those data land in Snowflake, they land with everything tagged, with active data access governance policies in place, and any highly sensitive values tokenized. Only ALTR can accomplish this because of the architectural advantages made possible through our unique integration withSnowflake. We have a growing library of open-source connectors for best-in-class solutions for ETL/ELT providers and Data Catalogs, and some providers are even building ALTR directly into their offerings (more on these exciting developments soon…).
Why is Shifting Left Critical for Your Data Governance Solution?
For many organizations, significant levels of compliance, governance, security, and privacy risks have yet to be rationalized for data in transit to the cloud. These gaps between Source Systems and Cloud represent major security threats and significant compliance issues for organizations operating in highly regulated environments like Healthcare and Financial Services.
ALTR can deliver immediate time to value, closing these compliance and security gaps from source, to Snowflake, to your data consumers. No other solution on the market today can make that same claim. ALTR’s SaaS based approach to data governance and data security is unique and is why we’re the only Data Access Governance solution that can take the same powerful capabilities over Snowflake, and shift them left to orchestrate further upstream in your data architecture.
Our esteemed competitors typically require a 6-month implementation cycle for their offerings, and they often only apply to data that already exists in Snowflake. Because of their legacy architectures and proxy-based approaches, they cannot be instrumented as highly-available, cloud-native services elsewhere in the data journey. These organizations cannot shift left™ and cannot help your organization close any compliance, security, or privacy gaps that exist before data hits Snowflake.
How Can ALTR Offer a Shift Left Approach?
ALTR is the first and only data governance solution to build a cloud-native integration with Snowflake using its external function capabilities to bring data governance and data access into the Snowflake environment. Snowflake has incredibly powerful native capabilities for data governance, yet at scale, these can be extremely complex, time-consuming, and require hours of manual SQL coding.
ALTR’s architectural advantages allow for classification, data governance, and access controls to occur seamlessly with our point and click user interface. ALTR orchestrates data governance in Snowflake because we’ve capitalized on their powerful native capabilities, making these features infinitely easier to use at scale. ALTR removes the complexity of leveraging Snowflake to its full capacity and increases the utility of Snowflake to all customers by making it safe for highly sensitive workloads and opening it up for entirely new use cases.
ALTR is uniquely positioned to offer shift left™ capabilities because we allow you to implement data governance policy into ETL pipelines, into data catalogs, into streaming busses - anywhere in your architectural diagram that exists to the left of your cloud data warehouse.
Wrapping Up
Leaving your sensitive data unsecured and out of compliance until it reaches the cloud means it’s at significant risk of exposure.The design principles of ALTR’s highly available, cloud-native, SaaS-based offering for Snowflake makes ALTR the only Data Access Governance and Security solution that can ensure the protection of your sensitive data from source system, to cloud, to data consumer.
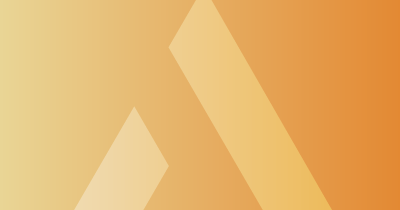
Aug 16
0
min
Snowflake Views: DIY vs ALTR
ALTR Blog
It goes without saying that in today’s environment, governing and protecting sensitive data requires using different tactics to execute an effective security strategy. Here at ALTR we offer numerous methods to choose from for your business needs; the capability to govern Snowflake data views in situations where you might want to see data that’s combined or separated is one to consider.
This blog provides a high-level explanation of what a ‘view’ is, the benefits it offers, how it works to manually govern views in Snowflake, and how to use ALTR to automate the governing of views by taking advantage of Snowflake’s native capabilities without needing to write SQL code. A couple of use case examples and a how-to demonstration video are also included that we hope you’ll find helpful.
What are Views and What are the Benefits it Offers?
A ‘view’ is a Snowflake object that allows a query result to be accessed just like it was a table. Think of it as a named query that has been saved. Snowflake users can then query this saved query as if it were a table.
Since the data within the view is the result of the query, then data engineers can create separate views that meet the needs of different types of employees, such as accountants and HR administrators at a hospital.
There are several different types of views in Snowflake that all have different behaviors such as ‘Regular Views’, ‘Materialized Views’, and ‘Secure Views’; however, for the sake of brevity, this blog will only explain views in general terms. For details on how the types of views in Snowflake differ, visit Snowflake Overview of Views.
Benefits that Views Offer
Using ALTR to govern views will enable you to only extract the data that you want to see. As a result, it will be easier to understand when you have a large amount of data.
You will also benefit by being able to grant privileges on a particular view to a specific role, without the people in that role having privileges on the table(s) underlying the view. For example, you can have one view for the HR staff and one view for the accounting staff, so that each of those roles in the hospital can only see the information needed to perform their jobs.
How Snowflake Views Work if You DIY
As stated earlier, there are different types of views that Snowflake supports. Each of them will require you to write SQL code to do it and will require you to define each ‘view’ based on the type you prefer to implement. This can be time-consuming to do and must be maintained as your business scales.
How ALTR’s Policy Automation Works with Snowflake Views
Our policy automation on Snowflake views supports column access and masking. It also enables you to identify and connect columns that exist in Snowflake Views and apply column access policies and masking rules to those columns all without writing SQL code.
Like tables, columns in views must be connected to ALTR before they can be included in governance policies. To govern a column in a Snowflake view, follow the steps below.
- From the Data Management page, click the Add New button.
- In the resulting form, select a Snowflake database.
- Next, click the View tab (shown in the screenshot). This will enable you to identify a specific column from the view to connect by selecting the schema and view for that column.
- Click Connect. Once a column in a Snowflake view is connected to ALTR, then it can be included in column access policies just like columns from tables.
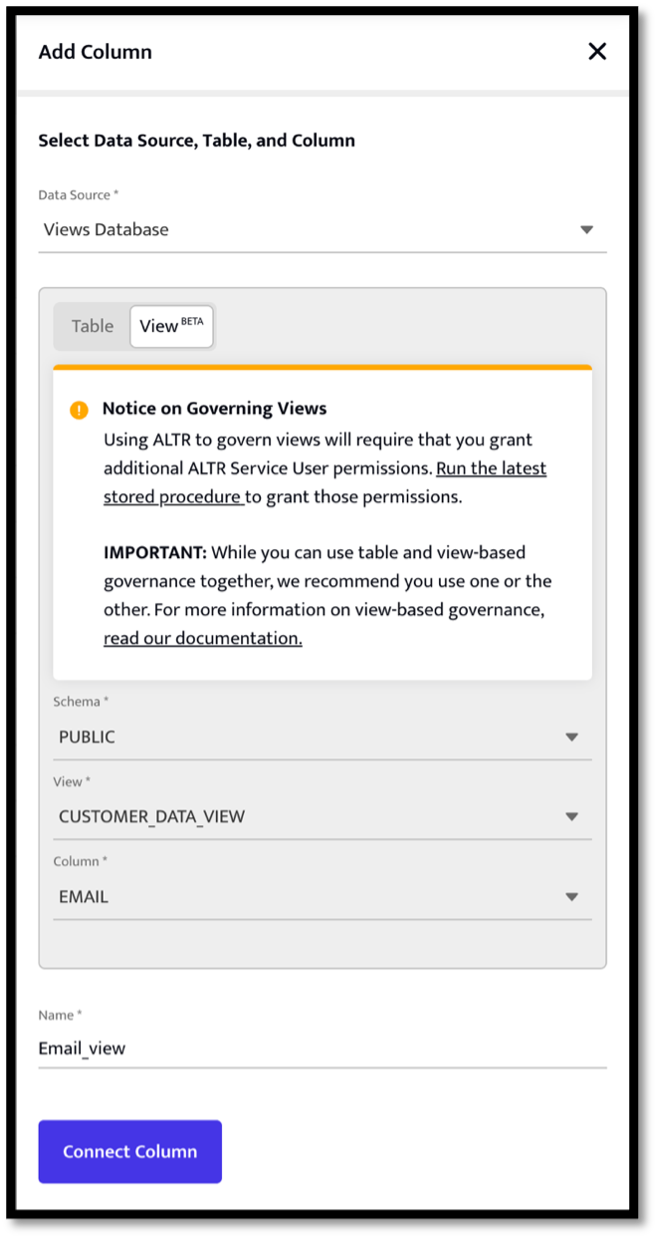
NOTE: Columns in views can also be governed through our Management API. For more details, see our Swagger documentation.
ALTR Use Cases for Snowflake Views
Good to Know: Views in Snowflake inherit the governance policies of their base tables; so, if you query data in a view, then Snowflake will still apply any Dynamic Data Masking Policies and/or Row Access Policies assigned to the Views base table(s). Because of this, it's usually much simpler to only apply governance rules once to the data in tables and leverage this functionality to prevent an explosion of masking policies. However, there are some cases where you may want to apply and manage policies at the View level. As seen in the previous section, ALTR makes adding and/or updating data access policies on views very simple.
Here are a couple of use case examples where using ALTR to govern sensitive data from Snowflake Views can benefit your business as it scales up with Snowflake usage.
Use Case 1. Your organization has a database that’s shared across different Snowflake accounts that you don’t want others to query directly. In addition, Snowflake limits the application of masking policies on the share.
To govern data within a share, you can create a separate database with views that select from the shared database. You can then govern access to columns in these views from the ALTR UI without writing SQL code. This means that you can delegate this administrative task to members of your infosec team instead of DBAs.
Use Case 2. Your Snowflake configuration primarily relies on users, BI tools, etc., querying Views instead of Tables.
Similar to the use case above, if your organization only presents views to end users and never exposes the databases directly, then you can control access to columns in these views from the ALTR UI.
Automate Snowflake Views with ALTR
By using ALTR to govern Snowflake Views, you can minimize data breaches and make informed decisions to execute an effective data security strategy. We’ve made it so simple to use that it’s just a point-and-click in ALTR and you’re done!
See it in Action
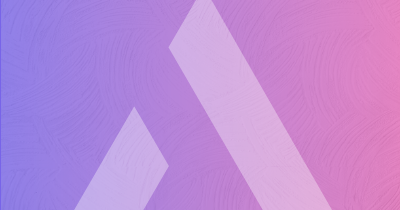
Nov 9
0
min
Automated Data Access Governance at Scale with ALTR
ALTR Blog
Data Access Governance is critical to any organization's data strategy. It ensures that the right people have access to the right data at the right time, identifies where sensitive data is being stored, and protects that sensitive information from unauthorized access. With effective Data Access Governance, organizations can strategically improve their compliance with regulatory requirements, reduce the risk of data breaches, and ensure that their data is being used for its intended purpose. It involves understanding who has access to what data, why they have access, and how that access is being managed and monitored. By implementing robust Data Access Governance, businesses and organizations can achieve greater control over their data and minimize the risks associated with data misuse and abuse.
What is Data Access Governance?
Data Access Governance is the process of managing and controlling access to data within an organization’s greater data protection strategy. It encompasses defining policies and procedures that govern who can access certain data, when they can access it, and how they can use it. The goal of Data Access Governance is to ensure that sensitive data is protected from unauthorized access, while also ensuring that the correct people have access to the information they need to do their jobs effectively.
ALTR sits at the intersection of Data Access Governance and Data Security, allowing DBAs, Data Engineers, Data Architects, or any day-to-day businesspeople to govern data access easily and without code. Many companies claim to provide solutions for data security but leave you with gaps in your data security pipeline, opening your organization up to breach. ALTR’s Data Access Governance solution puts the keys in your hands to understand what data you have, create policy around who can access what data and at what frequency, and stay on top of regulations and compliance with near real-time query audits.
Key Principles of Data Access Governance
While there are many ways to administer an effective data access governance program, strong data access governance generally revolves around the following five fundamental principles:
Transparency
As an organization, it's essential to be transparent about what data you're collecting and why you're collecting it. Clarifying what data assets you have and spreading this knowledge across your organization and customers is of utmost importance for your data governance framework. Transparency ensures that all internal and external stakeholders understand the purpose and scope of data collection efforts, fostering trust and compliance within your data governance practices.
Integrity
Data integrity is paramount in data access governance. It ensures that data remains accurate, consistent, and trustworthy throughout its lifecycle. In governance, integrity involves safeguarding data against unauthorized alterations or tampering. Robust access controls, encryption, and regular data quality checks are essential to maintain data integrity. Data users can trust that the information they access has not been compromised or altered inappropriately.
Accountability
Accountability is critical in data access governance, as it assigns responsibility for data-related actions and decisions. Every user, whether an individual or a system, should be accountable for their actions regarding data access. This includes tracking who accessed data, what changes were made, and when these actions occurred. Establishing clear roles and responsibilities ensures that individuals are answerable for their data-related activities, reducing the risk of unauthorized access or misuse.
Consistency
Consistency in data access governance ensures that access policies and practices are uniformly applied across the organization. Access controls, permissions, and policies are consistently enforced regardless of the data source, department, or user. Consistency reduces confusion and the potential for security gaps. Standardized practices simplify management, auditing, and compliance, leading to more effective data governance.
Collaboration
Collaboration is essential for effective data access governance. It encourages cross-functional teamwork among departments, including IT, data stewards, compliance teams, and business units. Collaboration ensures data access policies and decisions align with business objectives and regulatory requirements. It also helps identify and mitigate potential data access risks through collective expertise and knowledge sharing. In a collaborative environment, stakeholders work together to balance data security, compliance, and the organization's need for data access to drive innovation and productivity.
What are Steps of Data Access Governance?
Data Access Governance involves establishing policies and procedures that govern who has access to what data and under what circumstances. The principles of data access governance include:
- Defining the scope of access- Defining the scope of access involves internal standardization of access levels surrounding the data that your organization holds. A successful Data Access Governance strategy must start with seeing the scope of access and ensuring it is clearly defined to all parties. Data classification can help simplify this process tremendously by allowing data owners the visibility to see exactly what data exists that needs to be protected. ALTR lets you classify data for free on Snowflake! Learn how here.
- Establishing roles and responsibilities- Once you understand what data you have and determine which of it is sensitive, you must establish the roles and responsibilities around who is in charge of maintaining that data’s health. Clear and well-defined responsibilities ensure data is never left unmonitored, and greatly reduces the risk of breach.
- Implementing appropriate access controls- After defining what data is sensitive and establishing roles and responsibilities, the next step is implementing the appropriate access controls. This involves creating and defining policy around who is allowed access to what data and at what frequency. ALTR’s point-and-click UI allows data users the full flexibility to set correct access controls simply and scale quickly.
- Continuously monitoring and auditing access- It may feel tempting, once the work has been done to establish rules and create policy, to think that your sensitive data will run by itself. In a study done by Stanford Professor, Jeff Hancock, it was determined that, “85 percent of data breaches are caused by human error,” meaning that your data needs to be continuously monitored to protect against the human errors that may lead to breach. ALTR automates this process – further reducing the risk of human error, by providing real-time alerting capabilities and access to audit logs.
What are the Key Benefits of Data Access Governance?
Both obvious and not, there are numerous benefits to implementing a strong data access governance policy in your organization.
- It helps to ensure that sensitive data is protected from unauthorized access, reducing the risk of data breaches and other security incidents. “In 2022, the number of data compromises in the United States stood at 1802 cases,” Statista reports, this number is up 63% since 2020. Security breaches will only continue to rise as hackers become savvier, and human error remains. Implementing strong data governance with a tool like ALTR that has a proven track record of securing data is critical.
- Data Access Governance can also help to ensure that employees have access to the data they need to do their jobs, while preventing them from accessing data that is not relevant to their roles. This can help to improve productivity and collaboration while minimizing the risk of data misuse or exposure.
- Data Access Governance can help organizations comply with relevant regulations and industry standards, reducing the risk of penalties and legal action. Whether your organization must be PCI compliant, or you fall under an industry data regulation, choosing a data access governance tool that will secure your sensitive data, give your data users transparency and scalability, and offer real-time alerting is a critical priority.
What are the Challenges of Data Access Governance?
While ALTR’s automated, real-time features take the stress out of implementing, scaling, and monitoring a data access governance strategy, some organizations may face challenges when it comes to defining roles for policy management.
- Ensuring all stakeholders are on the same page: Before any policy can be created, data can be governed, or access can be monitored, all stakeholders must be on the same page.
- Determining access levels: Determining which roles or departments should have access to what data, and how much access they should have involves initial legwork of enforcing a hierarchy of status when it comes to data access. Prior to setting the parameters of role-based access or tag-based access, there needs to be clearly defined guidelines and agreement on access levels.
- Setting clear expectations: After the initial leg work is done to ensure a successful data access governance implementation, it’s critical to continue ongoing conversation to minimize the risk of responsibilities slipping through the cracks. We recommend pre-determining who will lead the charge in maintaining good data hygiene.
Once all parties are on the same page prior to initial implementation, ALTR makes creating, enforcing, and monitoring policy simple and effective.
What Industries are Deploying Data Access Governance?
Data Access Governance is a crucial aspect of data safeguarding across all organizations and all industries. Industries such as finance, healthcare, and retail are just a few examples of those who should be implementing Data Access Governance.
- Financial Services – By controlling who has access to what data, financial institutions can prevent data breaches and unauthorized use of customer information. Additionally, implementing data access governance can help financial services organizations meet regulatory requirements such as PCI-DSS and GDPR, while emphasizing protecting their members data. ALTR allows FinServ organizations the ability to quickly classify data, set policy around data, and see real-time audits of their protected information.
“Helping people navigate their financial journeys is the mission of TDECU, a Texas-based credit union with more than 366,000 members and $4.7 billion in assets. TDECU relies on large amounts of data to understand its members, ensure excellence across banking and operations, and improve the member experience.
Leveraging ALTR for automated policy enforcement, in tandem with Snowflake’s integrated security features, aligned with TDECU’s need for transparency, compliance, and control. Tokenization-as-a-service, data masking, thresholding, and integration with enterprise data governance solutions, including Collibra, were a few reasons why TDECU chose ALTR.”
Read more about why financial service organizations are choosing ALTR over others: https://www.altr.com/resource/tdecu-takes-data-driven-approach-supporting-members-financial-journeys
- Healthcare – It is crucial for healthcare companies to take essential measures like data access governance to ensure the privacy and security of their patients' personally identifiable information (PII) data. ALTR enables healthcare institutions to control data access and prevent data breaches and unauthorized use of patient information in real-time. By utilizing Data Access Governance, healthcare companies can easily meet regulatory requirements such as HIPAA and GDPR and ensure their patients information remains secure.
- Retail – Retail corporations are in charge of storing and securing the sensitive information of their customers- from shipping addresses to email addresses and occasionally credit card numbers. In order for retailers to ensure their customer’s PII is secure, they must implement a complete Data Access Governance solution. ALTR’s ability to set masking policies easily and with no-code allows retail corporations the ability to maintain a high level of security and quickly scale policy as needed.
“One of ALTR’s Enterprise customers, a multinational privately owned fast-fashion retail corporation with a direct-to-consumer presence, recognized the need to correctly store and protect the sensitive data entrusted to them. This corporation is responsible for over 60 million customer email addresses, mailing addresses and names.
After discussing the customer’s business goals, ALTR rolled out a two-step plan to accomplish the retailer’s data governance needs, starting with a custom masking policy on customer PII and following that with access controls.”
Read more about how ALTR helps retail organizations secure their sensitive data: https://www.altr.com/resource/case-study-multinational-retailer-secure-customer-pii.
What are the components of a successful Data Access Governance Strategy?
Understanding What Data You Have
Classifying your data is one of the most critical parts of beginning to protect sensitive data. The process of classifying your data allows you to begin to understand what data you have access to and identify what of that data is highly sensitive. Understanding what data is sitting in your database and identifying the columns that exist with sensitive data, puts you in a healthy position to begin setting policy and creating access controls.
Creating Policy Around Who Can Access What Data, at What Frequency
- Locks: Once you have a grasp on what data exists in your database, you can begin setting policy to ensure your data is secure and is protected from breach. ALTR’s Locks allow you to configure roles that are allowed access to data and how they are permitted to consume that data. These locks function on a least privileged access model, ensuring that even if a manual error is made, your data still remains secure. When data is queried for if your database, depending on the lock set and the person running the query, the data can return in no mask, partial mask, or full mask, dependent on the access control set.
- Thresholds: Just because a certain user group should be able to access data, doesn’t always mean that they should have unlimited access to that data. ALTR’s patented rate-limiting capabilities is key to a successful Data Access Governance strategy. Threshold alerting allows you to create policy around how many data values are being queried for and at what frequency or time of day. Thresholds allow the data owner to take the sensitive data combined with the lock and prescribe how that data can be consumed. ALTR’s real-time alerting capabilities can log that a threshold is happening or block the query altogether – giving you real-time access to know what is happening with your data at scale.
Data Usage Heatmaps & Query Audits
Protecting sensitive data – query audits & data usage heatmaps
Once your key protection measures are put into place, continuous monitoring and managing the way data is being used is critical for your Data Access Governance plan. A quick and accurate way to view data access and data usage, will ensure your organization is ahead of the curve on the front of securing sensitive data.
ALTR’s Data Usage Heatmaps show a simple view of the relationship between the roles that access data, and how much of the data is being consumed. The heatmap (shown below) offers drill down capabilities, giving you the flexibility to see activity that makes up the aggregation of data usage. By understanding who is accessing what data and at what frequency, you can baseline normal data usage for your organization and create policy around that.
Data has become the most valuable asset for businesses and organizations. Because of this, it is essential to have proper data security measures in place to protect sensitive information from unauthorized access and misuse. Whether for PCI compliance, GDRP regulations, or the many other reasons people choose to begin securing their data, Data Access Governance is crucial to your organization’s strategy to protect sensitive data.
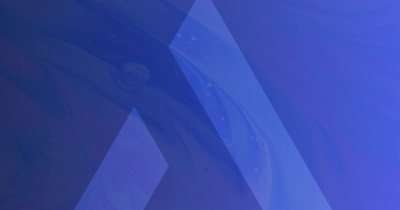
Aug 2
0
min
3 Dimensions to Determining the Right Role-Based Access Controls
ALTR Blog
While there are lots of rules around how data should be protected at rest to prevent theft or breaches, the real threat to data comes from letting people use it. In fact, the easiest way to make data safe is to put it in a vault and throw away the key. But that defeats the purpose of storing data in the first place: using it to gain insight. Your highest risk and highest reward come at the intersection of users and data. That’s why role-based access controls are so critical to both data usage and data security.
What is Role-Based Access Control?
Role-based access controls restrict access to sensitive data with policies associated with various roles. These roles could be job function, job level, department, region or more. Rather than setting access by individual user or tool, these roles are set up and assigned specific permissions based on what someone in that role needs to access to do their job. A marketing person may have one set of permissions while a finance team member may have another, or Admins might have higher level access than line of business users. When new users come on board or people change jobs, the only change required is what roles they’re assigned to. This makes data access easier to manage and less error prone.
3 Strategies for RBAC
While an RBAC approach makes a lot of sense, one of the biggest challenges we see our customers facing is determining the framework they should use. Should their roles be set by department? Should it be by job level? Based on what we’ve seen work at our customers, we recommend starting with understanding how volatile your company’s business environment is: specifically, how much and how often the data, the rules around it, and which users need it will change over time.
With this strategy in mind, here are three frameworks we’ve seen successfully help companies manage the data and user intersection via role-based access controls.
RBAC Case One: Your Data, Rules and Users Rarely Change – Set It and Forget It
For smaller companies in a more static industry, all three of the variables might not be very variable. For example, a regional bank might be looking at the same kinds of data consistently over time: who logged into the banking portal, how many payments went out, and how many ATM withdrawals there were, from which ATMs? Because they’re not rolling out new product lines or other drivers of new data very often, the types of data they analyze to run their business don't change often.
And because it’s the financial services industry, the banking rules around data security and governance are rigidly structured, specific, and slow to change. It's rare that a new regulation around the care of personal financial data rolls out in the US. Finally, in some part because of the size of the company and focused use of the data, the data users don’t change – it’s the same 5 to 10 data analysts running the same numbers daily or weekly.
In this scenario, a company can have a pretty straightforward RBAC configuration that doesn't require advanced data classification or tagging. The company can focus on well-defined “role-to-data” relationships.
For example, all PII data could be controlled, but the way that it is masked and the amount shared is determined by the role of the person accessing it. Minimal and straightforward policies could be set for how each specific role can access data:
- Marketing role has access to all data but it’s masked
- Data scientists have access to unmasked data but only 2,000 records per day
- Administrative users could have access to unmasked data as well, but only 20 records per day
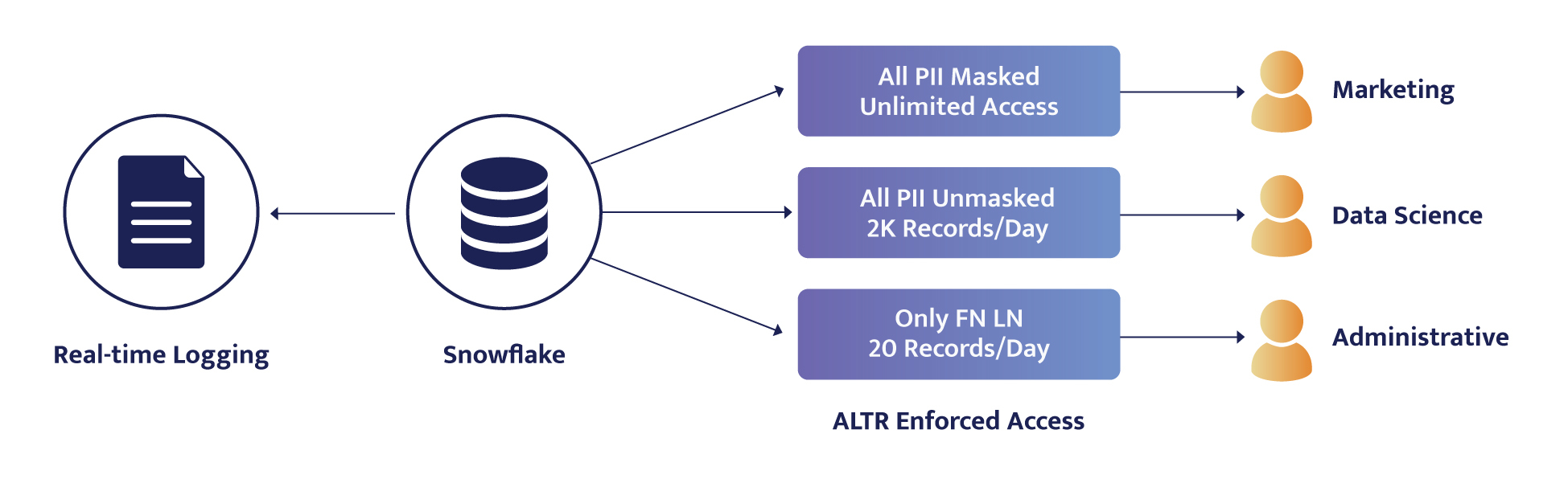
Active Directory can be integrated with Snowflake to share the role data.
RBAC Case Two: Your Data and Users Change Often but the Rules Don’t – This is Manageable
In a more dynamic industry or in a company more mature in its data lifecycle journey, there can be more variation in data and in the users needing the data, while the rules themselves don't change much. For example, a company may be bringing in different types of data from across the company, like payroll or shipping costs. Or they might be moving into new lines of business that require different kinds of data like the most popular product color or busiest intersections. They may have a decentralized data process such that various product teams can classify, tag, and add data to the data warehouse, then request access.
In this scenario, a company can make the rules specific to the type of data and the type of access that should occur. For example, they could set up data access policies and then assign the policies appropriate to the roles:
- PII SSN – No Access
- PII SSN – Last Four
- PII SSN – Full Access
- PII Phone – No Access
- PII Phone – Last Four
- PII Phone – Full Access
A specific role could then be granted one or more of these policies. Sales may get PII Phone – Full Access + PII SSN No Access.
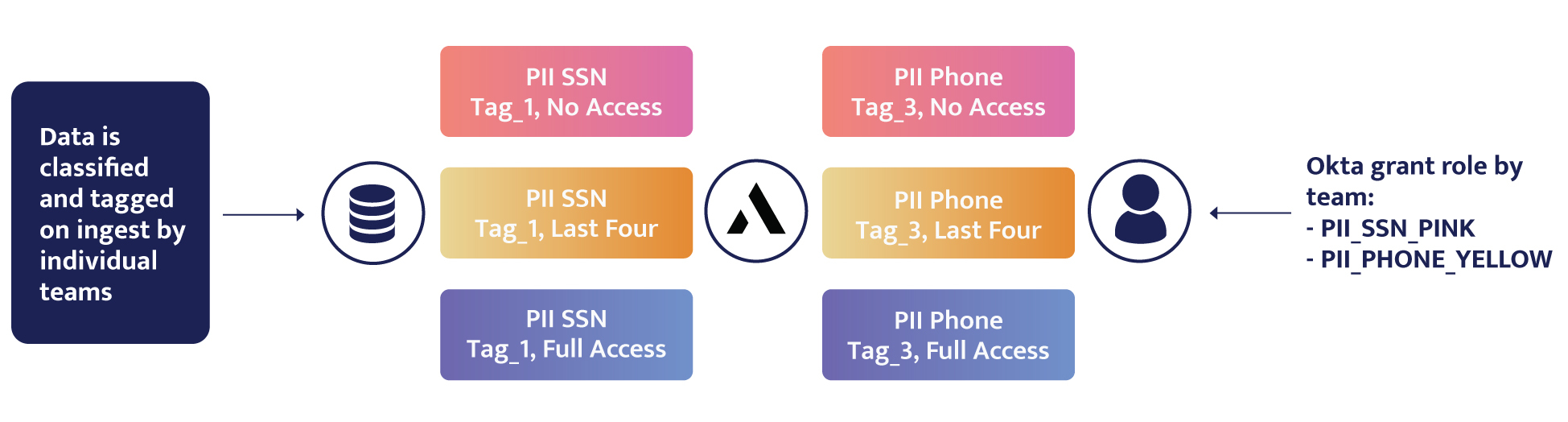
As data is loaded into Snowflake, it is classified in real-time and brought in with that classification such that it fits into one of these roles via how it's tagged. Companies can then use Okta or Active Directory to assign these policies to roles.
This means that as the data changes, it's classified in a variable way, and as the users change, whether they're new users or existing users gaining or shedding roles and responsibilities, they're added to new roles and policies in a variable way. The policies, however, are set just once because the rules around which kinds of data are sensitive and how it should be controlled don't change.
This is the most scalable approach to access control.
RBAC Case Three: Everything is Changing – Try to Keep Up
Unfortunately, not every company can fit into the previous scenarios. The third situation is the most challenging: The data changes constantly, the rules change constantly, and the users change constantly. We see this more often than you might think in specific types of companies: very large enterprises acquiring new companies in new markets or moving into new locations with new regulatory environments that are all very data-driven and data-focused throughout the entire business. These enterprises must deal with a trifecta of variability: new types of data coming in, new rules based on the industry and location, and new users across the company wanting and needing access. Because they're out in front at the leading edge, they're all still just figuring out how to manage all these moving parts.
In this case, a user may need to switch out their role multiple times throughout the day and hence access depending on what team they're working with and the hat they're wearing. A data engineer, for example, might be helping the sales team with something, and then the next fire to put out is with the data science team. Their functional role might be data quality engineer, and within that function, the user may be an admin for some data sets but just a data consumer for others; for example, the user could be an account admin for marketing because they’re GDPR-certified but a read-only user for finance because they don’t have a Series 7 and can’t see customer income statements.
Because it's challenging to set up static rules in this scenario, a hierarchy structure allows the RBAC to scale by placing policy over both functional roles and technical roles. Instead of making (and updating) a ridiculous number of separate roles, a data team can use that custom logic to evaluate the user when they’re running a query (what hat are they wearing when running the query?) and the classification of the data they’re trying to access. They can write about 8 or 10 lines of code that evaluates this dynamically and applies the correct access level for the role they’re playing at the time.
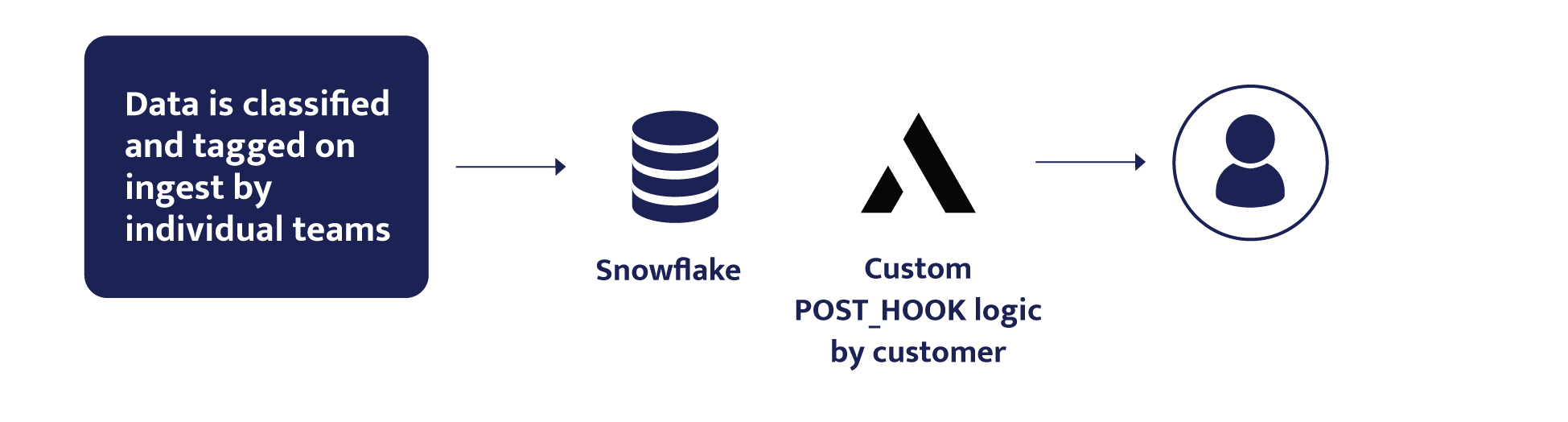
Role Hierarchy:

Conclusion:
The key to an effective role-based access control structure is understanding the fundamental forces affecting your data. Every business is different in the way that it consumes, stores, and processes data; in the way in which it follows regulations or defines internal policies; and in how it onboards, offboards, and categorizes its users. Those three dimensions can be unique to every organization but will generally fall into one of the above categories.
Starting from one of these as a foundation will help ensure your access controls are scalable and manageable for your business environment, and more than anything else, secure.

Jul 19
0
min
What is Data Masking: An Expert Guide to Safeguarding Your Sensitive Data
ALTR Blog
In the realm of modern enterprises, safeguarding sensitive data is paramount. Data breaches and regulatory compliance challenges loom large, demanding robust solutions. Fear not, for data masking emerges as the agile and versatile knight in shining armor, equipping organizations with the power to shield their precious data assets while maintaining usability and compliance.
This guide delves into the dynamic world of data masking, exploring the factors driving its adoption, the different types of masking available and critical technique selection considerations for successful implementation.
But First, What is Data Masking?
Data masking is a data protection technique involving transforming or obfuscating sensitive information within an organization's databases or systems. It aims to conceal or alter the original data to render it unreadable while maintaining its functional and logical integrity.
By replacing sensitive data with fictitious or anonymized values, data masking safeguards individuals' privacy, mitigates the risk of unauthorized access or data breaches and ensures compliance with data protection regulations. This process enables you to maintain data usability for various purposes, such as testing, development, analytics, and collaboration, while minimizing the exposure of sensitive information.
Why is Data Masking Important?
Compliance with Data Protection Regulations
Companies are often required to comply with data protection regulations such as the General Data Protection Regulation (GDPR), Health Insurance Portability and Accountability Act (HIPAA), and Payment Card Industry Data Security Standard (PCI DSS). Data masking helps you meet these regulatory requirements by protecting sensitive data and ensuring privacy.
Safeguarding Sensitive Information
Companies possess a vast amount of sensitive data, including personally identifiable information (PII), personal health information (PHI), financial records like credit card numbers (PCI), intellectual property, and trade secrets. Data masking allows you to protect this information from internal and external unauthorized access. By masking sensitive data, you can limit exposure and prevent data breaches.
Mitigating Insider Threats
Insider threats are a significant concern for companies. Employees, contractors, or partners with legitimate access to sensitive data may intentionally or accidentally misuse or disclose it. Data masking restricts the visibility of sensitive data, ensuring that only authorized individuals can view authentic information. This reduces the risk of insider threats and unauthorized data leaks.
Minimizing Data Breach Risks
Data breaches can lead to significant financial and reputational damage for companies. By masking sensitive data, the stolen or leaked data will be useless or significantly less valuable to attackers, even if a breach occurs. Masked data does not reveal original values, reducing the impact of a violation and protecting the privacy of individuals.
Creating Secure Non-Production Environments
Companies often use non-production environments for development, testing, or training purposes. These environments may contain sensitive data copies from production systems. Data masking ensures that sensitive information is replaced with realistic but fictional data, eliminating the risk of exposing real customer or employee information in non-production environments.
Enabling Data Sharing and Collaboration
Data masking allows you to securely share sensitive data with third parties, partners, or researchers. By masking the data, you can maintain privacy while still allowing data analysis, research, or collaborative efforts without compromising the confidentiality of the information.
Preserving Data Utility
Data masking techniques aim to balance data privacy and data utility. Companies must ensure that masked data remains usable for various purposes, including application development, testing, data analytics, and reporting. You can protect data using appropriate masking techniques while retaining its value and usefulness.
Types of Data that Require Data Masking
Various types of data can benefit from data masking to ensure privacy and security including:
- Personally Identifiable Information (PII): This includes names, addresses, social security numbers, passport numbers, and driver's license numbers.
- Financial Data: Credit card numbers (or PCI), bank account details, and financial transaction records are sensitive data that warrant data masking.
- Healthcare Information: Protected Health Information (PHI) like medical records, patient diagnoses, treatment details, and health insurance information must be masked to comply with regulations like HIPAA.
- Human Resources (HR) Data: Employee records, salary information, and employee identification numbers may require data masking to protect privacy and prevent identity theft.
- Customer Data: Customer names, contact information, purchase history, and loyalty program details should be masked to safeguard customer privacy.
- Intellectual Property: Trade secrets, patents, research and development data, and proprietary information should be masked to prevent unauthorized access and maintain competitiveness.
Types of Data Masking
Static Data Masking
Static data masking permanently replaces sensitive data with fictitious or anonymized values in non-production environments. It aims to provide realistic but de-identified data that can be used for testing, development, training, or sharing purposes while preserving privacy and security.
Static data masking typically operates on a copy of the original dataset, where sensitive information, such as personally identifiable information (PII) or financial details, is masked with fictional equivalents. This process ensures that the masked data retains the same structure, format, and relationships as the original data while rendering the sensitive information unreadable and meaningless to unauthorized individuals.
Dynamic Data Masking
Dynamic data masking allows real-time masking of sensitive data at the point of access based on user roles and permissions. With dynamic data masking, the sensitive data remains stored in its original form. Still, it is dynamically masked or obfuscated when queried or accessed by users who do not have the necessary privileges.
This technique provides fine-grained control over data exposure, ensuring that individuals only see the masked data they are authorized to access. Dynamic data masking helps prevent unauthorized users from viewing or accessing sensitive information while allowing authorized users to interact with the data in its unmasked form.
Deterministic Data Masking
Deterministic data masking is a data protection technique where sensitive data is consistently transformed into the same masked output value using a predefined algorithm or function. Unlike other masking methods that introduce randomness or variability, deterministic data masking ensures that the same input value will always result in the same masked value.
This approach is instrumental when data relationships, referential integrity, or consistency must be maintained across different systems or environments. However, it is essential to consider potential privacy and security risks associated with deterministic data masking, as the consistent masking pattern could potentially be exploited through reverse engineering or pattern recognition techniques, necessitating additional safeguards to protect sensitive information.
Data Masking Techniques
When we use the term “data masking” by default we’re often referring to the practice of replacing some numbers in a string with asterisks – such as an email address like ****@altr.com. However, data masking can actually refer to a wide range of techniques for obfuscating and anonymizing data. Here are a few data masking techniques.
Format-Preserving Encryption
Format Preserving Encryption (FPE) allows data to be encrypted while retaining its original format, such as length or data type. It ensures compatibility with existing systems and processes, making it useful for protecting sensitive data without extensive modifications. FPE can be deterministic or randomized, providing consistent or variable ciphertext for the same input. It is commonly used when preserving data format is crucial, such as encrypting credit card numbers or identification codes while maintaining their structure.
Data Tokenization
Data tokenization replaces sensitive data with unique tokens or surrogate values. Unlike encryption, where data is transformed into ciphertext, tokenization generates a token with no mathematical relationship to the original data. The token serves as a reference or placeholder for the sensitive information, while the actual data is securely stored in a separate location called a token vault. Tokenization ensures that sensitive data is never exposed, even within the organization's systems or databases.
Data Scrambling
Scrambling involves shuffling or rearranging the characters or values within a data field, rendering it unreadable without affecting its overall structure. This technique is commonly used for preserving data integrity while masking sensitive information.
For example, consider a dataset containing employee salary information. With data scrambling, the original values within the "Salary" field are shuffled or rearranged in random order. For instance, an employee with a salary of $50,000 might be masked as $80,000, while another employee's salary of $75,000 could become $35,000. The resulting scrambled values retain the structure of the data but make it challenging to associate specific salaries with individuals.
Data Substitution
Substitution replaces sensitive data with fictitious values, ensuring that the overall format and characteristics of the data remain intact. Examples include replacing names, addresses, or phone numbers with random or fictional counterparts.
Data Shuffling
Data Shuffling rearranges sensitive information randomly, breaking the relationship between values while preserving data structure. For example, imagine a dataset containing customer information, including names and addresses. With data shuffling, the original values within each field are scrambled, resulting in a randomized order. For instance, the name "John Smith" might become "Smith John," and the address "123 Main Street" could transform into "Street Main 123."
Value Variance
Value variance adds an element of unpredictability to the masking process. It ensures that the resulting masked value varies across instances even when the same original value is encountered. For example, a social security number "123-45-6789" might be masked as "XXX-XX-XXXX" in one instance and "555-55-5555" in another. By introducing this variability, value variance thwarts attempts to correlate masked data, making it significantly more challenging for unauthorized individuals to uncover sensitive information.
Nulling Out
Nulling out replaces sensitive information with null or empty values, removing any trace of the original data. This technique is beneficial when sensitive information is not required for specific use cases, such as non-production environments or scenarios where privacy is a top concern. Nulling out eliminates sensitive data, minimizing the risk of accidental exposure or unauthorized access.
Pseudonymization
Pseudonymization replaces sensitive data with pseudonyms or surrogate values. The pseudonyms used in the process are typically unique and unrelated to the original data, making it challenging to link the pseudonymized data back to the original individuals or sensitive information.
For example, healthcare data might contain a patient's name, "John Smith," address, "123 Main Street," and medical record, "PatientID: 56789." Through pseudonymization, the organization replaces these values with unique and unrelated pseudonyms. For instance, the patient's name could be pseudonymized as "Pseudonym1," the address as "Pseudonym2," and the medical record as "Pseudonym3." These pseudonyms are consistent for a particular individual across different records but are not directly linked to their original data.
How to Determine Which Data Masking Technique is Right for You
When determining which data masking technique to apply, several factors should be considered:
Data Sensitivity
First things first, you must understand the sensitivity of the data being masked. Identify the specific data elements that need protection, such as personally identifiable information (PII), financial data, or healthcare records. This assessment helps determine the level of masking required and guides the selection of appropriate techniques.
Regulatory and Compliance Requirements
Consider the relevant data protection regulations and compliance standards that govern the data. Different regulations may have specific requirements for data masking or anonymization. Ensure that the chosen technique aligns with the regulatory obligations applicable to the data.
Data Usage and Usability
Evaluate how the data will be used and the level of functionality required. Consider the intended application, such as testing, development, analytics, or research. The selected technique should preserve the usability and integrity of the data while protecting sensitive information.
Data Relationships and Dependencies
Assess the data relationships and dependencies within the dataset. Determine if any referential integrity constraints, foreign critical dependencies, or relational dependencies need to be maintained. The chosen technique should preserve these relationships while masking sensitive data.
Performance and Scalability
Consider the performance impact and scalability of the chosen technique. Some masking techniques may introduce additional processing overhead, impacting system performance or response times. Evaluate the system's capacity to handle the masking process effectively and efficiently, especially for large datasets or complex queries.
Security and Access Controls
Evaluate the security requirements and access controls associated with the data. Consider the level of granularity needed to control access to masked data. Some techniques, such as dynamic data masking, provide fine-grained control over data exposure based on user roles and permissions.
Data Retention and Data Lifecycle
Assess the data retention policies and the lifecycle of the data. Determine if the masked data needs to be retained for a specific period and if there are any data destruction or archival requirements. Consider how the chosen technique aligns with the data retention and lifecycle requirements.
Cost and Resources
Evaluate the cost and resource implications of implementing the chosen masking technique. Some techniques may require specialized tools or resources for implementation and maintenance. Consider the budgetary constraints and resource availability within the organization.
Wrapping Up
In a world where data is king and privacy is paramount, data masking emerges as the unsung hero in data security. It's the guardian of sensitive information, the gatekeeper against breaches, and the enabler of trust in an interconnected landscape. With a careful blend of innovation and best practices, data masking allows organizations to dance the delicate tango of privacy and usability, ensuring data remains safe while retaining its functionality.

Jul 11
0
min
ALTR’s Integration Tokenizes Data Automatically in the Matillion Data Pipeline
ALTR Blog
Today’s business environment has no time for silos or lack of collaboration. This challenge is coming to a head at the intersection of data and security. Data teams focus on terms like “quality, accuracy, and availability,” while security teams care about “confidentiality, integrity, and risk reduction.” This often means Data teams want “real-time access” at the same time that Security teams require “real-time security.” But the truth is that both actually have the same goal: extracting maximum business value from the data.
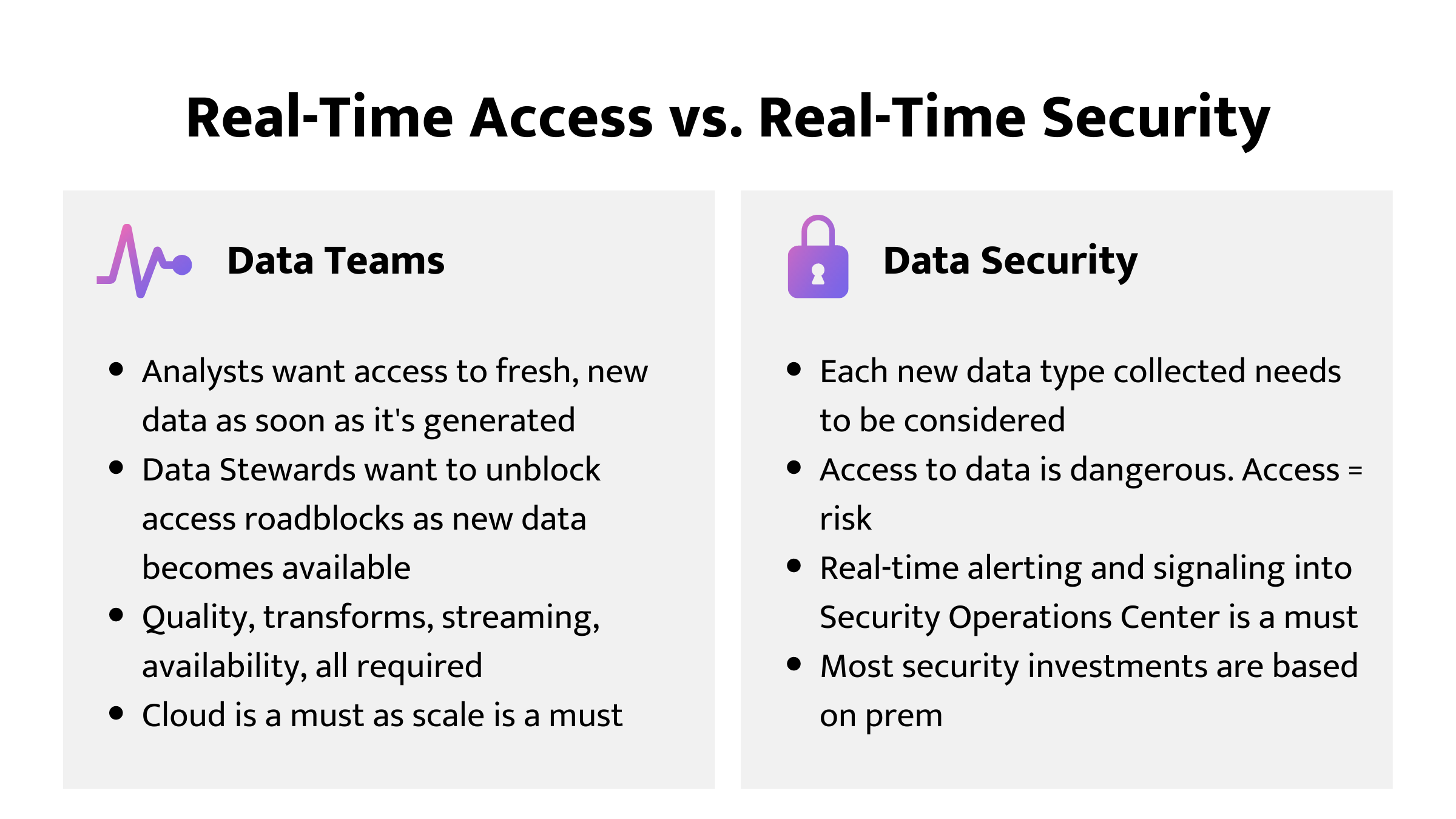
Integrated Security = Streamlined Value
After teams realize they have the same goal, the next step is to converge around shared tools and processes. In many companies, data moves at the speed of the business and increasingly this means at the speed of the cloud. In order to keep up, both data and security teams need tools that have been built for that speed and delivery. The data productivity cloud from Matillion combined with ALTR’s SaaS data access governance and data security platform can be the shared tool set needed to deliver this streamlined value.
Integrated Data Stack
Figure 2 below might look like a complicated data ecosystem, but the point is that it’s actually not. Think about your own stack - this probably looks pretty familiar. That’s because you undoubtedly have a lot going on with the data in your business. What you should notice in this diagram is that Snowflake remains at the center, and everything else works around it. ALTR integrates and works with existing tools to deliver security so that it doesn’t disrupt or interfere with your existing stack – BI tools, Data apps, custom code.
Matillion is a platform to help you get your data business ready faster. It does this by Moving data, Transforming data and Orchestrating the data Pipelines.
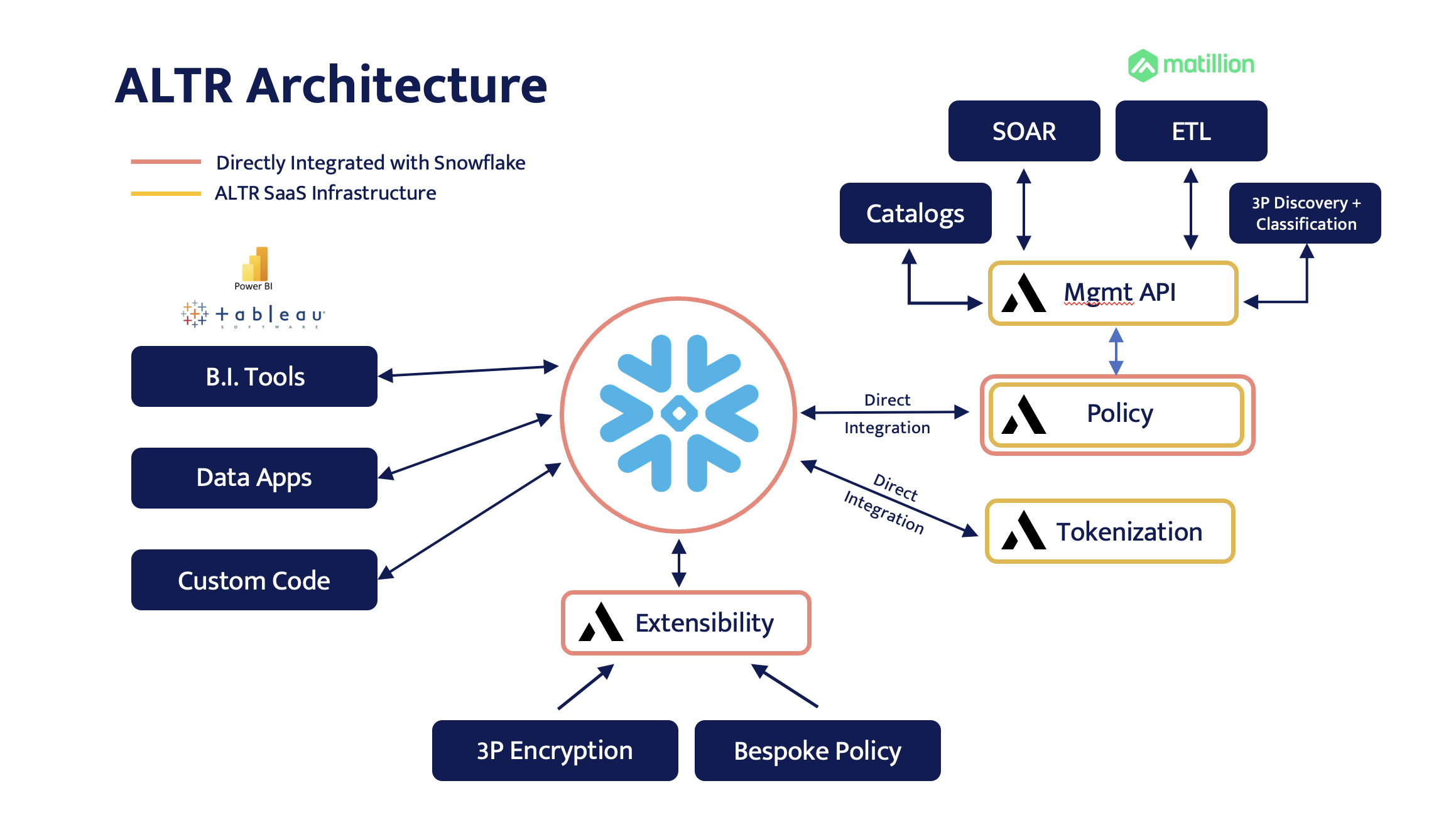
ALTR Tokenization + Access Control + Real Time Alerting
The killer integration to solve for the shared responsibility of CDO and CISO is tokenization + access control + alerting. The ability to ensure privacy, security, and governance are all addressed with a single technology is key. This can be achieved with tokenization plus access control for integrated policy enforcement.
• Data is classified and tagged from ingestion point
• Data is automatically protected at ingestion point based on tag-based policy
• RBAC and other Governance Access items configured once
• Data lands in Snowflake ready to be queried according to policy. No SnowSQL. No SDLC. It just works.
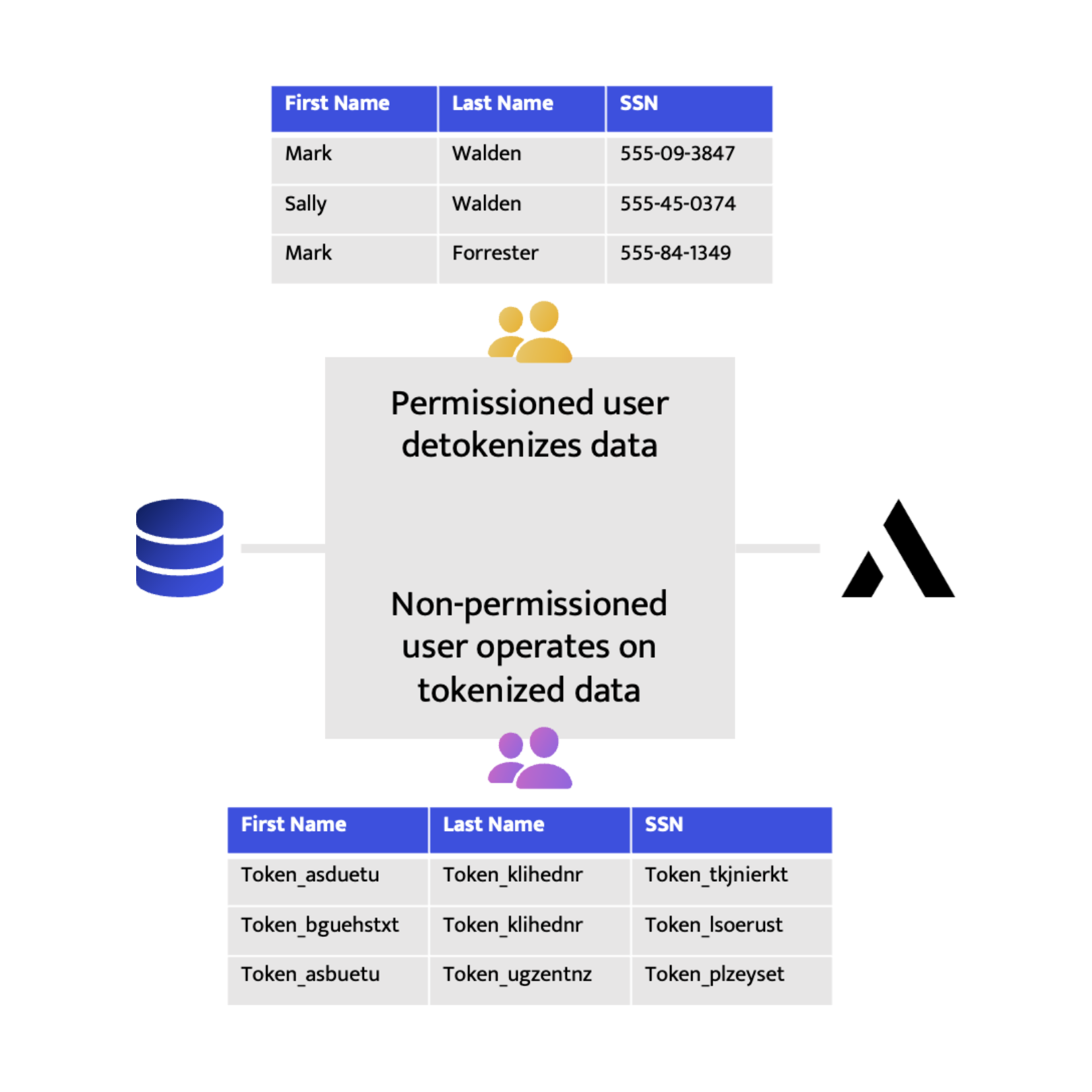
Operationalize Tokenization + Access Control + Real Time Alerting on Matillion Data Productivity Cloud
With ALTR’s new integration for the Matillion Data Productivity cloud, set up is easy – ALTR is natively integrated with Matillion. This native integration is currently a proof-of-concept but will be live on the new Matillion Data Productivity Cloud very soon!
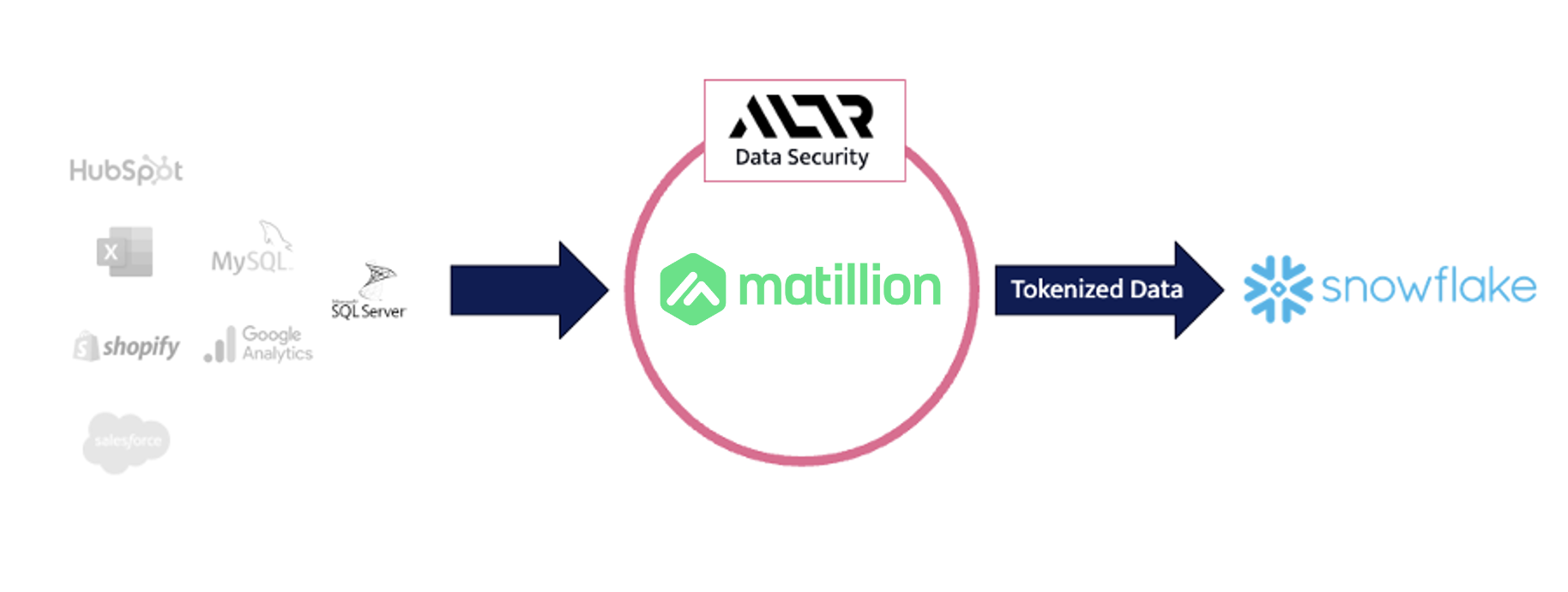
Data classification done by ALTR is set up in Matillion. Then that data is tokenized based on those classification tags automatically when it lands in Snowflake - on the fly. For example, if Social Security numbers are found during the classification process, columns are tagged with SSN, and if the policy requires that data be tokenized, it will be done automatically. This helps to satisfy data security requirements natively in your data pipeline. De-tokenization rules are based on the user, and it doesn’t matter where the user accesses the data from – from Snowflake UI or in Matillion – ALTR’s data access governance policy is applied because data is sitting in Snowflake in its tokenized form. Data teams appreciate this as they want to access the data as soon as it's available in Snowflake. With tokenization + access control, both teams are getting what they need from the already invested tool sets.
It also doesn’t matter which data source the data originates from – RDS, Workday, SAP, Salesforce. Wherever you’re pulling data from, new data is flowing into the pipeline is categorized, tagged and tokenized based on the pre-set policies around data types. That means whenever data teams want to add another data source, it will be secured.
As this data is accessed, security teams continue to receive customized access history logs which can be configured to alert when certain types of access occur. This access might be outside working hours, across many different data types, or a larger than normal request of sensitive data from a user or a role. Security teams can be certain that only appropriate access is occurring, and data teams know what guardrails they need to operate within.
This solution removes all the bottlenecks of migrating data by doing the hard security stuff required automatically. It also means data teams can stop thinking about security and focus on other data issues like quality and continued migration while knowing they’re meeting the requirements of their partners on the security team.
Full tokenization plus policy integrated directly into your ETL pipeline regardless of the source – no one else makes securing your data migration this easy.
See it in action…
Try Matillion Free...
and ALTR Free Today!
Get the latest from ALTR
Subscribe below to stay up to date with our team, upcoming events, new feature releases, and more.
Thank you! Your submission has been received!
Oops! Something went wrong while submitting the form.